From Theory to Therapy: Accelerating Ligand-Based Virtual Screening with AI and ML Technologies
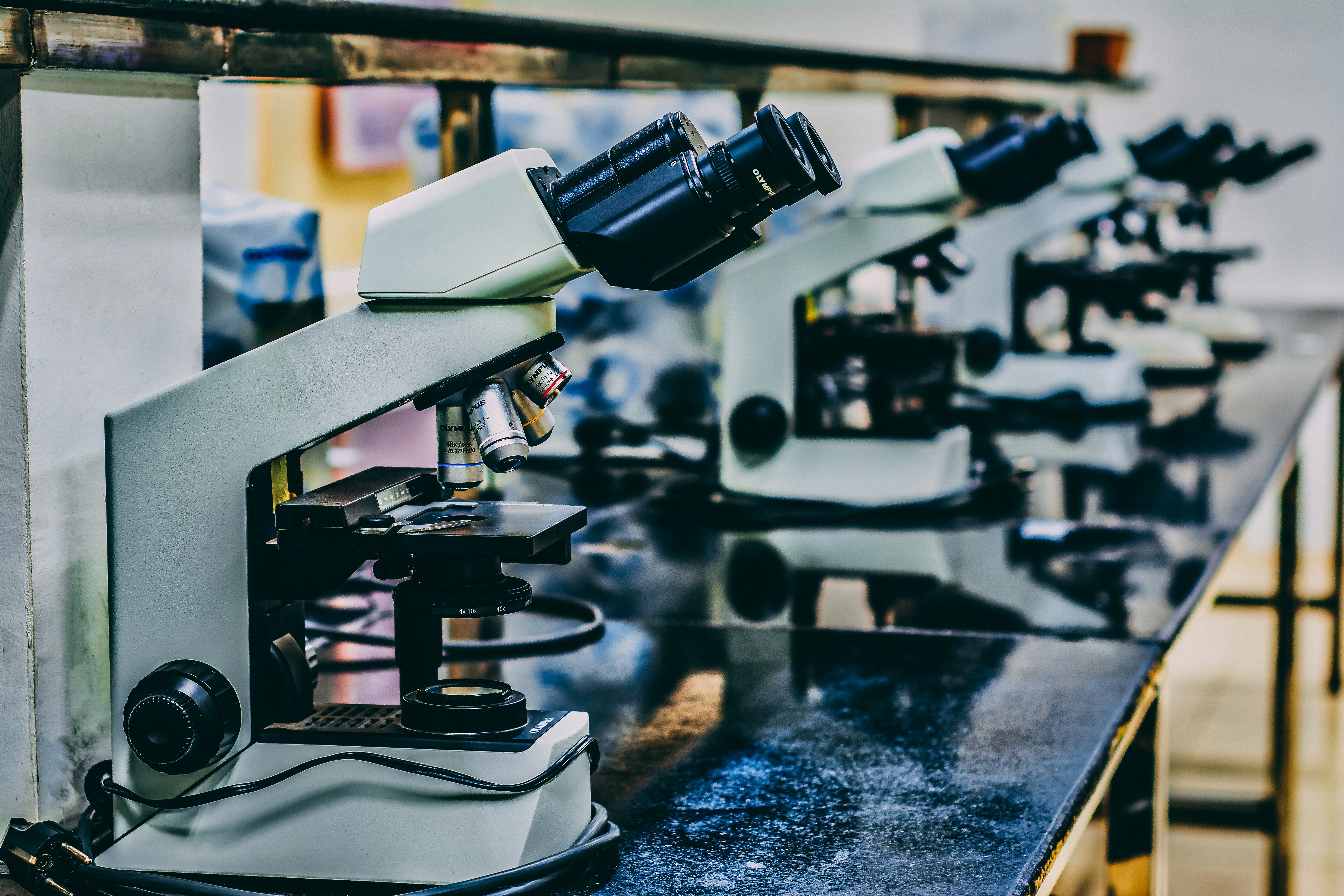
Ligand-based virtual screening (LBVS) represents a cornerstone in the drug discovery process, offering a computational approach to identifying novel compounds that may bind to specific biological targets. This methodology hinges on the principle that molecules similar in structure are likely to exhibit similar biological activities. Traditionally, LBVS has enabled researchers to sift through vast chemical spaces, pinpointing candidates for further experimental validation. However, the approach is not without its challenges, including the handling of vast datasets and the complexity of molecular interactions.
However, Artificial Intelligence (AI) and Machine Learning (ML) technologies have started to significantly impact various scientific fields, with drug discovery being no exception. AI and ML promise to transcend traditional LBVS limitations by harnessing computational power to process and learn from data in unprecedented ways. This month, Discovery By Oxford Global dives into the transformative role of AI and ML in ligand-based virtual screening, exploring how these technologies are not just enhancing but revolutionizing the process, making it more accurate, efficient, and innovative
Understanding Ligand-Based Virtual Screening
Ligand-based virtual screening is a method used in the early stages of drug discovery to identify molecules that could bind to a biological target of interest, typically a protein that plays a role in disease. Unlike structure-based methods that require a detailed 3D structure of the target, LBVS relies on information from known ligands—molecules already known to interact with the target.
