Computational Drug Design: Applications of AI and Machine Learning in Development
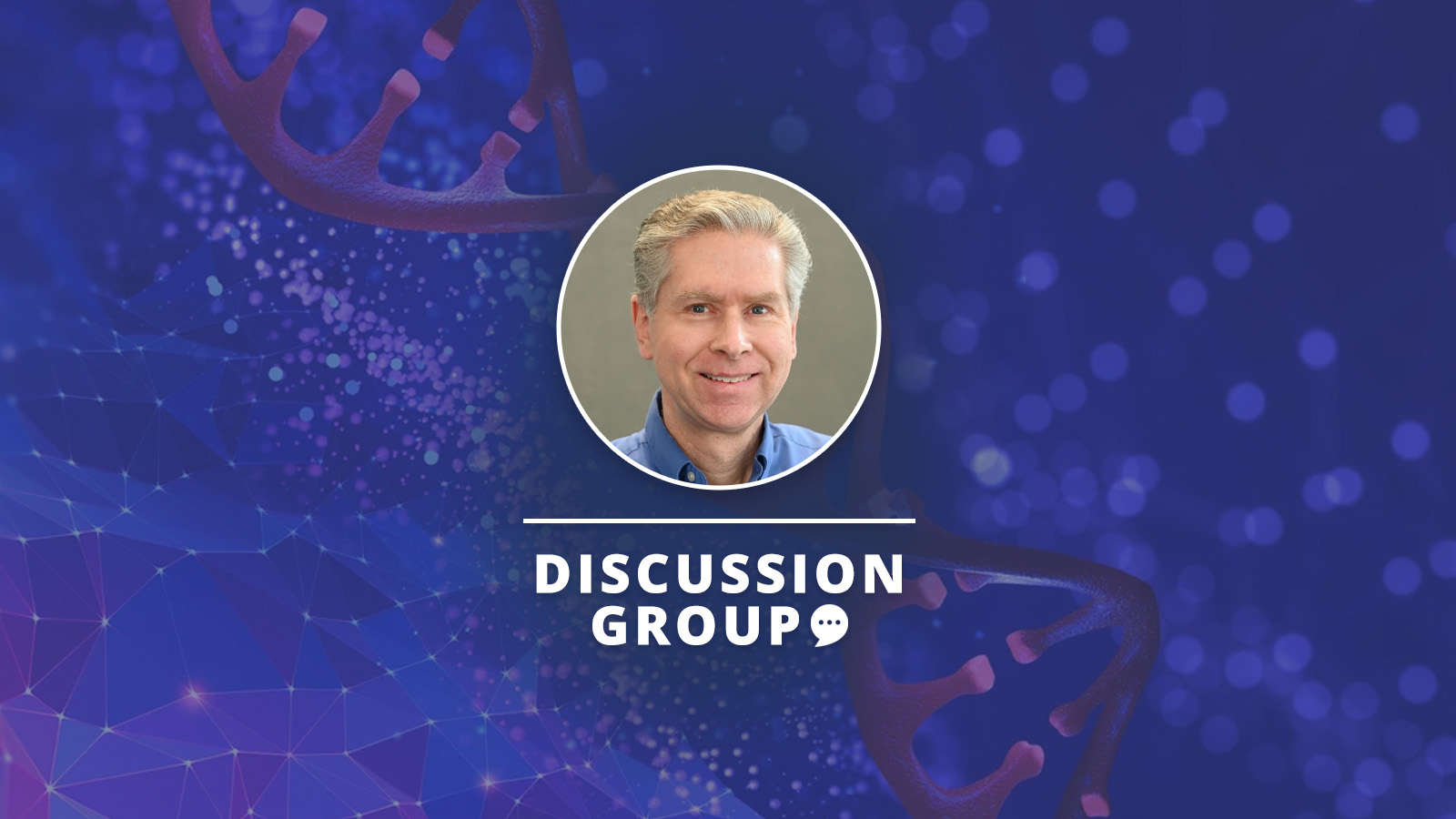
Our October 2022 PharmaTec discussion group focused on the applications of artificial intelligence (AI) and machine learning (ML) in computational drug design, and brought together thought leaders from across the pharmaceutical sphere. The discourse was led by Peter Henstock, Machine Learning and AI Lead at Pfizer, who touched on deep learning processes and the feedback necessary for the optimisation of drug design and development.
Henstock opened the discussion by providing some context on his own background in drug discovery and design. He explained that his focus was on getting AI ‘everywhere’, although he conceded that the effort to implement this approach was akin to ‘pushing a rock uphill’. “People point to the applications of AI in imaging — removing red-eye in pictures, for example — and new approaches with deep learning as proof that neural networks can work within that space.”
