Leveraging Compound and Therapeutic Antibody Analytical Data
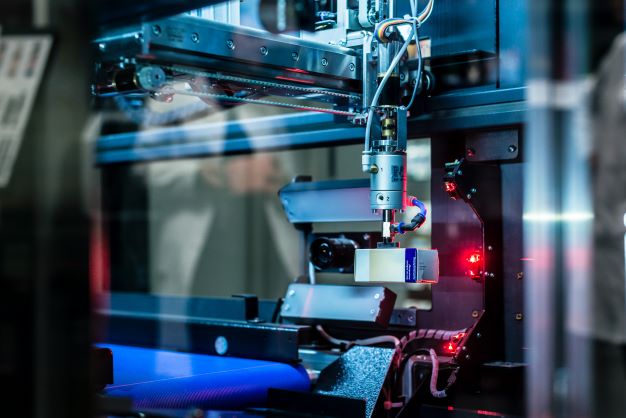
Presented by: Dr. Felipe Albrecht, Senior Scientist, pRED Data & Analytics, Roche
Edited by: Oliver Picken and Ben Norris
Plastic cups and analytical data share a few similarities: neither should be seen as disposable, and both benefit from efforts to improve their reusability. By creating successful end-to-end laboratory and data workflows, companies such as Roche are aiming to increase their data efficiency and productivity. Felipe Albrecht, Senior Scientist at pRED Data & Analytics at Roche, explained his aim to ‘free’ data and unlocking its value for lab scientists, data science, artificial intelligence (AI), and machine learning (ML).
Data Production and Value Generation
Felipe Albrecht opened his presentation at our PharmaData & Smartlabs 2021 event with a concession to current trends in data analysis. “I think we are aware of the reduction in R&D productivity,” he surmised. By the close of the 2010s, R&D returns had fallen to their lowest level in almost a decade, down from 10.1% in 2010 to 1.9% in 2018. “What can we do from the IT perspective to improve this?”, Albrecht queried.
Various levers exist which can be used to augment and accelerate digital drug discovery, including digital workflow automation, just-in-time operations, and augmented drug design. A major focus is the FAIRification of preclinical data, along with approaching the data on a pragmatic ‘as and when’ basis.
One of the key issues at present is that preclinical analytical data is currently scattered across different systems which are not interconnected: there is little potential for researchers to leverage insights from the available analytical data. Molecular information is generated in the analytic analysis, where the data is generated for answering specific scientific questions, and then it is stored in an archival system.
“Usually, the data is stored in siloed repositories – so analytical data is generated and then stored in a shared folder,” said Albrecht. “That’s how data siloing works.” The captured data is then subject to compliance requirements, as it needs to be accessed at later stages. The way this data is perceived is integral to future usage and applicability. But what are the main frameworks which exist to oversee this management?
The Dichotomy of Analytical Data Value
An important aspect of the discussion around the FAIRification of data which is sometimes overlooked is that mechanisms for data collection and storage are already well-established. “We have the existing IT systems – which are good enough,” said Albrecht. “They provide a means to analyse the data, store and share the results.” However, this generated data is often treated as an experimental by-product that does not bring additional value to the research process.
“We create the analytical data to answer some chemical and biochemical questions,” Albrecht continued. “They are not bringing extra value when we look at the data outside of the project.” He emphasised the need for powerful lab and data capture workflows, where the data is handled with proper care and forms a key focus of the research process. “We should view the generated analytical data not as a by-product of the research process that you have to store for compliance, but as something you look forward to generating in order to bring more value in future, there is a lot of knowledge in this data,” said Albrecht.
- In Conversation With Dr Maya Natarajan: Pioneering Graph Analytics
- FAIR Metadata: Management and Principles
- Democratising Data Access to Improve Patient Outcomes
By utilising solid metadata descriptions, researchers can organise their data into a position where experts or data scientists can access the database and quickly have access to the data they are looking for. Following these principles, data itself should be provided with ‘smart searching’ methods to assist in locating the required information. This data should – ideally – be easy to access through reanalysis. The first step towards encouraging data reuse is to stop viewing it as disposable.
Paths for Leveraging Analytical Data
After outlining these goals, Albrecht emphasised the importance of empowering better data reliability with a focus on FAIR principles. These principles are findability, accessibility, interoperability, and reusability, with a broad view to optimising for scale. “These are generic terms, but they offer good guidance,” Albrecht said. “It is still necessary to implement their principles in lab workflows.”
One of the initial steps in the journey towards fully realised data FAIRificiation is the organisation of closed format files with proper metadata, as Albrecht explained. “We must organise data based on its metadata, and go further, where we can use semantic models in the metadata. So, the data itself is not just a bunch of numbers but something with meaningful impact, content, values, and semantics.” A major focus of reusing the data is supporting open standards for storing the data content. Currently there are two main open standards for analytical data: AnIML and Allotrope.
AnIML is a textual data format – information is stored in XML file format, with controlled vocabularies at different levels of implementation, making it simpler and quicker to use. Allotrope is based on the HDF5, which is a binary data format. It sorts the data using ontologies and semantic content regions, and has different components that are more sophisticated and complex. “AnIML in my opinion and experience is simpler and quicker to use,” continued Albrecht, “but the major programming languages have libraries that we can access allotrope data.” Albrecht suggested that, regardless of the method of choice, freeing data for analysis is important for broader holistic investigation. “The first step is ensuring all data is in one standard, AniML, Allotrope, or even an in-house format.”
Data Solution and Approach
“This discussion between external development or in-house development is an old discussion,” Albrecht said. The advantages to in-house development make it a more desirable option for Roche given their experience with lab automation. “We improve our knowledge and leverage that automation,” added Albrecht. By pursuing this approach, Roche can develop their own in-house data solution and build internal knowledge on AnIML and augment their internal lab automation expertise. But for this to work, it is important to work with experts that bring new knowledge and insights.
The LAD (Leveraging Analytical Data) project, with its platform MAX (Molecular Analytics Exchange) provides a comprehensive end-to-end modality. The MAX platform benefits R&D productivity by ensuring a stable contribution to the analysis dataset. As Albrecht said, “You can use the data many, many times, so the value is much higher.” New pharmaceutical molecules take years in the development pipeline – by supporting the analytical data capture access, researchers can reduce the time taken in future experiments by using the existing data as reference and controls.
Rounding off, Albrecht emphasised the importance of focusing on the capture of data and metadata. “When you think about data reuse, and data capture, the annotations for the metadata should be strong.” He described the FAIRification process as a long-term journey in addition to being an IT project. “We IT experts must work closely together with the lab scientists, as one team. Listening to their needs and suggestions but also providing good IT solutions in a timely manner. Also, data must be correctly handled and be available to different groups – it must belong to the organisation and not be siloed at one lab or department. After this, we must work to unlock the data from binary formats, but we must not do it alone, but to work together with different companies and partners.”
Want to know more about the latest trends in the industry and the arguments surrounding data standardisation and analysis? Head over to our PharmaTec portal for the latest insights into FAIR data and lab digitalisation from some of the industry’s best and brightest. To register your interest in our upcoming Pharma Data UK: In-Person event, visit our event website.