Advancing Precision Medicine
Providing scientists with interdisciplinary knowledge to transform human data into precise patient-centric solutions by fuelling accelerated breakthroughs in multi-omics, biomarker & imaging technologies
Events
Community
Services
Resources
PLUS Pass
In-Person Events
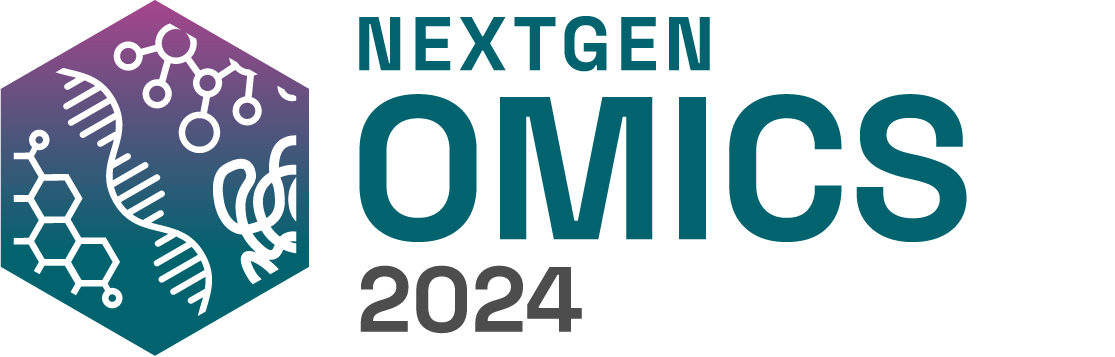
23 - 25 Oct 2024 | London, UK
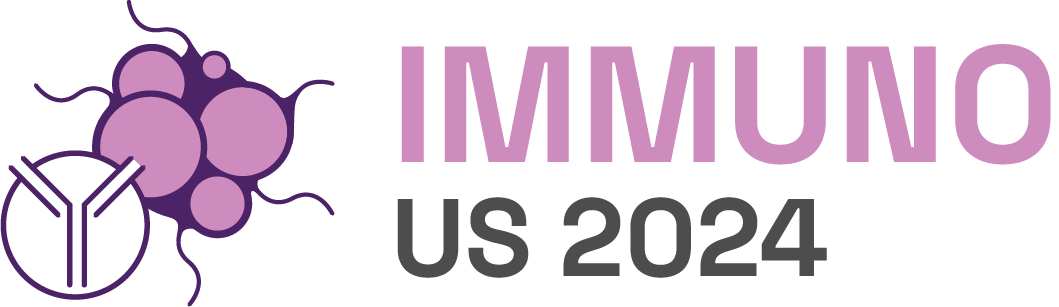
28 - 29 Oct 2024 | San Diego, CA
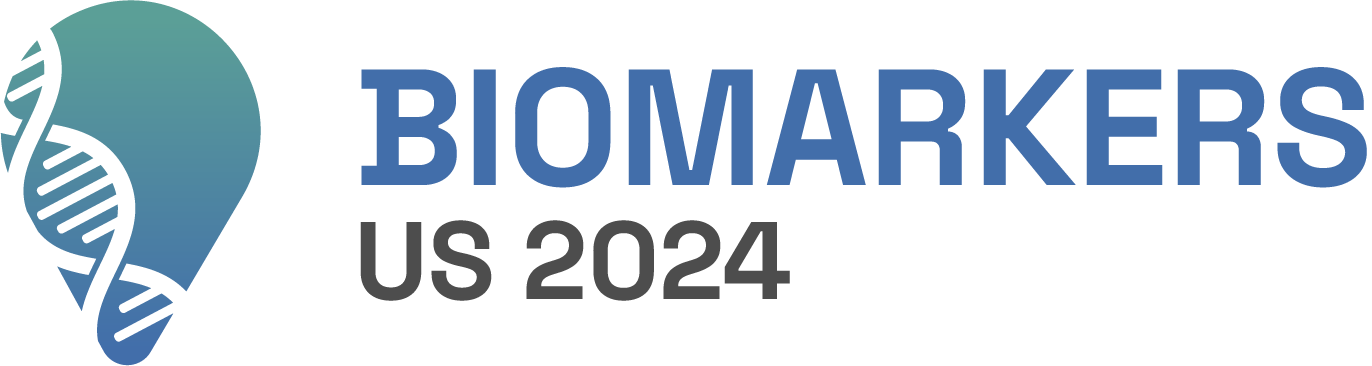
28 - 29 Oct 2024 | San Diego, CA
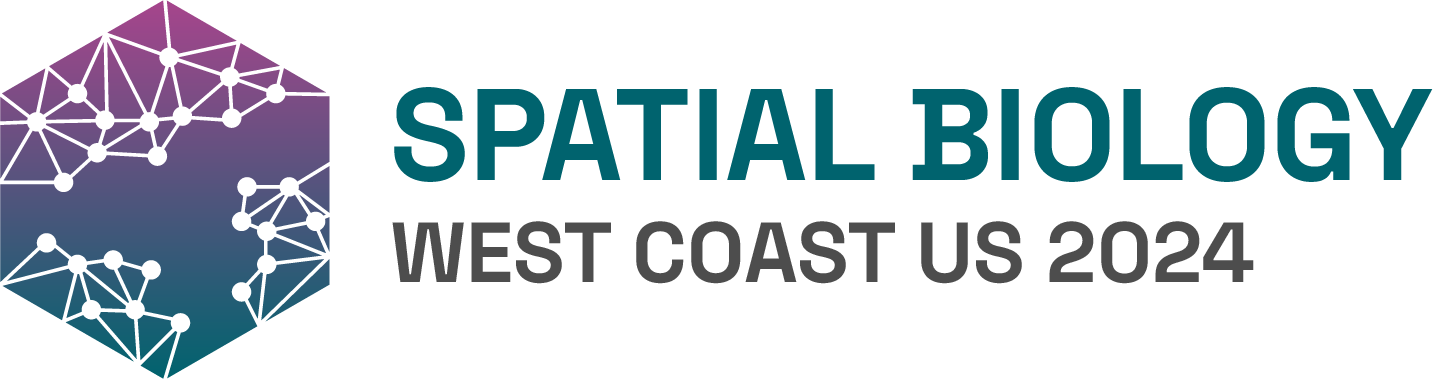
04 - 05 Dec 2024 | San Diego, CA
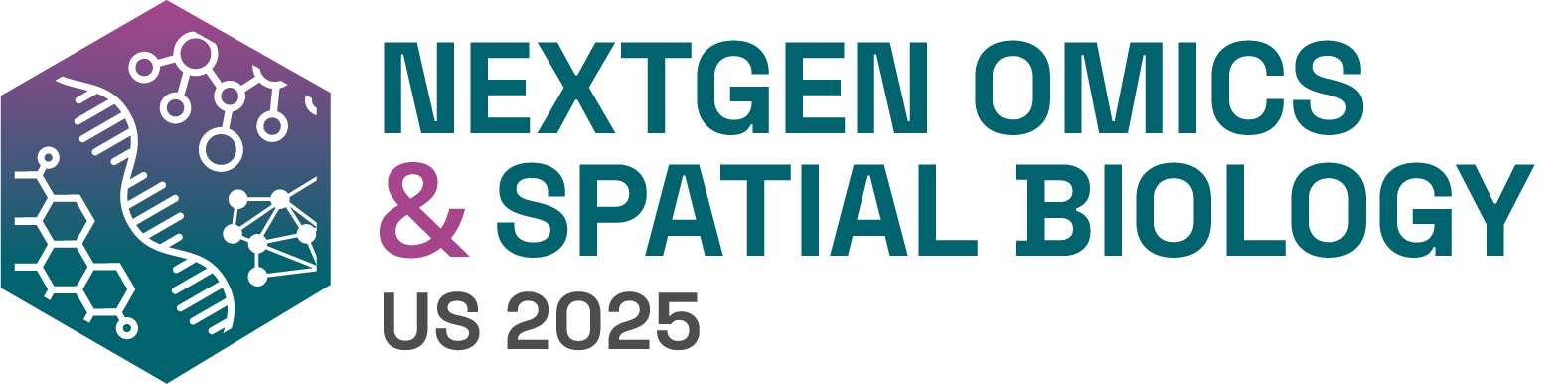
27 - 28 Mar 2025 | Boston, MA
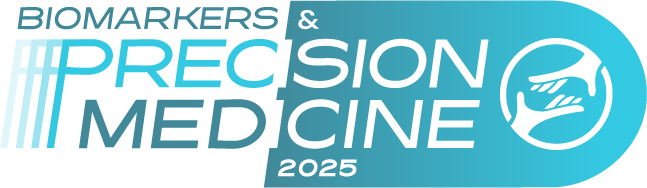
30 Sep - 01 Oct 2025 | London, UK
Online Events & Activities
Precision Medicine & Our Community
We bring together pioneering minds from the biopharma and research communities, providing a platform for exchanging insights and accelerating the development of targeted therapies that transform patient outcomes
Our In-Person & Digital Services
We work with cutting-edge vendors within precision medicine, supporting them to forge in-depth connections with customers, showcase their solutions and position themselves as market leaders.
