AI Model Signifies 'Paradigm Shift' for Traumatic Brain Injury Risk
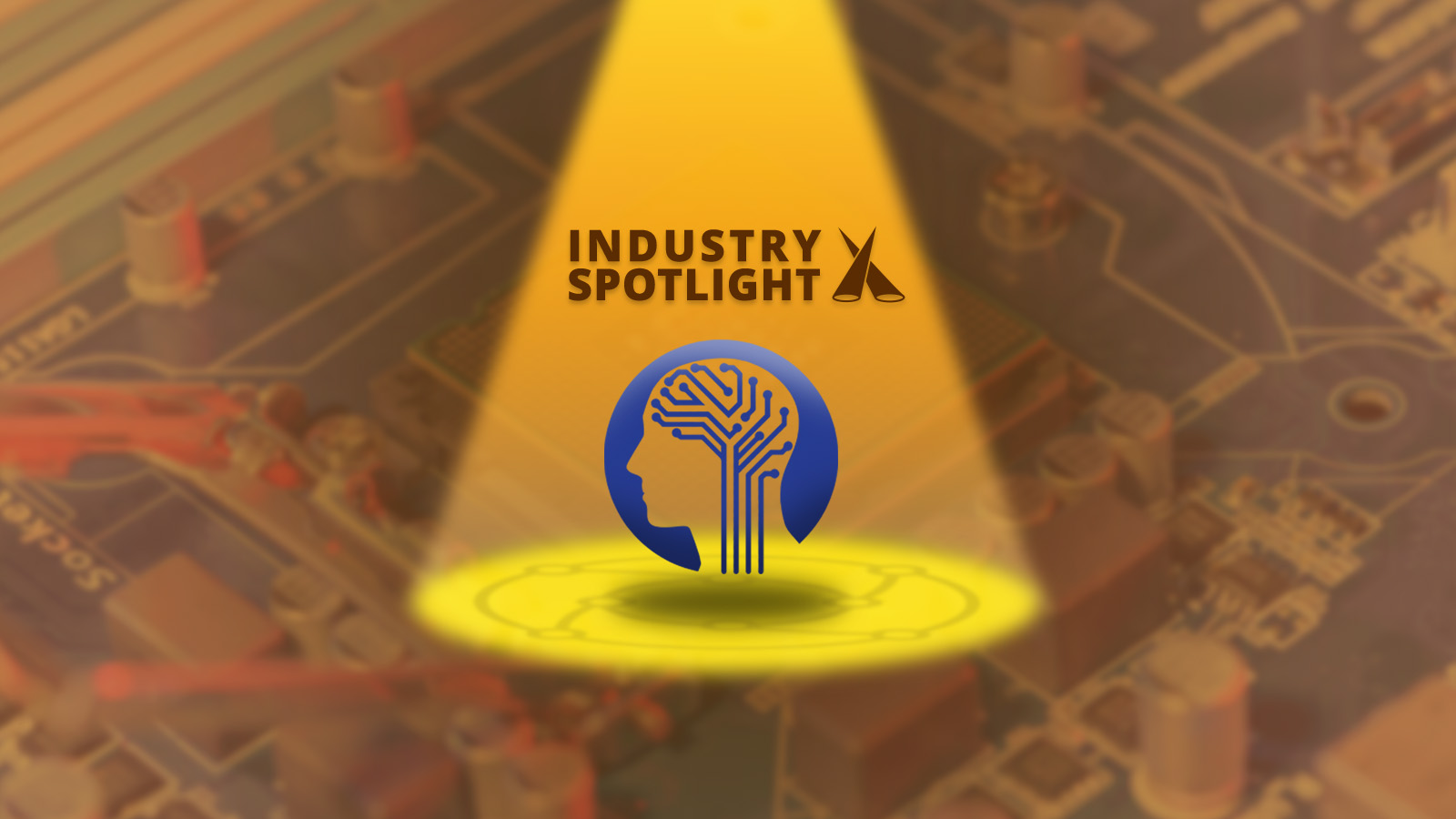
An official press release issued on March 1st by Stanford University has announced its success in leveraging artificial intelligence (AI) to help identify an optimal computational model for indicating the mechanical stress on brain tissue caused by brain damage. Their findings may help to drive the accuracy of understanding why some traumatic brain injuries lead to long-term damage and why others do not.
The model in question is called the Constitutive Artificial Neural Networks and adopts a “model-of-models” methodology to establish the most appropriate material model for explaining brain behaviour. It evaluates over 4000 possibilities, helping researchers understand the mechanical forces that cause stretching, compression, and twisting in the brain tissue.
- Machine Learning in Clinical Data Analysis: From Alan Turing to Modern-Day Data Sequencing
- AI/ML Approaches in Drug Development: Applied Machine Learning in Clinical Trials
- Explainable AI: Addressing Challenges for Machine Learning Implementation
Ellen Kuhl, Professor of Mechanical Engineering and Director of the Living Matter Lab, is the senior author of the study proposing the Constitutive Artificial Neural Networks. In the official press release, she explained, “The problem in brain modelling to date is that the brain is not a homogeneous tissue – it’s not the same in every part of the brain. Yet, trauma is often pervasive.
“The brain is also ultrasoft, much like Jell-O, which makes both testing and modelling physical effects on the brain very challenging.” The Constitutive Artificial Neural Networks differs from conventional off-the-shelf neural networks due to its ability to provide novel insights into the brain’s physics.
In particular, the lab has identified ‘physically meaningful’ parameters relating to brain damage risk. These parameters include varying shear stiffnesses in the cortex, basal ganglia, corpus callosum, corona radiata — at precisely 1.82, 0.88, 0.54, ad 0.94, respectively.
In describing the computational methods behind such a discovery, co-author and doctoral scholar Sarah St. Pierre said, “We take user selection out of the equation by allowing machine learning to examine the data and decide which model works best.”
"Constitutive Artificial Neural Networks could induce a paradigm shift in soft tissue modelling, from user-defined model selection to automated model discovery."
“Automating this process lowers barriers to model the brain. Now, every Stanford student can do this,” she continued. The hope is that brain trauma researchers can now more accurately simulate and comprehend precisely where brain trauma originates.
Consequently, Stanford University’s Constitutive Artificial Neural Networks model will inspire the next-generation design of protective equipment and treatments. As a next step, the laboratory will collaborate with the simulation software company, Dassault Systemès Simulia, to incorporate automated model discovery into their future analysis workflow.
According to Kuhl, “What’s really most exciting ... is that Constitutive Artificial Neural Networks could induce a paradigm shift in soft tissue modelling, from user-defined model selection to automated model discovery. This could forever change how we simulate materials and structures.”
Linka, K., St. Pierre, S. R., & Kuhl, E. (2023). Automated model discovery for human brain using Constitutive Artificial Neural Networks. Acta Biomaterialia. https://doi.org/10.1016/j.actbio.2023.01.055
Get your weekly dose of industry news and announcements here, and keep up to date with the latest ‘Industry Spotlight’ posts. For other PharmaTec content, please visit the PharmaTec Content Portal.
Want to find out more about the innovations happening in pharma data? Join Oxford Global’s annual Pharma Data & Digital Medicine event today. This 2-day conference brings together a panel of prominent leaders and scientists, sharing new case studies, innovative data, and exciting industry outlooks.