The Future of Spatial Technology, Analysis, and Imaging
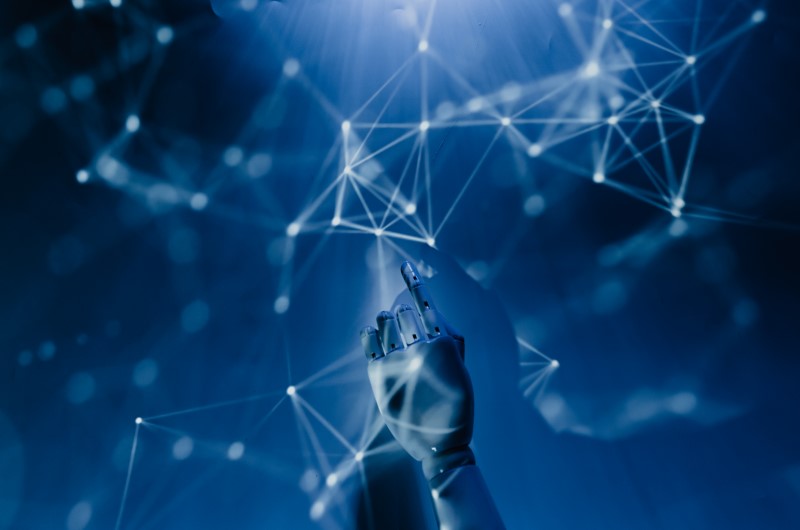
What is the future of spatial technology? How will spatial analysis and spatial imaging evolve over the next couple of years? And is what is needed to streamline the development of spatial omics?
During Oxford Global’s annual Spatial Biology US event held in 2022, a panel of experts congregated to discuss the future directions and projections for the spatial technology industry. The panel was moderated by Michael Surace, Director, TME & IO, Translational Medicine at AstraZeneca and welcomed key opinion leaders from both academia and industry.
- Spatial Analysis of TME Microglia in Melanoma Brain Metastasis
- FDA Clears Genomic Profiling Test for Solid Tumours
- Spatial Omics Technologies: Spatial Proteomics, Mass Spectrometry, and Imaging Data Uses
Panellists included Faisal Mahmood, Principal Investigator & Associate Professor, at Harvard Medical School; Arvind Rao, Associate Professor, at the University of Michigan; Jonathan Liu, Professor of Mechanical Engineering, Bioengineering, and Laboratory Medicine & Pathology, at the University of Washington; and Alex Tamburino, Director, Spatial Transcriptomics and Multiomics Sequencing Lead at Merck and Co. This insight article looks at some of the exclusive takeaways from the discussion.
Pushing Spatial Technologies into the Future
When it comes to clinical validation of spatial technologies, there are several criteria that must be met. AI-based technology models require rigorous FDA approval; they are among those innovations that undergo scrutiny of “the highest level,” Surace explained.
As AI and AI imaging become more mainstream, the regulations around clinical validation will have to match the pace and nuances of technological advancement.
For the clinical validation of spatial technologies to become more widespread, a more flexible approach to approval is needed. It can be argued that although the FDA conducts itself with extensive rigour, there is room for movement in the computer science and machine learning community. This is because the field is still working on developing better interpretability tools and methods to accurately represent data and features that remain abstract.
The data derived and inner workings of technologies such as deep learning can remain slightly hidden or aloof. Methods are being developed to essentially open this black box, but the strategy to do so must be very fluid and variable. As AI and AI imaging become more mainstream, the regulations around clinical validation will have to match the pace and nuances of technological advancement.
Industry Standardisation of Data
Another key component in unlocking the future of spatial technology, analysis, and imaging is the issue of data standardisation. “There are some concerns in the field that during clinical validation, we risk paying too much attention to, or ‘overfitting,’ a certain feature of a model that is not generalisable,” Surace explained.
In data science, overfitting is a phenomenon that occurs when a statistical model is only able to perform accurately with its training data and not against unseen data. This means the algorithm unfortunately cannot achieve its purpose and lacks relevance to the disease progression in question. With overfitting, encouraging results achieved on a model do not always generalise well in practice.
To overcome this risk, post hoc analysis is key. For instance, with AI-imaging it is beneficial to perform further analysis on the regions identified during prediction. This is because quantitative analysis based on human knowledge of the entire test set ensures greater accuracy. Independent dataset testing indicates how well the spatial technology model will generalise to out-of-domain data. The panel agreed that this method provides optimal opportunity for mitigating overfitting risk and thus enabling standardisation of data.
Collaboration Between Human and Artificial Intelligence: Predicting the Long-Term Projections
The relationship between human and computer in spatial omics is set to evolve in the coming years. Within the industry, the interface between machine learning and advanced algorithms as a human aid is a well-established and proven strategy for diagnosis. But what are the long-term projections of a human and non-human collaborative team is going to look like?
In determining the ideal operating point between human and machine collaboration, it is necessary to anticipate “how to meaningfully calibrate the uncertainty associated with predictions or suggestions from an AI agent and the combination of multiple rational agents in the decision-making process.” This contribution, voiced by Rao, attests to the intricacies and responsibilities of AI for healthcare.
Rao continued by explaining, “in this high-stakes, asymmetric risk game of computerised healthcare, there are multiple people and disciplines involved. And despite the intensive training and credibility each component brings — which is intrinsic to the decision-making process — when they come altogether, there could be a cascade of errors.”
Nevertheless, there remains an equal chance of achieving a fully synchronised and “agreeable” process management that extends all the way from input to functional and achievable output. “Therefore, we cannot ignore the amazing potential of such a collaboration when it comes together and works well,” he continued.
What is clear when contemplating the future of spatial technology is that it is not simply a case of ‘human versus computer’ but rather computerised assisted functioning that extend our rational capabilities. Moreover, when it comes to pathologists working in diagnosis, the majority are looking for an easy, understandable, and accurate means of interpreting large data sets, and computerised technology is the way to achieve this.
Visit our Omics Portal to learn more about the latest research into spatial omics and its developments. If you’d like to register your interest in Oxford Global's upcoming Spatial Biology UK: In-Person event, click here.