Leading Perspectives on Using AI Tools to Drive Drug Design
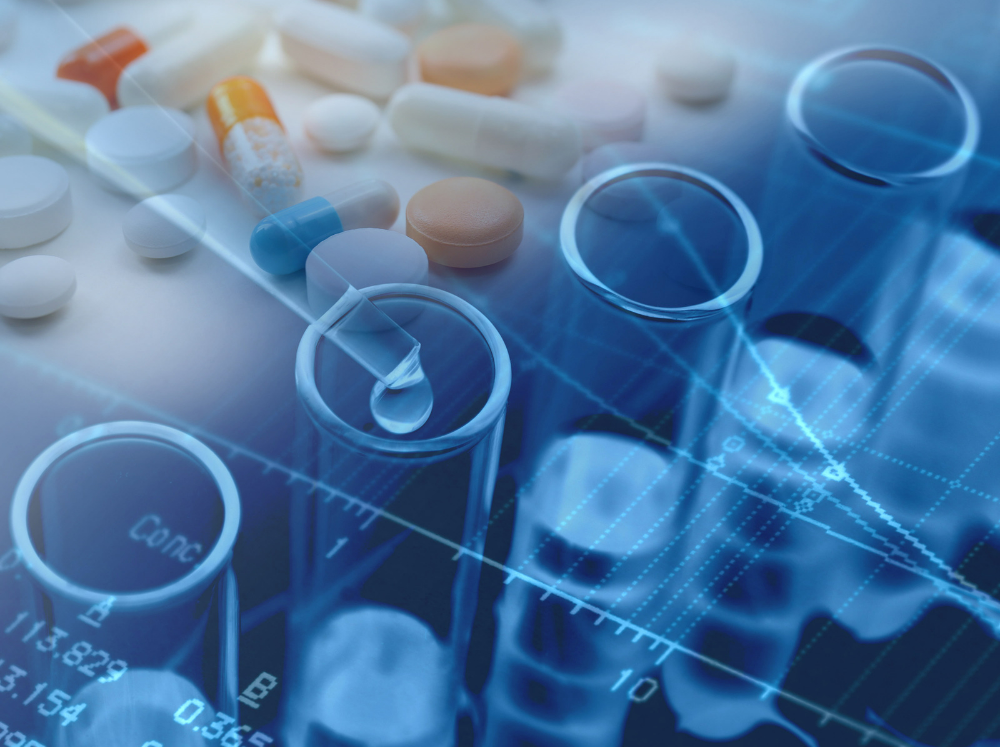
Artificial intelligence has, in recent years, jumped off of the pages of science fiction and into science journals. Among the many applications of the technology, harnessing artificial intelligence and machine learning (AI/ML) methods to aid drug design has prompted both expectation and interrogation.
Tools including generative chemistry, automated synthesis, and AlphaFold, have acclaimed potential to lay the tracks from crystal structure to designed drug, explained Govinda Bhisetti, who moderated the AI Tools and Drug Design panel discussion at Discovery US 2022.
Bhisetti is Vice President of Computational Chemistry at Cellarity. He was joined by Eric Martin, Director at Novartis, Istvan Enyedy, Senior Director of Computational Chemistry at Theseus Pharmaceuticals, and Andrea Bortolato, Director of Drug Discovery, SandboxAQ. The group came together to address the use of AI/ML technologies applied to drug design from a variety of perspectives.
Using Predictive Models
Martin began the discussion by outlining his work in generative chemistry projects. He has worked on a collaboration with Simulations Plus, a software company that develops ML aided tools for absorption, distribution, metabolism, and excretion – toxicity (ADMET) prediction. Simulations Plus’s software uses evolutionary AI technology to generate their predicted structures, as opposed to a forward synthesis or deep neural network-based methods.
- Alphabet’s Calico and Terray Tx Announce Partnership to Tackle Age Related Disease
- $1.2m Quantum Computing Drug Discovery Grant Secured by Penn State Researchers
Understanding when generative chemistry is most useful is vital for its implementation. According to Martin, he encountered a dilemma when de-briefing his chemistry team: “if you’re in an early-stage project, there is not enough data to come up with good predictive models.” And so, the team may be able to generate the compounds just fine but find it problematic to score them.
