Multi-Omics Data Analysis and Management
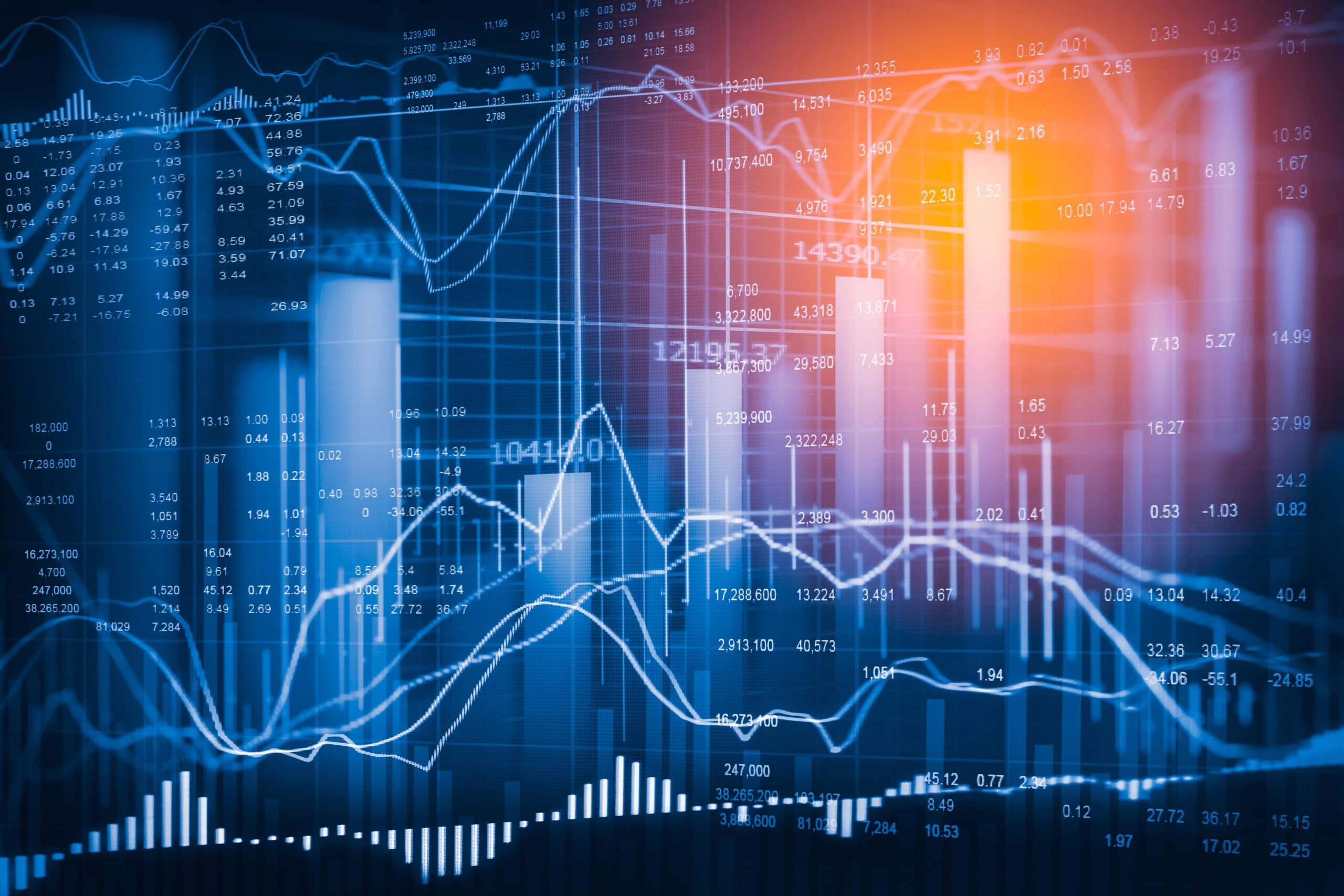
Data is one of the most important assets for a company. The amount of data generated by pharmaceutical companies, particularly omics studies, is enormous. However, over time, it becomes difficult to manage and effectively utilise the vast amounts of data generated daily. Furthermore, with the decrease in sequencing cost, the rate of data collection is expected to increase in the future. For this reason, it’s more important than ever to implement strategies and procedures to ensure researchers can extract value and insights as quickly as possible.
