AI/ML Approaches in Drug Development: Applied Machine Learning in Clinical Trials
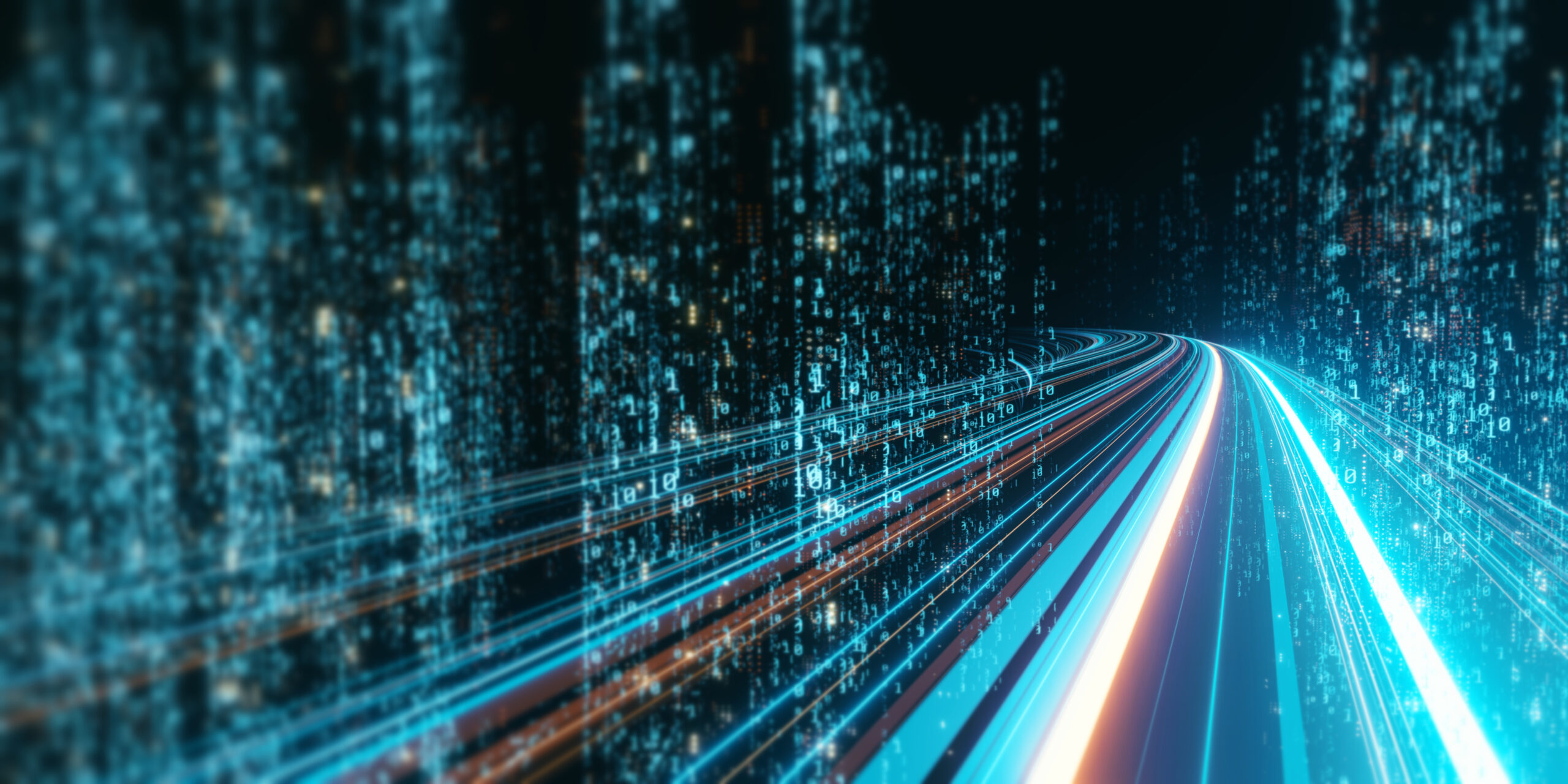
Artificial intelligence (AI) and machine learning (ML) can play multiple roles in drug discovery, subject to the stage at which both are implemented and the way pharmaceutical manufacturers adopt them into their research. Currently, a major focus in industry is on improving the success rate for drug candidates in clinical trials using informatics, with an emphasis on the integration of AI/ML approaches in drug development.
The tracking and usage of RWE has been accelerated by the arrival of the digital era, which has facilitated the collection and storage of vast amounts of health-related data with greater ease. However, technological advancements have also opened the door for an entirely new avenue of drug design and development. In some recent submissions to regulatory bodies such as the FDA, AI and ML approaches have been used to predict the potential safety risk of a drug based on its structure, physicochemical properties, or target affinity.
