What is AlphaFold?
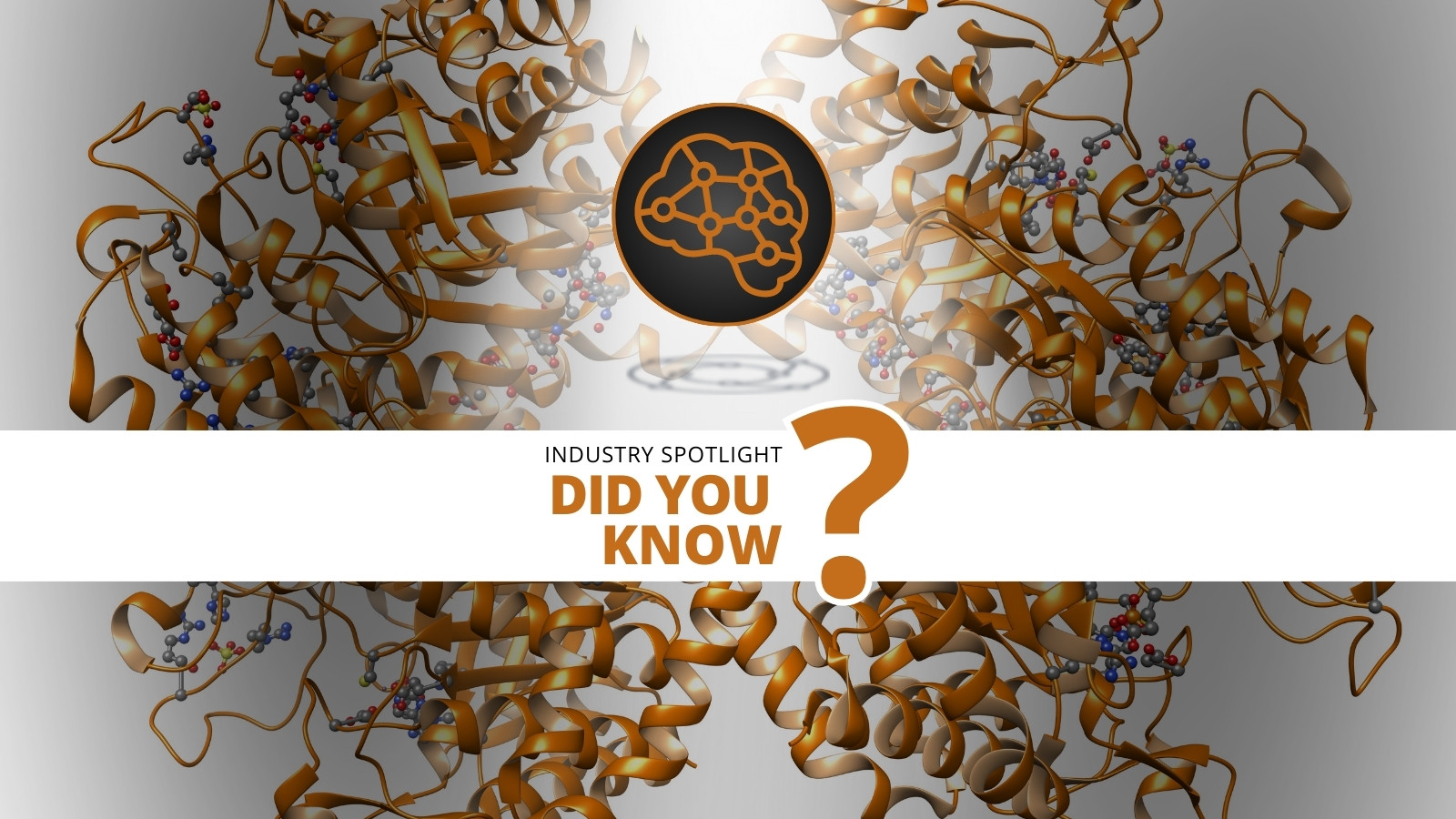
The Protein Folding Problem : a ‘Grand Challenge’ of Biology
A protein’s unique characteristics and function is down to its three dimensional shape or conformation. Therefore, there is a great deal to be gained by figuring out a protein’s structure starting from its amino acid sequence. The final stage on the journey from DNA to RNA to protein is the process by which a protein attains it shape. But how does an unfolded polypeptide become a stable, three-dimensional structure, and can you simulate this process from just an initial amino acid chain?
That’s a question that computational biologists have been trying to answer since the 1960s. The 3D structure of proteins is generally determined through X-ray crystallography techniques but there have been efforts to use computation. Due to the astronomical amount of possible configurations that any one polypeptide chain could arrange itself in, molecular dynamic simulations of protein folding are very limited. For large proteins with explicit water, other methods need to be considered to simulate their folding.
RELATED:
AlphaFold 1
It was clear that solving the ‘protein folding problem’ using computation would take new and innovative ideas. In 1994, a competition to test the various methods of doing exactly that was set up, called CASP (Critical Assessment of Structure Prediction). In 2018, Alphabet company DeepMind entered their contender: AlphaFold an artificial intelligence which would employ deep learning techniques to solve protein structures.
