Using ML with DEL: Philogen and Google Partner in New Study
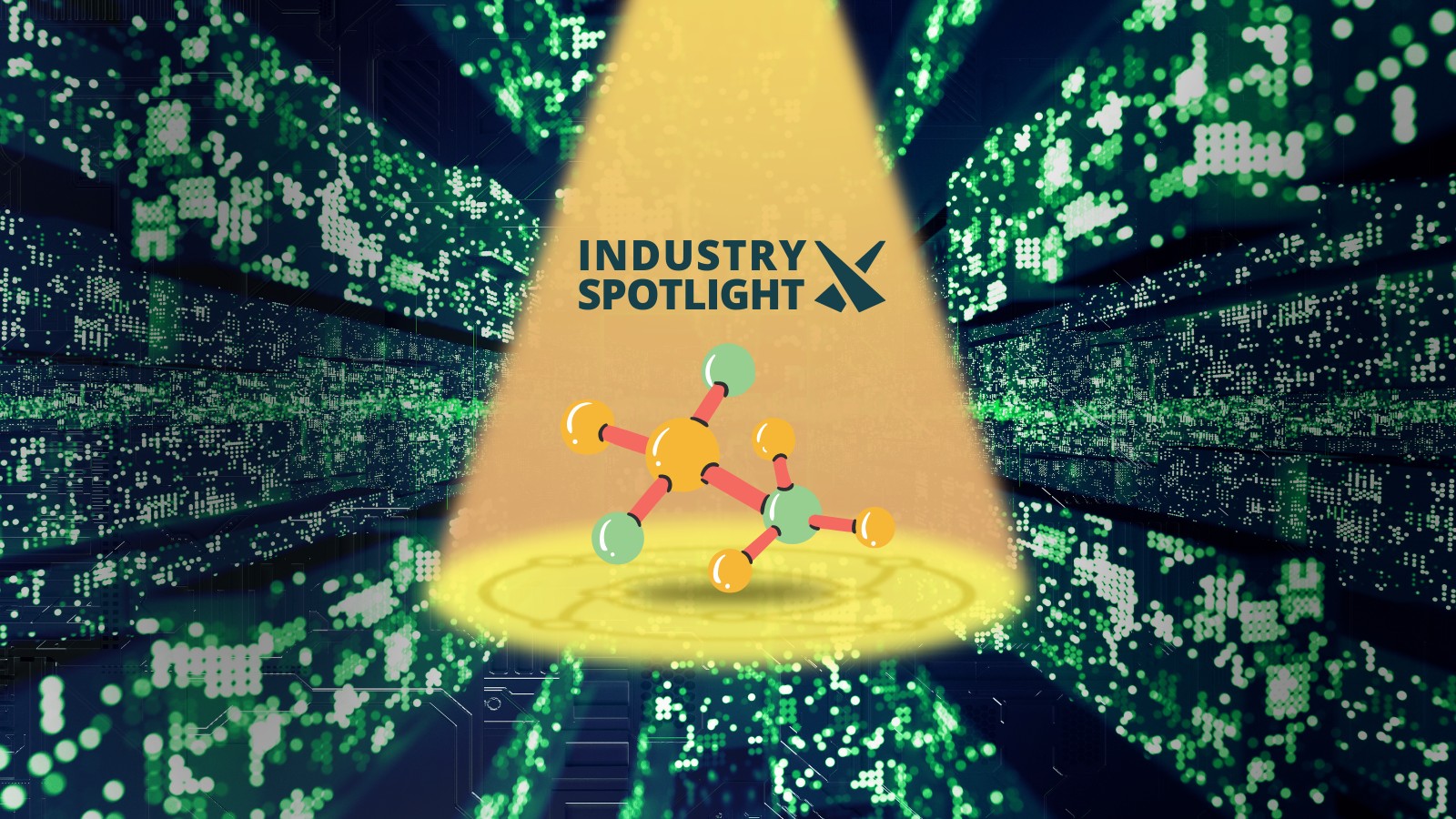
Clinical biotech company Philogen has announced the publication of a collaborative study with Google that applies machine learning (ML) models to HIT finding with DNA-encoded libraries (DELs). Their testing has been able to yield lead compounds with high affinity and high selectivity in preclinical mouse models.
Philogen is a Swiss-Italian biotech that focuses on developing antibody-based and small molecule therapies for deadly and serious diseases. The company uses DEL technology through their subsidiary, Philochem AG.
Dario Neri, CEO of Philogen, commented on the collaboration: “We are excited by the potential of the synergy between DNA-Encoded Chemical Libraries and Artificial Intelligence. The powerful discovery approach that we have developed together with Google should be broadly applicable to additional targets of pharmaceutical interest for the discovery of novel drug prototypes.”
- How DEL Screening is Used in Living Cells
- Target Identification, Phenotypic and Cell-based Screening
- Addressing Specific Targets and Dealing with Target Engagement
What is a DEL?
DELs are gigantic libraries of compounds that can be used to screen against a immobilised protein target. Each small molecule is linked to a unique DNA sequence which helps scientists identify and track it. The efficiency of a DEL screen lends to its low-cost, high-throughput nature as a DEL can contain billions of molecules.
The Method
A DEL screen can return huge datasets in its results due to the vast number of compounds and targets that can be screened at once. Those huge datasets can be used for both affinity maturation and binding characterisation via computational methods.
Philochem and Google have developed a HIT to lead strategy using ML models that are trained on DEL data. They used DEL screening and ‘instance-level deep learning modelling’ to identify compounds that target the cancer marker Carbonic Anhydrase IX (CAIX).
CAIX has low expression on healthy tissues but over expression on cancerous tissues, making the target a clinically validated marker of hypoxia and of clear cell renal cell carcinoma (RCC).
The companies screened CAIX against three DELs and used the data it returned to train their ML models to generate new compounds that were similar to the hits but not present in the original library. Those new molecules were then also screened against CAIX.
They also used their ML model to prioritise the hits it generated for translation into leads. Those leads were tested in vivo against tumour cells that express CAIX.
Results
The team found binders to the surface of CAIX-expressing tumour cells in their cellular binding assays. The strongest result that they found yielded a compound with a binding affinity of 5.7 nM, and preferentially targeted the tumour in in vivo models of RCC.
Get your weekly dose of industry news?here?and keep up to date with the latest?‘Industry Spotlight’ posts.?For other Discovery content, please visit the?Discovery Content Portal.