Machine Learning in Clinical Data Analysis: From Alan Turing to Modern-Day Data Sequencing
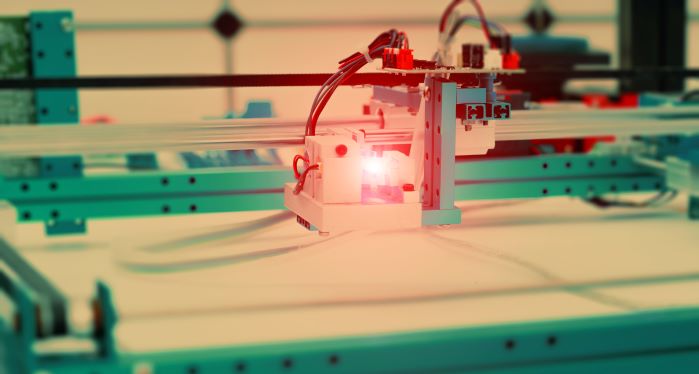
Presented by Maria Del Pilar Schneider, Senior Data Mining Statistician at Ipsen
Edited by Ben Norris
Machine learning can be a powerful tool for augmenting drug discovery, but barriers remain for its broader integration into authentication pipelines. So why should researchers use AI to analyse healthcare data? For Maria Del Pilar Schneider, Senior Data Mining Statistician at Ipsen, the benefits of machine learning in clinical data analysis and data sequencing are enormous.
From Alan Turing to Modern-Day Data Sequencing
Artificial intelligence (AI) is a growing focus in healthcare: it has recently been applied in the areas of ophthalmology and telemedicine, as well as medical devices for disease. 2021 saw the publication of DeepMind’s AlphaFold 2 programme, an AI devoted to predicting protein structures for drug discovery. Its arrival has coincided with a boom in the amount of healthcare data being generated and stored through technologies such as next-generation sequencing.
Broadly speaking, AI is the field of science that deals with the integration of intelligent machines into practical applications. Machine learning is a statistical technique for fitting models to data which can be used to train models with data to make predictions. DeepMind is an example of a deep learning network: a framework which attempts to mimic the human brain and the activities of neurons to make predictions.
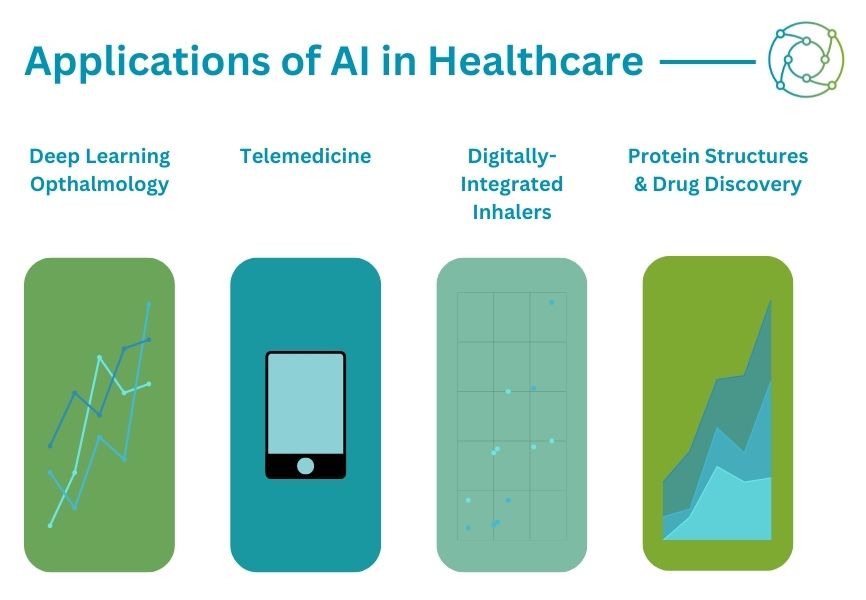
“Machine learning is not new,” Schneider said to the audience at PharmaData UK 2022. “It started to develop in the 50s, and also with Alan Turing.” Some of the biggest breakthroughs and developments have come in the last decade: previous applications had been slowed by limited computing capacity, but recent developments have blown that limitation out of the water. From 2012 onwards, there has been an explosion in the data output of prediction models.
Why Use Machine Learning to Analyse Clinical Data?
A key focus for the recent drive towards applying machine learning to clinical data and data sequencing has been the acceleration of clinical discoveries. “We want to improve the success of clinical trials, which are currently quite low,” said Schneider. A major strength of machine learning in clinical data analysis is its utility in handling large and heterogeneous data sources, such as demographics, vital signs, biomarkers, genomic data, and real-world information. “This process can help us to find new patterns in the data,” Schneider continued. “Here, we have the freedom to explore the data and try to find something we haven’t seen.”
- Read Now: Oxford Global's R&D Key 40 Stories
- Discussion Group Report: Applications of AI and Machine Learning in Computational Drug Design
- Applied Machine Learning in Clinical Trials
Schneider emphasised the importance of the choice of machine learning methods to be used when analysing healthcare data. “It will depend on many factors,” she explained, “including the type of data, the model output, and interpretability.” Schneider also encouraged her audience to consider that some datasets may not necessarily have the key information needed to improve predictions. In terms of computing time, nowadays a major focus in software and hardware development is on improving prediction power and output while saving energy.
Case Studies: Machine Learning in Clinical Data Analysis
One example given by Schneider concerned how machine learning and data mining can be used to improve the design of a clinical trial regarding relevant inclusion and exclusion criteria. “We wanted to identify and characterise patients who were not responding to treatment to see if there were some common characteristics between them,” Schneider explained. First, machine learning algorithms were used to identify baseline characteristics, such as demographics, vital signs, disease history, biomarkers, and disease markers. These were identified as having had the highest contribution to the classification model, and subsequent classification focused on identifying responders and non-responders to treatment.
The objective was to identify and characterise patient subpopulations through parameters evaluated at the baseline by using machine learning algorithms. “We tried to characterise the non-responders and identify some relevant common characteristics between them,” said Schneider. Two groups of patients were identified, one demonstrating a chronic condition and the other demonstrating a severe condition. “The impact is that now the team can use this information to better define the inclusion or exclusion criteria of patients,” she added.
Lessons from Machine Learning and Ongoing Developments in AI
As Schneider summarised, the main constraint across each technique is always the data. “At the end we have a more concise dataset, and nowadays we collect data everywhere, but that doesn’t mean we create access,” she said. “Every country has its own legislation regarding data use and access — for example, GDPR regulations in Europe.” Until international data sharing frameworks are implemented to bring about a more uniform approach to data sharing between institutions, this will remain a hurdle for machine learning in clinical data analysis.
“We need data, but people as a society are concerned regarding what will be done with that data.”
The main focuses of current machine learning and AI research and development will include testing clinical scenarios to identify the patients who will benefit most from treatment. Schneider also feels an imperative to increase society’s knowledge about data use and the benefits of AI to assess potential ethical concerns which may arise from this. “We need data, but people as a society are concerned regarding what will be done with that data.” She closed by asserting there is a need to increase transparency and understanding of the benefits of data sharing and AI technology.
Want to read more about the potential applications of AI and ML in drug development? Head over to our PharmaTec portal to get the latest insights on new and upcoming approaches in data analysis. If you’d like to register your interest in Pharmaceutical Mobile Robotics: In-Person, visit our event website to download an agenda.