Revolutionizing Drug Discovery: The Power of AI in Drug Screening and Clinical Trials
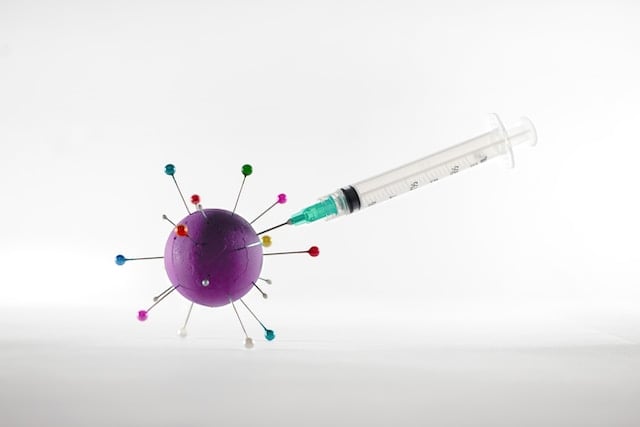
In the ever-evolving landscape of medical science, the utilization of artificial intelligence (AI) has emerged as a game-changer especially in the world of drug discovery
With its incredible potential, AI is dramatically transforming drug discovery and development, ushering in a new era of efficiency and innovation with significant impacts in drug screening and clinical trials.
AI Drug Discovery: A Transformative Force
Streamlining Drug Discovery Processes:
Traditionally, drug discovery has been an incredibly time-consuming and expensive endeavour. Developing a single drug can take upwards of a decade and cost billions of dollars. However, AI is changing this landscape by speeding up the process.
- According to a report by the Pharmaceutical Research and Manufacturers of America (PhRMA), it typically takes 10 to 15 years for a new drug to go from the discovery stage to reaching patients. AI can significantly reduce this timeframe, potentially saving countless lives.
- For example, Insilico Medicine, a biotechnology company, used AI to discover a drug candidate for fibrosis in just 21 days. This is a fraction of the time it would typically take using traditional methods. Such efficiency allows researchers to explore more potential drug candidates in a shorter period.
Enhancing Drug Target Identification:
Identifying suitable drug targets is one of the earliest and most critical stages in drug discovery. AI's ability to analyse vast datasets and find hidden patterns has proven invaluable in this regard.
- Take Alzheimer's disease, for instance. Using AI, researchers have been able to analyse genetic and clinical data to identify novel drug targets. This could potentially lead to more effective treatments for a condition that has eluded us for decades.
- According to a study published in Nature Communications, AI algorithms were employed to analyse genetic data from thousands of individuals, revealing new insights into the biology of Alzheimer's. This innovative approach has the potential to accelerate the development of targeted therapies.
Accelerating Drug Screening:
With AI-powered algorithms, researchers can quickly sift through vast chemical libraries to identify promising candidates.
- Atomwise, an AI-driven drug discovery platform, made headlines by identifying a potential drug for Ebola in just a matter of days. Traditional methods would have taken much longer to reach this crucial discovery.
- This accelerated drug screening is not limited to infectious diseases. AI can also be applied to various conditions, ranging from cancer to rare genetic disorders, paving the way for more efficient and precise drug development.
Personalized Medicine:
AI's ability to analyse individual patient data has opened the door to personalized medicine. Instead of one-size-fits-all treatments, AI can help tailor drug therapies to a patient's specific genetic makeup and medical history.
- For example, in oncology, AI can analyze a patient's tumor genetics to recommend the most effective targeted therapy. This approach minimizes trial and error in treatment selection, potentially improving patient outcomes and reducing side effects.
- The potential of personalized medicine is immense, and AI is at the forefront of making it a reality.
AI in Clinical Trials: A Paradigm Shift
Patient Recruitment and Eligibility:
AI can analyse patient data from electronic health records, identify potential candidates, and match them to suitable trials.
- Trials.ai, a platform utilizing AI, has demonstrated its effectiveness in this regard. By improving patient recruitment, trials can progress more smoothly and efficiently.
Predicting Patient Outcomes:
Predicting how patients will respond to a drug is a crucial aspect of clinical trials. AI can analyse patient data to identify factors that influence treatment outcomes, helping researchers design better trials.
- A study published in Nature Medicine used AI to predict patient responses to immunotherapy in lung cancer. This kind of predictive power can lead to more successful trials and faster drug approvals.
Safety Monitoring:
Ensuring the safety of trial participants is paramount. AI can monitor patient data in real-time, detecting adverse events or anomalies that might go unnoticed with traditional methods.