Increasing Collaboration: The Global Alliance for Genomics and Health (GA4GH)
Ensuring that data is interoperable and shareable, not just inside an organisation but across collaborative projects, borders and systems, will require extensive change across the industry. Lindsay Smith, a Work Stream and Clinical Projects Manager for the Global Alliance for Genomics and Health (GA4GH), helps make data sharing easier to achieve.
Smith, speaking at Oxford Global’s PharmaTec 2020 event, expressed her view that “the research and healthcare communities must agree on common methods for collecting, storing, transferring, accessing and analysing data. The Global Alliance for Genomics and Health was established to address this need by developing standards and policies for responsible and secure sharing of genomic and health-related data. We strongly align with the FAIR data principles and philosophies: making data Findable, Accessible, Interoperable and Reusable.”
- Fair Data Implementation: A Worthwhile Investment
- Three Market Trends Transforming Pharmaceutical Data Management
Data Use Ontology (DUO)
One GA4GH standard Smith shared her knowledge on was DUO, or ‘Data Use Ontology.’ DUO allows users to tag datasets with use conditions based on the consent information obtained from a patient.
She explains that “every institution uses unique language in their informed consent forms to describe their dataset’s secondary use restrictions and conditions. Unfortunately, this means that each data access request must be manually evaluated against the data use conditions. Consequently, Data Access Committees typically respond to such requests in two to six weeks, considerably slowing down the pace of research.”
In DUO, there is a hierarchy of terms with two main branches. The first is permission terms that describe what you are allowed to do, such as specific research areas. The second is modifier terms. These are additional requirements or limitations, such as a geographical restriction.
The patient consent is captured through a consent form which gets translated into DUO codes. Smith clarifies that these codes “are attached to a dataset in the data repository. On the other side, as a data requester, you would fill in a Data Access Request stating the specific disease area you are researching. That goes to a Data Access Committee that can match your label with the existing labels in the data repository. So instead of having to read texts or requests, or match different heterogeneous statements, it is a much simpler label-matching process for the Data Access Committee.”
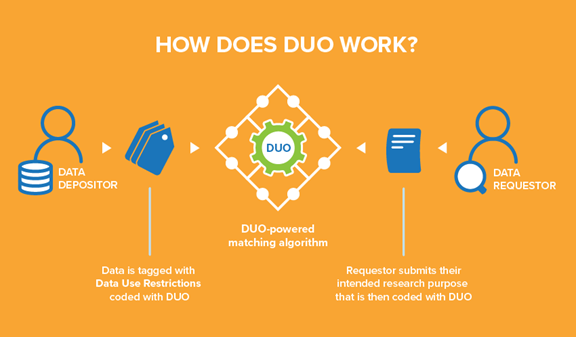
Data Standardisation
Many scientists have been working to establish the relevant data standards for omics data. Due to the complex nature of omics sciences and the varied, ever-changing methods of data collection and reporting, progress has been slower than in other fields
Giovanni Dall’Olio, Data Product Owner at GSK shared some of the progress that has been made at the company. “Previously, our data was scattered across several machines and data storage solutions, and our objective was to put them all together into a data lake. One of GSK’s key goals was to develop a pipeline for this and process incoming data in the same way. So, you have experiments from all departments, groups, and therapy areas in one accessible pool.”
Dall’Olio gave an example of how GSK is doing this with sample requests. He explained that “we standardised the method that scientists use to request a sample. So, previously, every department used a different process. So, what we did was to produce one single entry point, which took the form of a web interface. One of the advantages of this method is that we standardise the captured metadata. We can ask the same question to everyone, and we can also capture metadata about the technical process, such as which platform was used with CRO sequences to sample, and so on.”
