Merck KGaA: Accelerating Drug Discovery with Automation and Artificial Intelligence
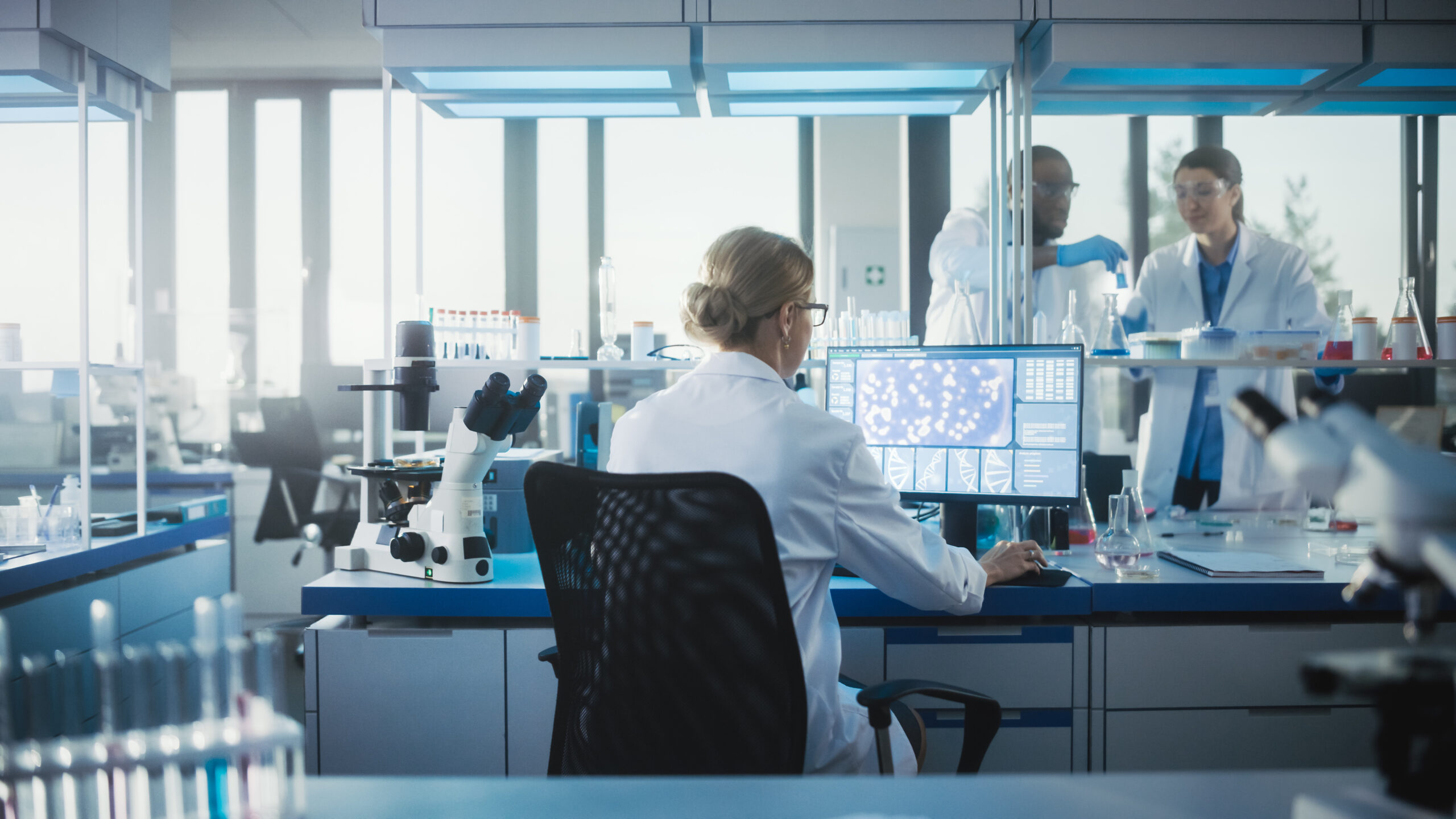
A problem that makes drug discovery so time-consuming and costly is that chemical space is so enormous. For pharma scientists, finding a molecule with effective properties in the body can often feel like finding a needle in a haystack.
This is an area that researchers are increasingly using new technology to help them solve. Novel computational methods have been shown to be effective in narrowing down the unmanageable pools of data in big pharma’s libraries, selecting only the compounds worth considering.
Friedrich Rippmann is the Global Head of Computational Chemistry & Biologics at Merck KGaA, a company working on streamlining its supermassive database of small and large molecules. Rippmann expanded on Merck KGaA’s process in his presentation at Oxford Global’s 2021 Online Drug Discovery Week.
