The Black Box Effect - How Can AI and ML Provide Transparent Insights for Drug Discovery?
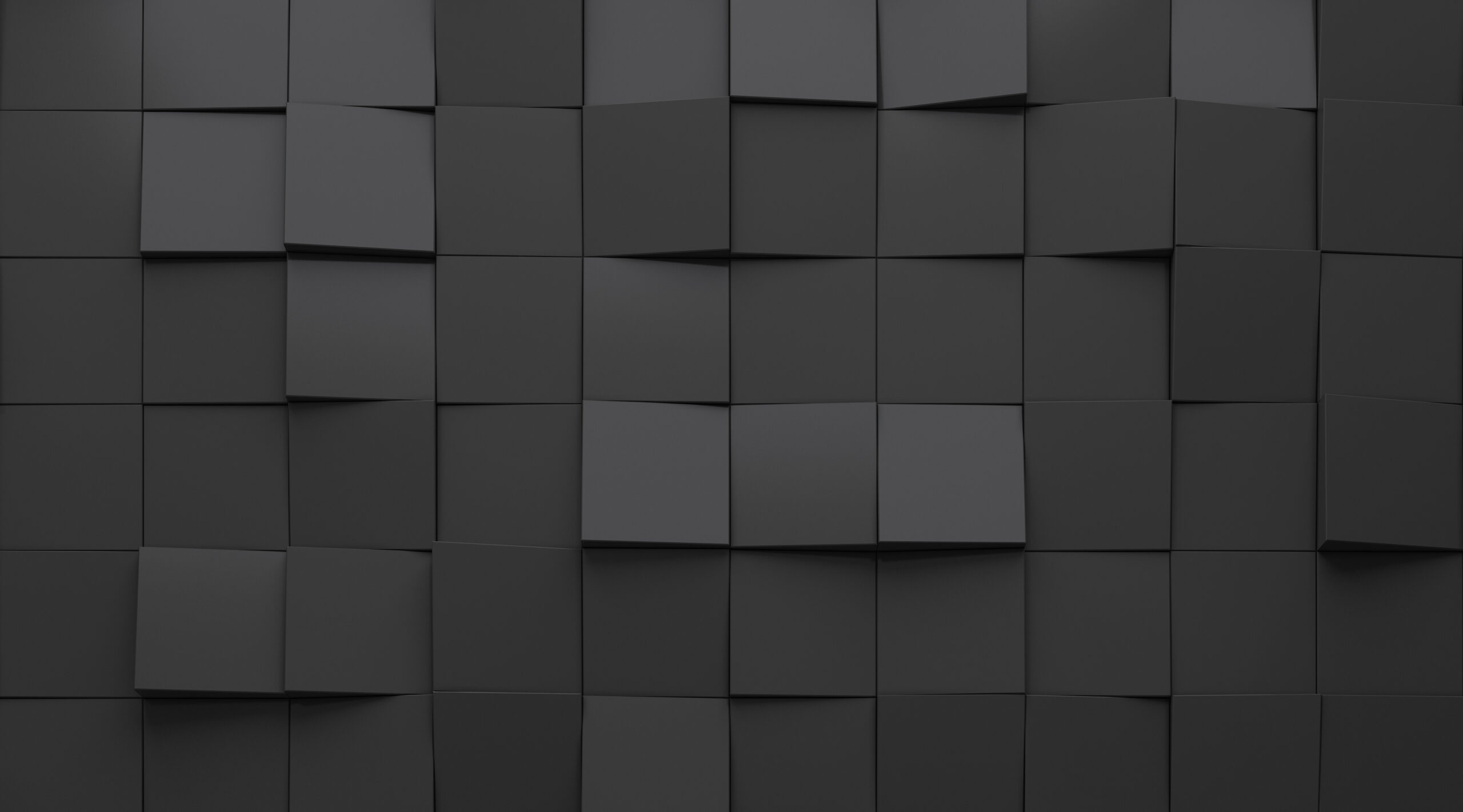
In data analysis, the ‘Black Box Effect’ refers to an artificial intelligence (AI) system, device, or program that provides useful information without revealing any information about its internal workings. The explanations for its results and conclusions remain hidden or ‘black.’
The Black Box Effect is a controversial and much-discussed topic in drug discovery, often raising questions about the feasibility of machine learning (ML). According to Martin Akerman, Chief Technology Officer and Co-Founder at Envisagenics, this issue becomes a major stumbling block for researchers during the drug discovery phase.
- What’s Next for Antibody-Based Therapeutic Discovery and Optimisation?
- Computational Engineering – the Future of Biologics Discovery?