Their approach was relatively successful, the team’s entry to CASP13 in 2018 came in first place, giving the best prediction for 25 out of 43 proteins. Although the team released their code to the public on GitHub, the program is intimately linked to both DeepMind’s internal workings and the CASP13 dataset and cannot be applied to new proteins out-of-the-box.
CASP14 and AlphaFold 2
At CASP14 In November of 2020, the latest edition of DeepMind’s program, AlphaFold 2, demolished its competition. It made the best prediction for 88 out of 97 proteins and achieved a median global distance test (GDT) score of 92.4/100.
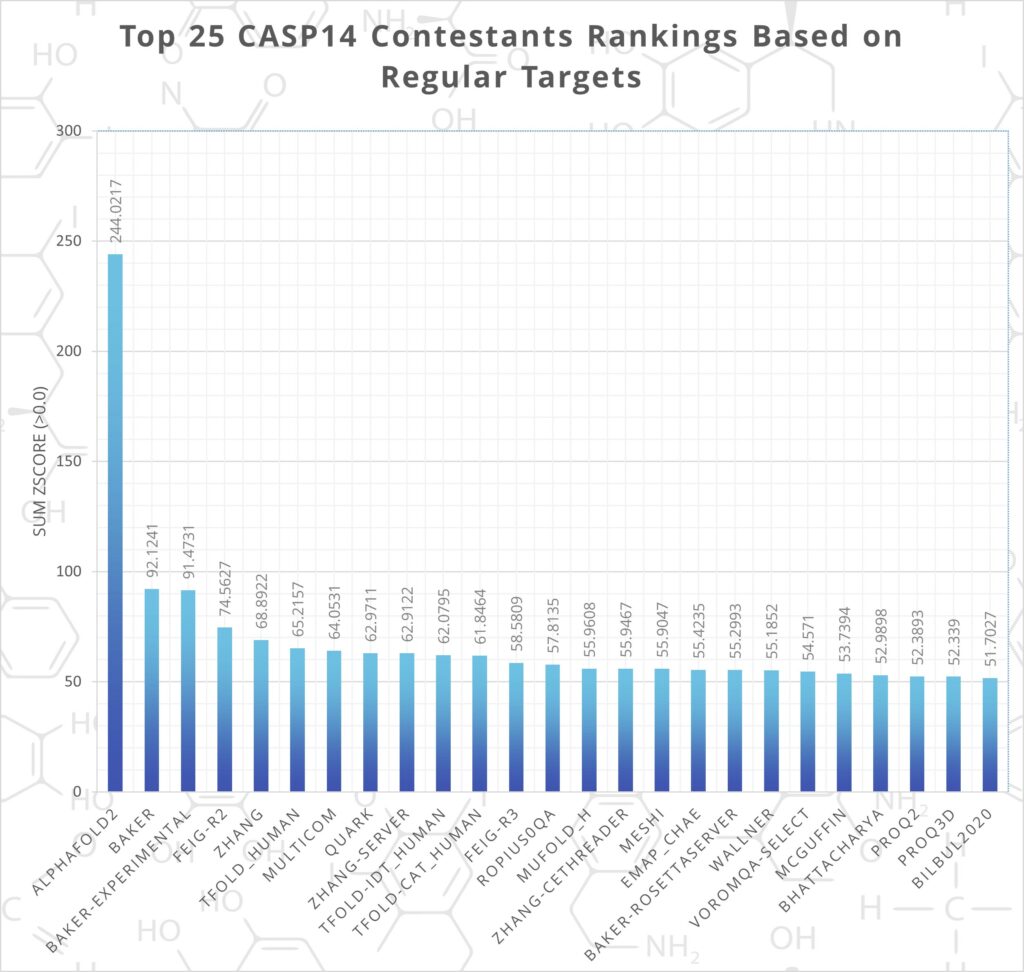
The software design of the new program made significant revisions to the original version, incorporating a system of subnetworks that combined to make a single architecture. Further iterations have been made to the program since CASP14’s success, including the ability to predict protein complexes. Although DeepMind did not enter CASP15, many of the other entrants based their work on AlphaFold 2.
Problem Solved?
So, has AlphaFold 2 solved the protein folding problem? Although it is able to predict the conformations of proteins with an accuracy comparable to practical methods like X-ray crystallography, their are limitations to its success. One problem is the fact that it is trained on already folded proteins, and therefore, its predictions can be optimistic when considering polypeptides that the program hasn’t seen before.
To learn more about AlphaFold, its limitations, and its use in drug discovery:
- AlphaFold and Drug Design: Has AI Solved Biology’s ‘Grand Challenge?’
- AlphaFold: Momentary or Revolutionary?
Get your weekly dose of industry news?here?and keep up to date with the latest?‘Industry Spotlight’ posts.?For other Discovery content, please visit the?Discovery Content Portal.