From Humble Beginnings to Automated Endpoints
Rippmann likened the development of drug discovery methods to how car manufacturing has progressed since its beginnings. Much like how drug discovery started, car manufacturing was an artisan’s craft—often the project of small teams of engineers in modest workshops.
Similarly, drug design used to take this niche and custom-made approach where chemists would work on medicinal projects in this artisan way. But the world of car manufacturing did not stay this way for long, and neither has drug discovery. Medicinal chemists now use bulk methods such as high-throughput screening, much like the assembly lines that produced cars on a larger scale.
This leads Rippmann to point out that the future of drug design is heading in an analogous direction to how engineers currently tackle car manufacturing. That goal is to take advantage of the superior efficiency of machines for drug discovery using automated processes and computational techniques.
Rippmann says that the ultimate upshot is that “computational and medicinal chemists are spared from tedious work. Rather, they can concentrate on devising strategies and analysing and interpreting results. AI for me rhymes with Assisted Intelligence.”
The AI and Automation Techniques That Merck KGaA is Using
Many factors should be considered for a drug candidate to be effective. It’s not just about its activity in the body; a drug must also adhere to factors such as selectivity, solubility, stability, permeability, and non-toxicity, among others. So, Merck KGaA needed to find a way to find the chemicals which suit these factors before they become a drug candidate.
Drug discovery at Merck KGaA works in a filtering process. MASSIV (Merck Accessible Inventory) is an aptly named library of over 1020 different compounds, a database so extensive that screening requires the help of machines to find leads and hits.
The vast database is then filtered using Merck KGaA’s MOCCA platform (Merck Online Computational Chemistry Analyser). MOCCA is a prediction tool that can make on the spot suggestions about compounds, either pulled from the database or hand-drawn by researchers.
The platform uses a web-based front-end and graphical user interface, making it straightforward to conduct in-silico profiling of small molecules using predictive models. Researchers can then modify the compounds in-silico based on the software's predictions until they are happy with the results.
FEP+ (Free Energy Perturbation) is a powerful tool used to simulate ligand interactions between compounds and target proteins. Rippmann explained that, until recently, the hardware to model these interactions did not exist on a large enough scale in an industry setting. However, new advancements in graphics processing hardware have made these techniques viable.
The procedure boasts it can “cut through the vastness of chemical space” with the ability to analyse billions of molecules, filtering them down to a few dozen, and then calculating binding—all in a week. “When it works, it can give you a good estimation of the relative binding constant,” says Rippmann.
“Algorithms Inventing Truly Novel Molecules.”
However, the technology that Rippmann is most excited about is generative models, “algorithms inventing truly novel molecules.” Generative models use existing large databases of molecules as training data to generate entirely new molecules towards specified target functions.
Although a very new area of research, it is not as innately science fiction as it sounds. It’s a field that continues to expand, and Rippmann believes that the most critical breakthrough will be integrating those models into a more comprehensive automated workflow.
- Artificial Intelligence for Drug Design and Discovery
- Pharma Manufacturing: Digitalization and Industry 4.0
However, Rippmann considers it essential to advance this field further by simulating discovery and optimisation in 3D space. “Generative models in 2D space are nice to get novel ideas,” says Rippmann, “but they need to be checked in 3D, i.e., in the targeted protein cavity.”
The third dimension is something that current generative models cannot deal with accurately, but Rippmann is optimistic about its future prospects. Merck KGaA is currently working on its integration into automatic workflows. As Rippmann hinted, “stay tuned.”
The Future: Accelerated Development and Lower Attrition Rates
Rippmann says that these technologies are ultimately about the acceleration of drug discovery and lowering attrition rates. Advancing the field of drug development is ultimately about hope. Many people with rare or untreatable diseases rely on this acceleration as their source of hope. So, to them, AI and automation might be what turns that hope into confidence.
So, what’s next? Rippmann points to a significant triumph in artificial intelligence from DeepMind. He is “convinced that both small molecule and biologics design could use AlphaFold-like approaches. If we see the small molecule as a number of non-natural side chains, and then fold it together with the target protein.”
AlphaFold 2 has been on everyone’s minds since it made the best predictions in 2020’s CASP14 competition. Many computational biologists wonder where this powerful technology is going to take medicine; Rippmann’s suggestion could be the answer. “Much work still needs to be done in that direction,” he said, “but the perspective is clear.”
Join us at our next Discovery Europe conference to see the inventive ways in which the field of drug discovery is developing. Don’t miss a thing by signing up for our newsletter to access our latest Insights, Commentaries, and Discussion Group Reports.