Study Shows ML Optimises Therapeutic Antibodies
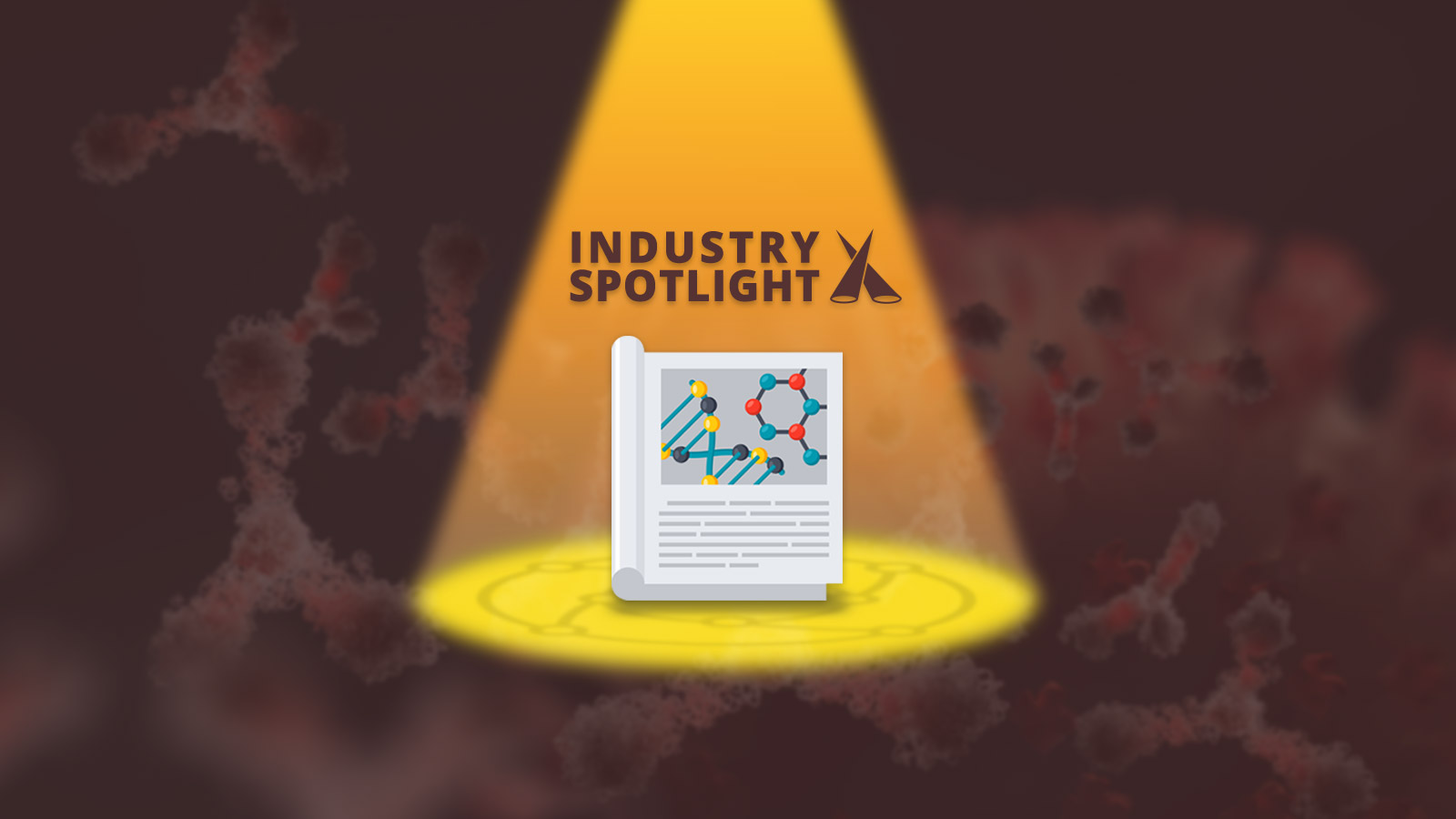
A recent paper published by Nature Biomedical Engineering showcases the efforts of researchers from ETH Zurich, Switzerland, in their development of a machine learning (ML) method to support the optimisation phase of therapeutic antibodies. The researchers showed that using computational deep learning techniques paired with predictive antibody specificity can optimise antibody variants from a diverse range of antibody sequences.
The article, “Optimization of therapeutic antibodies by predicting antigen specificity from antibody sequence via deep learning,” documents the study's aim to achieve and develop more effective antibody drugs.
- Could Targetting Complex Membrane Proteins be an Understudied Opportunity in Antibody Engineering?
- Discover More About Optimising Receptor Trafficking with Bispecific Antibody-Drug Conjugates
- What’s Next for Antibody-Based Therapeutic Discovery and Optimisation?
The ETH set out to streamline the labour and resource-intensive optimisation process by rapidly increasing the number of initial antibodies to be tested. Using Roche’s antibody cancer drug Herceptin, the team applied their ML approach to a database of 70 million potential antibody DNA sequences.
“To produce data for training deep neural networks, we deep-sequenced libraries of the therapeutic antibody trastuzumab (about 1?×?104 variants), expressed in a mammalian cell line,” the team explained (see citation). The ETH then created approximately 40,000 related antibodies whilst applying site-directed mutagenesis via CRISPR–Cas9-mediated homology-directed repair.
Computational deep learning techniques paired with predictive antibody specificity can optimise antibody variants from a diverse range of antibody sequences.
Results showed that 10,000 antibodies bound to the target protein. DNA sequences of the 40,000 were then used to train an algorithm to screen a computational library of approximately 1?×?108 trastuzumab variants. The trained neural networks predicated the HER2-specific subset and were “filtered for viscosity, clearance, solubility and immunogenicity to generate thousands of highly optimized lead candidates.”
The EHT scientists also found that “recombinant expression and experimental testing of 30 randomly selected variants from the unfiltered library showed that all 30 retained specificity for HER2”. They concluded that deep learning could facilitate antibody engineering and optimisation.
Mason, D.M., Friedensohn, S., Weber, C.R. et al. Optimization of therapeutic antibodies by predicting antigen specificity from antibody sequence via deep learning. Nat Biomed Eng 5, 600–612 (2021). DOI: 10.1038/s41551-021-00699-9.
Join us for Antibody Engineering: Online from 27 - 29 June 2022 to take part in a 3-day meeting that delves into emerging technologies in antibody-based molecular engineering, novel & difficult targets, antibody-based therapeutics & a meeting place for experts working within engineering, computational tools and biotherapeutics. For more information visit Oxford Global.