- This proactive approach to safety monitoring can prevent serious complications and improve the overall integrity of clinical trials.
Data Analysis and Insights:
The sheer volume of data generated in clinical trials can be overwhelming. AI excels in handling and analyzing this data, providing valuable insights that can inform trial decisions.
- IBM's Watson for Clinical Trials, for example, assists researchers in identifying relevant studies and extracting insights from unstructured data sources like medical literature. This accelerates the pace of clinical research.
Real-World Success Stories
Here are some real-world examples that highlight the remarkable impact of AI in drug discovery and clinical trials.
DeepMind's AlphaFold and Protein Folding:
In 2020 DeepMind unveiled AlphaFold (we cover this in more detail here) which is an AI system capable of predicting the 3D structures of proteins with remarkable accuracy. This breakthrough has profound implications for drug discovery, as understanding protein structures is crucial for designing targeted drugs.
- AlphaFold's potential in drug discovery was further underscored when it was used to predict the structures of key proteins in the SARS-CoV-2 virus responsible for COVID-19. This knowledge has informed the development of potential treatments and vaccines.
Recursion Pharmaceuticals and Rare Diseases:
Recursion Pharmaceuticals employs AI to identify potential treatments for rare diseases, a field often neglected due to the limited patient population. By analyzing cellular imagery, AI can quickly identify potential drug candidates for various rare diseases.
- This approach has led to promising discoveries for conditions like Cerebral Cavernous Malformation (CCM), where AI-driven drug screening identified existing drugs that could be repurposed for treatment.
Owkin and Collaborative Research:
Owkin is a company that facilitates collaborative research by securely sharing clinical data while maintaining patient privacy. Their platform uses AI to analyze this data, enabling researchers to draw insights from a wider pool of information.
- Such collaborative platforms have the potential to accelerate the development of treatments and therapies, particularly in the context of complex diseases like cancer.
Challenges and Future Prospects in AI Drug Discovery and Clinical Trials
While AI offers tremendous promise in drug discovery and clinical trials, it is not without its challenges. These challenges include:
Data Quality and Quantity:
AI systems rely heavily on vast amounts of high-quality data. Ensuring that data is both accurate and representative of diverse patient populations is crucial for the success of AI-driven drug discovery and clinical trials.
Regulatory Hurdles:
The integration of AI into the healthcare and pharmaceutical industries requires navigating complex regulatory frameworks. Clear guidelines and standards must be established to ensure the safety and efficacy of AI-driven interventions.
Ethical Considerations:
The use of AI in healthcare raises ethical questions related to privacy, consent, and the potential for bias in algorithms. Striking a balance between innovation and ethical responsibility is paramount.
Interdisciplinary Collaboration:
Effective implementation of AI in drug discovery and clinical trials necessitates collaboration between computer scientists, biologists, clinicians, and regulatory experts. Building interdisciplinary teams and fostering communication is essential.
Despite these challenges, the prospects for AI in drug discovery and clinical trials are incredibly promising.
Advanced AI Algorithms:
Continued advancements in AI algorithms will enhance the accuracy and predictive capabilities of AI systems. This will lead to more precise drug discovery and better patient outcomes in clinical trials.
Integration of Multimodal Data:
AI will increasingly incorporate diverse data types, including genomics, proteomics, and imaging data, to provide a more comprehensive understanding of diseases and treatment responses.
AI-Driven Drug Repurposing:
AI will continue to identify existing drugs that can be repurposed for new indications, potentially accelerating the availability of treatments for various diseases.
Patient-Centric Clinical Trials:
AI will enable the design of clinical trials that are more patient-centric, with tailored treatments and improved recruitment processes.
Global Collaboration:
Collaborative platforms like Owkin will foster global collaboration in research and clinical trials, allowing for the sharing of insights and data on a scale never seen before.
Conclusion
The integration of artificial intelligence into drug discovery and clinical trials is nothing short of revolutionary. From speeding up drug development to enabling personalized medicine, AI is reshaping the pharmaceutical industry. AI is streamlining processes, improving target identification, accelerating drug screening, and enhancing every aspect of clinical trials. The real-world success stories we have examined demonstrate that AI is not just a theoretical concept; it is making tangible contributions to medical science.
The future of drug discovery and clinical trials is undeniably intertwined with the power of AI. Embracing this new technology in drug discovery and clinical trials is essential and necessary for staying competitive and, most importantly, for advancing medical science to benefit patients worldwide and lead to more efficient and effective treatments but also unlock new possibilities in the quest to conquer diseases that have long eluded us.