Moreover, later stage projects often have so many constraints that using virtual libraries instead negates the need to use generative chemistry — "at least of the de novo kind, although perhaps the forward synthesis kind would be helpful there,” added Martin. He concluded that the ‘sweet spot’ for generative chemistry was its use in next-generation projects: molecules generated through medicinal chemistry that have a lot of data to go with them.
Describing this ‘sweet spot’, Martin said, “maybe it was successful in preclinical studies but now you have a new indication; or maybe it was unsuccessful because of a surprising tox finding and now you need to find a new alternative backup series.” In those cases, Martin said that there was enough data to make worthwhile predictive models while there being enough flexibility within the chemical space to make generative chemistry useful.
Compounds that are recommended by generative chemists are then taken into a Simulations Plus program called MedChem Designer. The software uses a ChemDraw-like interface which allows the chemicals to be modified interactively according to the chemist’s experience. By tweaking the compound, the program will then generate a dashboard of model predictions.
Martin said that “almost all of the compounds that were suggested by the program were tweaked in some way by the medicinal chemists.” The capacity for human interaction with the generated molecules was appreciated by the project’s teams, allowing for more flexibility and synergy with the chemist’s expertise.
Predicting Pharmacokinetic and Toxicological Properties of Compounds
Enyedy’s experience with computational drug design included working on automation when he was working at Biogen. “There is a lot of effort across the drug discovery process to automate every step,” he said. For example, Enyedy described information mergers: “grabbing data from various resources to prioritise targets or find issues with compounds.”
Furthermore, Enyedy talked about automation of protein structure prediction, “that’s where we use AlphaFold to automate building a model.” Previously, finding the best template and optimising sequence alignment was done manually, then structures were built using homology modelling, but Enyedy pointed out that “if you aren’t careful, the homology models can be useless.” That being said, AlphaFold is a tool in its early stages which means it has deficiencies, for example its success is contingent of the amount of information available to build a model.
Once a target is selected and its structure is available to the chemistry team, the next stage is to automate generating compound ‘ideas’. “I have been using SYNSPACE for over a year now and it has been very useful to generate makeable compounds,” said Enyedy. The technology also generates synthetically feasible compounds which can help a CRO cut back on the time it takes to make the compound.
“Once we have generated our ideas, we can prioritise them using ML models based on the data,” explained Enyedy. At the beginning of the project, there may not be much data to use for this, so in these cases structure-based or pharmacophore-based models can be utilised for prioritisation.
Enyedy mentioned that using pharmacophore-based models could seem counterintuitive but explained that he had done something similar before. While working as a postdoctoral researcher, Enyedy investigated dopamine transporter inhibitors, looking for compounds that would treat cocaine addiction. “We used cocaine as the starting point and were able to use a pharmacophore built on it to prioritise compounds that showed good activity in animal models,” he explained.
Enyedy concluded that the main area in which ML models are useful and fairly mature is for predicting pharmacokinetic and toxicological properties of compounds. In his assessment, the next step toward ‘true AI’ is to put all the automated components together in one platform — “one that can take a target and generate a drug, making its own decisions along the way.” He added that implementing decision-making into the platform will incorporate statistical analysis: “the software needs to figure out what to do next based on statistics.”
Using AlphaFold for Construct Design
Bortolato uses AlphaFold for construct design before crystallography, which he says he has found very successful. “If you have a new protein, there’s no Xray, and you want to understand what’s the best construct, AlphaFold can help.”
In some cases AlphaFold can be used for free energy perturbation (FEP) calculations, but with mixed success. According to Bortolato, “retrospective evaluation of FEP using AlphaFold in some cases went well, but others it didn’t, so I would say you just need to do your validation before that.”
In Bortolato’s opinion, the best solution for generative models is AstraZeneca’s open-source software called REINVENT, which he said was “really easy to use and constantly improving.” The program is based on the same principle as the GTP-3 AI model that generates text. “It generates ‘text’, but that text is actually a SMILES definition trained on ChEMBL,” he explained. REINVENT’s learning is reinforced by a composite scoring function consisting of user-defined components. Bortolato said that defining the reward function is the difficult part of using the platform and was “critical for designing compounds that reach their target.”
Join and network with over 200 industry leaders at Discovery US: In-Person, where we will address the latest advancements in target identification, validation and HIT optimisation.