Featured Resources
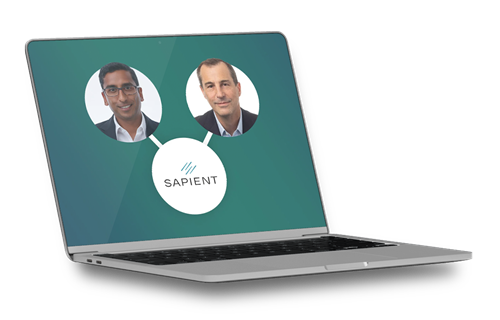
Featured Webinars
A New Golden Age for Target Identification: Accessing the Novel Druggable Space with Discovery Proteomics
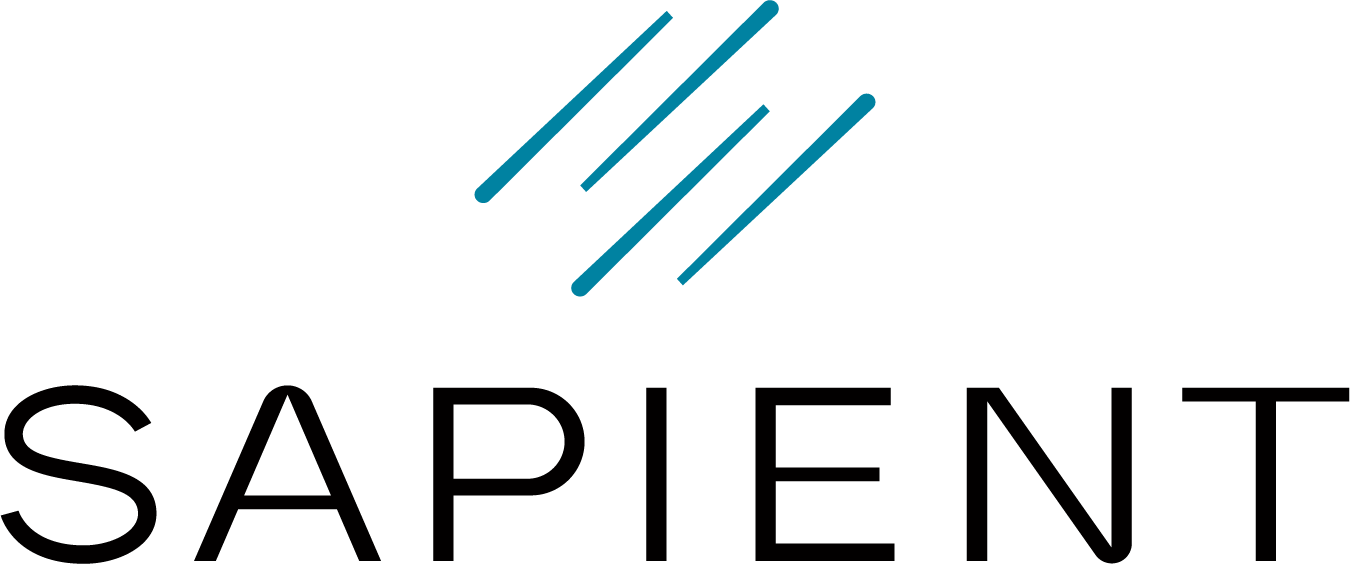
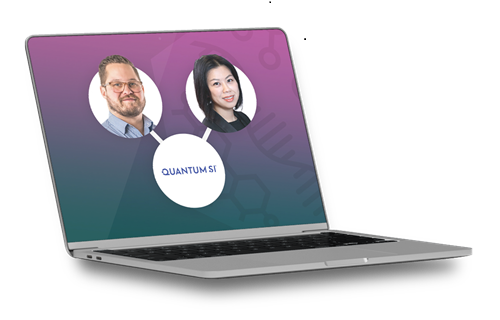
Featured Webinars
Accelerate Protein Screening by Sequencing Protein Barcodes on Platinum®

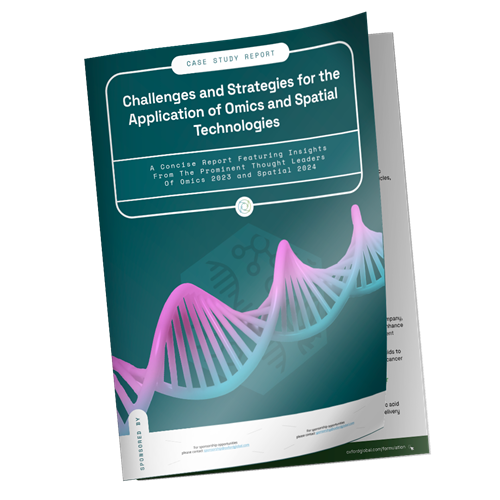
Featured eBooks & Best Practices
Challenges and Strategies for the Application of Omics and Spatial Technologies
Latest Resources
Imaging Starts Using Brand New Radiotracer from Nuclidium
Oxford Global PLUS Pass
Tailored for senior biopharma leaders & scientists, the PLUS Pass is your exclusive, all access gateway to 12 months of flagship conferences, online events and insightful content.