Data Processing
The next challenge is to process the raw data that comes from the sequencing in the form of fast queue files into a format that the end users can use.
Dall’Olio explains that “developing one single pipeline for processing all types of RNA-seq dataset is not an easy task. Because there are many options, there are many tools to process sequencing data. Many parameters may be optimised depending on methodology or type of sample. There are so many tools published and updated frequently in the scientific community. So, we need to define which are the best for the most generic cases.”
One way to deal with this problem is to use the concept of functional equivalence for the bioinformatics pipeline. Dall’Olio shares that his team “did was a study of how much changing a specific component of a pipeline will affect the result. So, this means running different variants of the same computation pipeline on the same data and studying how much these changes occur from one tool or the other.”
Cloud Computing & Multi-Omics Data Analysis
Cloud computing has skyrocketed in popularity in the last decade and has become increasingly useful for multi-omics data analysis, storage, and interpretation of omics data. For example, cloud computing can help analyse single-cell lines and cancer samples. Server-based analysis is expected to be crucial in the future of omics data management and analysis.
An example of the move towards embracing cloud computing can be seen in the Cancer Genome Atlas Project. The Cancer Genome Atlas is a joint project of the National Cancer Institute (NCI) and the National Human Genome Research Institute (NHGRI). The project provides one of the largest cancer genomics data sets available through public and private cloud repositories. Analysis of this data has unlocked new understandings of cancer, including discovering that stomach cancer consists of four distinct types rather than a single disease.
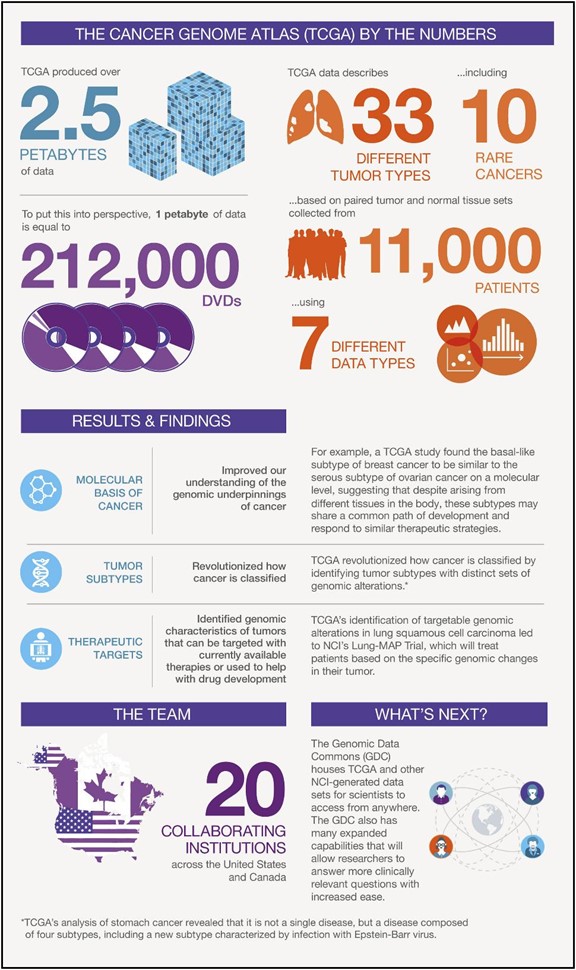
Final Thoughts & Conclusion
Multi-Omics data has the potential to transform our understanding of human biology. These huge datasets comprise gene expression data, transcriptional profiles, proteomic profiles, microbiome sequences and more. Omics data has required many innovations: new forms of data standardisation, data processing, and the application of cloud computing. In order to take this knowledge forward and unlock its true potential, the industry will need to come together, forming collaborations on a never seen before scale.
For more on omics data, please take a look at our dedicated omics content portal. Recent highlights include a discussion group report revolving around NGS data. Finally, consider joining us for NGS UK which will have a dedicated series of discussions and workshops on NGS Data and Bioinformatics.