- Nature Announces Top 10 R&D Institutes
“The Black Box Effect suggests that it's not always easy, relatable, or actionable to work with machine learning when there is an evident lack of understanding about the logic behind such predictions,” he explained. Now, however, this could all change. Envisagenics, an AI-driven RNA therapeutics development company, is working to overcome the Black Box Effect by seeking to optimise the transparency and interpretability of predictive models for drug target discovery in diseases shown to have RNA splicing deregulation.
Envisagenics – A Brief History
Envisagenics was founded in 2014 as a spin-out company from Cold Spring Harbor Laboratory and has a therapeutic area of focus on oncology, neurodegenerative, and metabolic diseases. They specialise in proprietary drug target discovery platforms driven by AI and ML and work with the following therapeutic modalities: antisense oligonucleotides and immunotherapies.
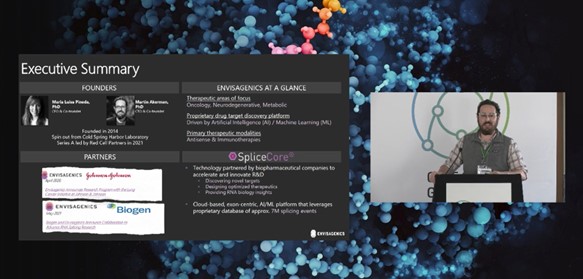
Martin Akerman, Chief Technology Officer and Co-Founder at Envisagenics, presenting at Oxford Global's Biologics: In-Person 2022.
SpliceCore® is Envisagenics’ proprietary platform, a cloud-based, exon-centric form of AI and ML aimed at accelerating and innovating research and development. The platform is capable of identifying up to 7 million unique splicing events and facilitates the discovery of novel splicing-derived targets. Other functions include designing optimised therapeutics and providing RNA biology insights that were not available before. Notable partnerships have included collaborations with Johnson & Johnson in the field of lung cancer and with Biogen in the area of central nervous system diseases.
“At its core, Envisagenics is a drug discovery company,” Akerman explained. This is because “new drug modalities call for new methods of discovering drug targets.” However, because drug development is expensive, there is a pressing need to mitigate risk. Envisagenics aims to achieve this using its target discovery technology that leverages RNA splicing quantification and interpretation. “Our promising AI/ML platform works to improve the drug target innovation rate, which has flattened in recent years,” Akerman added.
Component of a Transparent AI Model
Establishing a hypothesis-driven and predictive testing process is critical for achieving a transparent AI model. “There is a need to build more confidence around AI and reduce the Black Box Effect,” Akerman claimed. “The Envisagenics recipe that we follow to build AI takes this context into account.”
To achieve this, Envisagenics focuses on applying target discovery for specific drug modalities. Harnessing a modality-specific focus provides the necessary context for the software and helps to implement domain knowledge as a key component of the predictive engine. Domain knowledge acts as a feedback loop where prior understanding of the targets and their functionalities are incorporated into the model to improve relatability.
“The real art comes with trying to transform this biological insight into something that is intuitive and can be translated into an algorithm,” Akerman expressed. But how do you computerise biological information? Whilst many models and approaches rely solely on hypothesis context, Envisagenics incorporates intuitive information into its platform and establishes discrete and quantifiable predictive features.
There is a need to build more confidence around AI and reduce the Black Box Effect,” Akerman claimed.
So far, Envisagenics has been able to computerise and articulate value information such as RNA-protein and protein-protein interactions to identify drug binding sites. Akerman also explained how it is preferable to sacrifice a little bit of predictive accuracy for transparency during digitisation. This is because “if we cannot articulate what the features are showing in the predictive model, it will be even more difficult to interpret them retroactively.”
Achieving Results with SpliceCore
Using the SpliceCore platform, Envisagenics has been able to successfully predict splice switching oligonucleotide (SSO) compounds that modulate alternative splicing using molecular interaction data. For this, Envisagenics decided to focus on the spliceosome and investigate its complex structure of networks to uncover “regulatory circuits”, which are the mechanistic units utilised by the spliceosome to regulate splicing.
Envisagenics’ SpliceCore technology has identified a novel drug target for triple-negative breast cancer as well as an optimal SSO binding site.
A total of 32 unique regulatory circuits were pinpointed and leveraged as ML features to predict splicing modulation. This information was then used to predict SSO drug targets, creating a druggability map of antisense oligonucleotides throughout the entire transcriptome. Envisagenics’ SpliceCore technology has identified a novel drug target for triple-negative breast cancer as well as an optimal SSO binding site.
By combing ML algorithms to prioritise splicing events and establishing a modular software technique to register regulatory information, Envisagenics was able to actively provide a transparent methodology and reduce the Black Box Effect. “With this outcome, we were able to qualify these assets in the laboratory, which provides great promise for our other ventures in application to drug target discovery,” Akerman said.
Join Oxford Global’s annual Biologics UK: In-Person event today. This 3-day conference brings together a panel of prominent leaders and scientists, sharing new case studies, innovative data, and exciting industry outlooks.