Opportunities for ML applications present themselves in the early stages of drug discovery and development, such as target discovery, data mining from past and current studies, prognostic biomarker identification, and the analysis of digital pathology data in clinical trials. There are some obstacles, chief among them being the lack of interpretability and repeatability. These are hurdles which will need to be overcome before AI/ML methods can be more broadly applied to drug design and automation. However, the pharmaceutical industry at large is increasingly of the view that AI and applied machine learning will be integral to the acceleration of clinical trials.
Minimum Dataset Size in AI/ML Approaches in Drug Development
A current avenue of discourse in drug discovery is the validity of AI-interpreted data in clinical trials. One key consideration in the application of AI/ML approaches in drug development is the size of the dataset available for interpretation through these approaches. In particular, this is dictated by the minimum size of a dataset sufficient for AM/ML approaches. Igor Rudychev, Vice President of Enterprise Analytics Horizon Therapeutics, asserts that terabytes of data can be developed from a small cohort of patients. From some perspectives, small clinical trials almost lend themselves to personalised medicine due to the greater depth of data and information that can be generated.
- Biobank Frameworks: Utilising Federated Machine Learning to Augment Data Solutions
- Real-World Data in Real-World Applications: Informing Future Approaches to Healthcare Provision
- End-To-End Automation: A Journey Towards Heightened Laboratory Efficiency
For Hongmei Huang, Vice President in Development Sciences at Genentech, a different cohort demographic could potentially exhibit a different response. Clinical trials can be designed so they can be more targeted with personalised healthcare. Data mining can be a suitable approach in this scenario, as it can be utilised to explore the data and find patterns and hints within the dataset. Complications can arise when data is merged from multiple clinical trials, but this may also present greater promises for future implementation.
Integrating AI-Driven RWE Interpretation in Clinical Trials
Some voices in the industry have expressed doubts over the implementation of AI and applied machine learning in this format. At one side of the debate are researchers who want to run more experiments to validate data around drug discovery, whereas AI scientists are keen to implement fully predictive models. Organisations such as the FDA are still eager to see explanations for the results from preclinical and clinical trials, which contrasts with companies who are primarily concerned with validating that the approach works.
A current constraint that could be removed with the refinement of data working approaches is the amount of data cleaning that needs to be carried out before this information can be interpreted. If researchers spend most of their time cleaning data then it eats into time that they could be spending applying that data to disease models. Building accessibility and interoperability into data systems is key to ensuring the pragmatic realisation of its full potential.
One important consideration in this approach is that AI and ML are, ultimately, tools for drug discovery. The right tool should be used for the right job, and any decisions made informed by these tools should be explainable. Maria Del Pilar Schneider, Senior Data Mining Statistician at Ipsen Innovation, also stresses the importance of considering the sources of data when interpreting information through AI and ML approaches. She offers the example of RWD collected in hospitals or health care units through electronic medical records or claims data. Although that information has been repurposed to answer medical questions, it was not collected originally for that reason. As such, data granularity might not be enough to answer all medical questions for specific indications.
New Directions and Considerations in Data Integration
With the limitations of healthcare data integration kept in consideration, many regulatory agencies are becoming more open-minded about data validation. Hongmei Huang said that virtual clinical trials are increasingly a topic of discussion, and mentioned examples where existing datasets had been augmented with applied machine learning prior to being submitted to the FDA for a new label. She said that the FDA would likely always want some data on a drug for safety reasons, but suggested that AI/ML applications could represent a means of accelerating the clinical development process.
There was also the acknowledgement that it can take time for machine learning models to become recognised as reliable within the industry and regulatory agencies. Schneider encourages her cohort to think out of the box and to be bold. Lot of data is becoming available, and that data is needed to test hypotheses and new models.
Another main area of focus within the AI and ML sphere is the verification of data. Hanati Tuoken, Principal Scientist at Boehringer Ingelheim, discusses his experience with biobanks — repositories of human biological materials linked to personal and healthcare information — and the importance of testing data validity. The emphasis here is on accumulating as much information as possible from different biobanks in the shortest amount of time.
Broader Applications of AI/ML Approaches in Drug Development
The recent surge in interest surrounding AI and applied machine learning is not unique to the pharma industry: other fields such as social networks, videogames, and other industries are currently using the technology. In many ways, it is already integrated and impacting day-to-day life. Schneider cautions that ML research is only one part of the equation, and implementing it into real practice is more challenging.
The focus has been, and will remain, on generating cheaper and more detailed data which can be interpreted more easily. What is being seen in the industry is the application of information learning insights for model learning and training. The focus is working on automation processes that arrive at the correct outcome following good science principles with an emphasis on the integration of AI/ML approaches in drug development.
Want to read more about the latest implementation approaches for AI/ML? Head over to our PharmaTec portal for insights from the industry’s best and brightest. If you’d like to learn more about our upcoming Pharmaceutical Mobile Robotics: In-Person event, click here to download an agenda and register your interest.