Real-World Data in Real-World Applications: Informing Future Approaches to Healthcare Provision and Drug Development
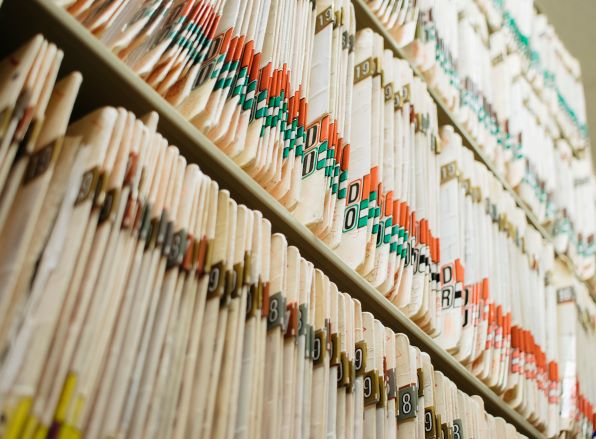
Real-world data — also known as RWD — is used by healthcare and regulatory bodies such as the FDA in the United States to monitor the post-market safety of newly-developed drugs. RWD is generated from existing healthcare data and information collected on the benefits and risks of a drug generated from a source other than traditional clinical trials. Its cousin, real-world evidence (RWE), is the clinical evidence regarding the usage and potential benefits or risks of a medical product derived from the analysis of RWD.
With the amount of user health data generated by wearable mobile devices such as smart watches rapidly increasing in the past few years, new data resources are increasingly available as an additional facet for the refinement of healthcare provision. The recent COVID-19 pandemic stands as a good example of the utility of capturing, sharing, interrogating, and integrating RWE for healthcare treatment. In the case of the pandemic, analysis of real-time electronic health records for routine clinical care resulted in better disease surveillance and produced evidence to inform public health decisions.
The RWD and RWE solutions market is on the rise: in 2022, the market for RWE solutions stood at an estimated USD$1.48 billion, and is anticipated to grow to USD$2.47 billion in 2026. A gentrifying global population with longer life expectancies is anticipated to propel the growth of the RWE solutions market — in other words, RWD-derived information will pose benefits for an elderly population more susceptible to chronic health risks.
Putting Real-World Data into Practice
One of the biggest differences between RWD and more traditional clinical trial data is that populations are much larger and more diverse. For Igor Rudychev, Vice President at Enterprise Analytics and Horizon Therapeutics, this facilitates the exploration of additional research questions. Clinical trial data may come from a dataset of a few hundred patients, whereas RWD population sizes may extend to millions of patients.
Among the manifold sources of RWD, healthcare data can take the form of information collected from routine clinical settings via anonymised electronic health records (EHRs), insurance databases, and patient registries. RWD can help with the exploration of additional research questions and can be used to fill in information gaps on treatment patterns in real medical settings.
The validity of RWE generated from RWD can be invaluable in informing decision-making across different aspects of healthcare provision. One example given by Rudychev involves lifestyle data collected during clinical trials for lung cancer between smokers and non-smokers. Patients who have recently quit smoking will report themselves as non-smokers despite real-world data representing them as hard smokers only a few years prior. By having access to healthcare data structured from RWD, healthcare providers can build a more accurate picture of patient conditions regarding more discretionary datasets.
Challenges in RWD Implementation
Although RWD and RWE represent extremely useful auxiliary approaches to informing patient outlook, there are certain challenges and limitations involved. One is that most aggregate sources of RWD are one to two years old, meaning they can be outdated when it comes to laboratory applications. Additionally, RWD may only provide a partial view of a patient’s journey: there may be missing data if a patient has moved between practices or clinics, resulting in patchy medical records and histories on their part.
- Telerobotics in Healthcare: From Space Robotics to the Mobile Lab Robotics of the Future
- Synthetic Data: Democratising Data Access to Improve Patient Outcomes
- Leveraging Compound and Therapeutic Antibody Analytical Data
A case study Rudychev provided was the emigration of patients abroad on holiday. Some patients from the northern US may move to Florida for the winter and see physicians there — know as snowbirds. If they may be diagnosed by a different physician, the data may be lost. The interchange of data and information between physicians and data labs can also result in some sections of data going missing — most crucially, missing biomarkers or lab results and genetic tests.
The Importance of Data Sharing in Consistent RWD Usage
The holistic integration and importance of data sharing is also key, particularly with coordinated treatments which involve multiple therapeutics. However, as patients often need to travel between wards and hospitals to receive both treatments, data can go missing. Rudychev said that all guidelines and physician reports indicate that the majority of patients receive chemotherapy and radiotherapy together. In spite of this, analysis of the relevant datasets suggests that only some patients receive both therapies together, with the rest receiving either chemotherapy or radiation.
Rudychev asserted that this made no sense since physicians do not administer radiation-only treatments, and that the more likely explanation was that clinical data as not being shared between institutions. He realised that, due to the nature of data aggregation, most large hospitals are reluctant to share their data on radiotherapies, so EMRT data is usually absent in combined datasets.
Using Deep Learning Approaches to Fill Gaps in Data
If some gaps in patient data histories are inevitable, then opportunities exist for filling them in synthetically. An approach championed by Rudychev is the integration of human intelligence using ensemble models. In the context of RWD and machine learning, ensemble models involve the use of multiple data models in concert to provide augmented predictive performance. As Rudychev explained, each ensemble is a very simple model, but there are millions working together.
Rudychev also mentioned expert systems created to mimic data protection officers (DPOs) — highly trained individuals who check internal data compliance within an organisation and who act as contact points for data subjects. The concept involves creating an AI model which mimics an expert’s behaviour, working to imitate the decision-making process in healthcare and treatment scenarios. This process is more repetitive, requiring feedback from the expert helping to pilot the system to refine the field of study, but each iteration aligns more closely with the actions and responses of real-world DPOs.
By combining deep learning approaches with expert systems to fill in gaps in research data, researchers can understand and better predict the progression of disease in patients. In this way, Rudychev hopes data sharing can be used to fill in gaps from multi-clinic treatments such as chemotherapy and radiotherapy. This would be a key priority before any submissions are made to the FDA, or to European or worldwide regulators. RWD is continuing to emerge as a vital source of clinical evidence: for researchers, pharmaceuticals manufacturers and healthcare providers, the challenge is to correctly integrate its use into ongoing and future research.
Want to learn more about the applications of RWD to healthcare provision and future research? Head over to our PharmaTec portal to see more insights from the industry’s best and brightest. If you’d like to learn more about some prime opportunities and challenges in Pharma R&D, click here to register your interest in our upcoming Pharmaceutical Mobile Robotics In-Person event.