You’ll gain priority access to all Oxford Global events across not just the Precision Medicine brand, but our NextGen Biomed and Discovery & Development brands as well – from in-person events in the UK, Europe and USA to our regular online symposiums, webinars and group discussions, each designed to provide a productive environment for knowledge-sharing and networking. Plus, our PLUS Platform hosts a wealth of on-demand event recordings, content articles and industry news to help you keep your finger on the pulse of emerging industry trends and technology advancements year-round.
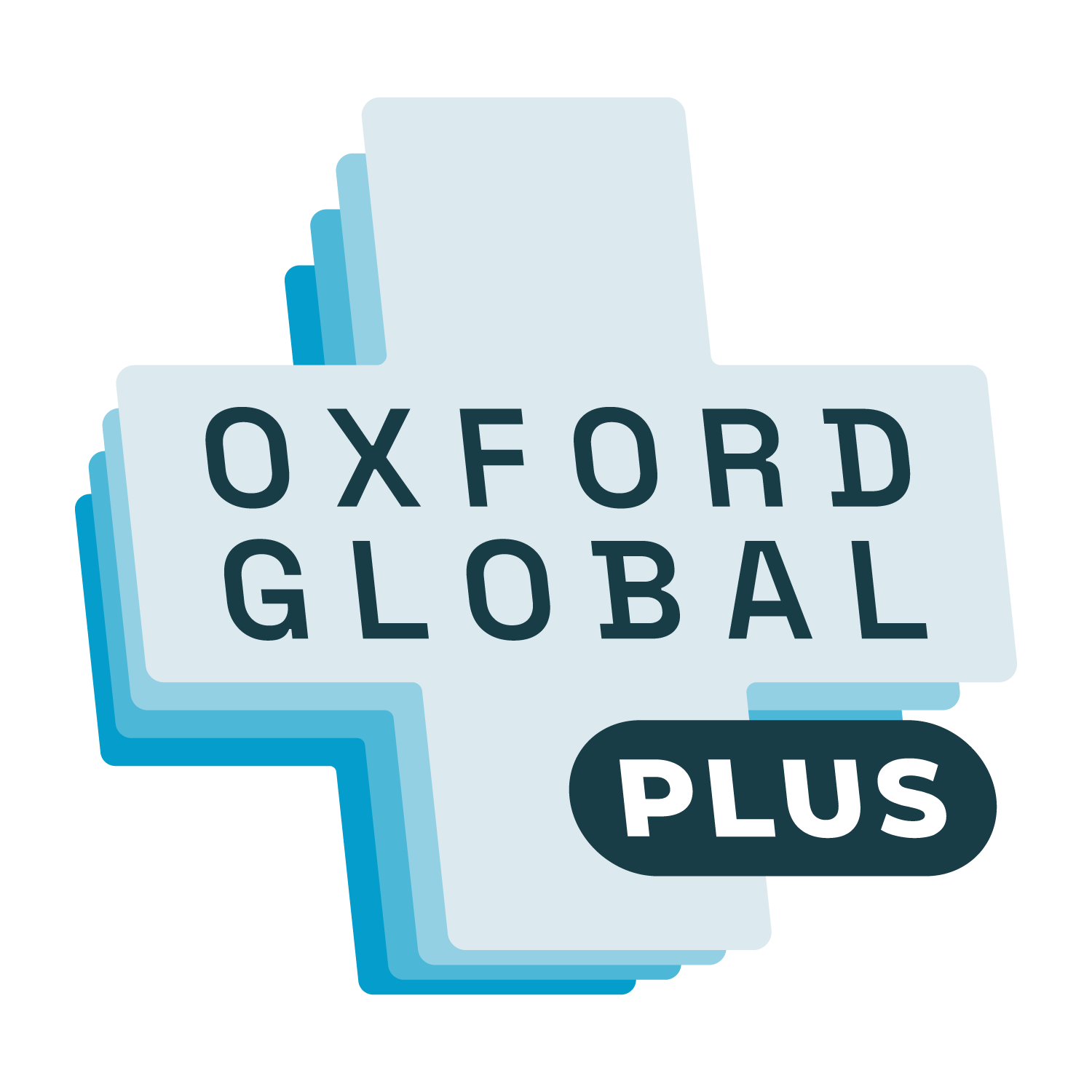