“I’m excited about the potential of the AI and structure-based approach to prediction modelling."
Dimitar Dimitrov, CEO at Bolka Pharmaceuticals, explained how his company worked on a pipeline for discovery and novel molecules. “With AI and machine learning, we use our platform and gather that solution in the market,” he explained. “The value of the data is delivered by an applied digital twin approach — we get all the data and we visualise the solutions with every use-case for new drug applications.”
Sung-Hun Bae, Senior Principal Scientist at Eisai US, said that conventional interpretations of drug discovery were traditionally divided into two camps: ligand-based approaches and structure-based approaches. “Over the past few years, a lot of prediction models based on public data or company data have predicted activities for the ligand-based approach,” said Bae. “I’m excited about the potential of AI and structure-based approaches — we have a lot of structural information, but it’s less developed in regard to that aspect.”
Ligand-Based Approaches and AlphaFold Prediction
One early query put to the group by Henstock concerned the process for target selection regarding structural AI in chemistry. One attendee said that obtaining a structure for a target is much more feasible now than it was ten years ago, but that there are still considerable constraints regarding time and cost. “Whereas I think I’m seeing that predictability of structures is becoming much, much better.”
- Read Now: Oxford Global's R&D Key 40 Stories
- Applying AI/ML Approaches to Drug Development Through Machine Learning
- Addressing Challenges for Machine Learning Implementation via Explainable AI
They added that there are cases now in the literature where researchers have predicted for structures prior to running programmes, with the outcomes in computational drug design arriving as more or less the same. “So you’d have a jump of nine to 12 months on your drug discovery program, which for me is a game changer.”
Deep Reinforcement Learning in Computational Drug Design
The next focus of the discussion was on the application of AI and Machine Learning approaches to fast sampling, making use of the structural information. The binding protein target can be derived from ligand structures. “Maybe the AI/ML structures can give us an advantage,” Bae suggested. Another discussion group attendee voiced agreement. “If it fits into a family of targets that structurally integrate then you could use AlphaFold for that,” he said. “It could integrate quite nicely.”
From here, the conversation moved to applications of deep reinforcement learning: a subfield of machine learning which considers the problem of a computational agent learning to make decisions by trial and error. “I must admit I’m quite excited about that area and the ability to engage with deep learning techniques,” said Henstock. Bae questioned the threshold for the minimum number of data points required. “How many data points would you want to feel comfortable in initiating an AI or ML approach like reinforcement learning or active learning?”
Multi-Task Assignment and Building Model Confidence
Discussing his own project, Dimitrov told the group he had invested heavily in an AI model pipeline, which was the result of a significant amount of experimentation. “We do a lot of prediction before we make any experimental in vivo or in vitro data,” said Dimitrov. An example Dimitrov gave was the prevention of cancer metastasis in mice. “We invest a lot of money in prediction, because good prediction comes through investment.”
“This is a really hot area,” said an attendee. “A scientist may come in with a problem and we may not have all the data, or we may have a limited set of data.” They added that researchers may need to take a strategic view on how data is gathered. “Now a lot of work is going into the data that is being gathered, digitised, and made available across the enterprise.”
High levels of confidence are essential to model success when it comes to practical applications for data. “We are focusing on distinguishing high levels of activity from low levels of activity,” they continued. Additionally, they emphasised the importance of conceptualising data on multiple levels and blending them into a more uniform approach.
Computational Drug Design: What Skillsets are Necessary in Chemists Today?
Turning lastly to the qualities that made a strong team of chemists in a modern research and development environment, Henstock observed that computer science and chemistry groups were increasingly merging for different purposes. Dimitrov said that an ideal team would be a cross-set between AI and life science. “When we want to get more innovation, we are focusing on the AI specialists and crossing between life science and AI.” Bae offered that his group has a dedicated member for data and informatics to interpret the information received. “The AI/ML approach for using clinical applications is now easily available using computational chemistry and structural biology,” he continued.
“One thing I’ve heard about a lot in the literature for AI is federated learning,” said Henstock: “the sharing of models or your data with other people for collaboration. Is that something that is happening within the chemistry space?” Another attendee replied by emphasising the need for a consistent outlook across multiple structures. “We expect the payoff to be huge — however, we need some evidence that is applicable not universally but broadly.”
Rounding off, Henstock asked the assembled figures what they were most excited about. Bae said that recent advances in AI/ML regarding chemical applications were much more representative than older configurations. “I think there is huge potential to improve the accuracy and speed of development — I’m really excited about that.”
Want to read more about new and upcoming approaches to drug design and validation? Visit our PharmaTec portal to stay up to date with the latest industry news and developments. If you’d like to take part in one of our future discussion groups, take a look at our PharmaTec community membership options.