The process involves comparing the chemical properties and structures of potential ligands against those of known active compounds. By employing various computational techniques, such as similarity searching, pharmacophore modeling, and machine learning algorithms, LBVS predicts the likelihood of a compound being active against a target.
Applications and Challenges
LBVS has been instrumental in identifying lead compounds in several therapeutic areas. However, the method faces significant challenges, including the need for large, high-quality datasets of ligands and the complexity of accurately modeling molecular interactions. The inherent limitations of traditional computational methods in handling the dynamic and multifaceted nature of biological systems further complicate the screening process.
Fundamentals of AI and ML in Drug Discovery
AI and ML stand out in drug discovery for their ability to learn from and make predictions based on data. These technologies can uncover patterns and insights from complex datasets much more efficiently than human researchers or traditional computational methods.
Types of ML Models in LBVS
- Supervised Learning:
These models are trained on labeled datasets, making them suitable for predicting the activity of new compounds based on prior knowledge. - Unsupervised Learning:
Useful in identifying novel patterns or clusters within data without predefined labels, unsupervised learning can help uncover unknown ligand-target relationships. - Reinforcement Learning:
This approach enables models to learn optimal actions through trial and error, offering potential in optimizing screening strategies and decision-making processes in drug design.
AI and ML Enhancements in LBVS
The integration of AI and ML into LBVS has led to notable improvements across various facets of the screening process:
- Feature Selection and Dimensionality Reduction
AI and ML excel in identifying the most relevant features of ligands that contribute to biological activity, streamlining the representation of compounds and enhancing the screening's accuracy.
- Improved Prediction Models
ML algorithms, especially deep learning, have demonstrated superior performance in predicting binding affinity and specificity, leveraging complex representations of molecular structures and interactions.
- Novel Ligand-Target Interactions
Deep learning models, through their ability to learn high-level features from data, have shown promise in identifying previously unknown ligand-target interactions, opening new avenues for drug discovery.
Case Studies: AI and ML in Action
The transformative impact of AI and ML on ligand-based virtual screening (LBVS) is best illustrated through specific case studies that showcase their potential in revolutionizing drug discovery.
- Breakthroughs in Identifying New Drug Candidates
One notable example is the use of deep learning models to identify potential inhibitors for the Ebola virus. Researchers utilized a convolutional neural network to screen over one million compounds, resulting in the discovery of new compounds that showed high binding affinity to the Ebola protein, which were later confirmed through laboratory tests. This success story underscores the efficiency and accuracy of AI in identifying viable drug candidates from vast chemical libraries. - Integration in LBVS Workflows
Another significant advancement is the integration of ML algorithms into existing LBVS workflows to enhance the prediction of drug-likeness and pharmacokinetic properties of molecules. For instance, a pharmaceutical company implemented an AI-driven platform to refine their screening process, resulting in a 50% reduction in time to identify lead compounds with optimal properties. This integration demonstrates how AI and ML can streamline drug discovery processes, making them more efficient and cost-effective.
Overcoming Challenges with AI and ML in LBVS
Despite their potential, the application of AI and ML in LBVS faces several challenges that researchers are actively working to overcome.
- Data Quality and Availability Issues
High-quality, annotated datasets are crucial for training accurate and reliable ML models. Efforts to address this include the development of public databases and the use of data augmentation techniques to enhance the diversity and quality of datasets available for training. - Computational Resource Constraints
The computational demands of AI and ML models, particularly deep learning algorithms, can be substantial. Cloud computing and specialized hardware, such as GPUs, are increasingly being utilized to meet these demands, enabling more complex models to be trained more efficiently. - Ethical Considerations and Bias Mitigation
Ensuring fairness and avoiding bias in AI-driven LBVS is paramount. Initiatives to address this involve the implementation of bias detection and mitigation strategies during model training, as well as the development of transparent AI systems that allow for the scrutiny of decision-making processes.
Future Directions and Potentials
The future of AI and ML in LBVS is bright, with emerging trends poised to further enhance the drug discovery landscape.
- Emerging Trends
Advancements in AI techniques, such as transfer learning and generative adversarial networks (GANs), hold promise for creating more accurate and generalizable models. These techniques can potentially reduce the need for extensive datasets and enable the discovery of entirely novel chemical entities.
- Impact on Drug Discovery
The continued integration of AI and ML is expected to significantly impact the speed, cost, and success rates of drug discovery. By enabling more precise predictions and efficient screenings, these technologies can reduce the time from initial screening to clinical trials, lower R&D costs, and increase the likelihood of discovering viable drug candidates.
Conclusion
The integration of AI and ML into ligand-based virtual screening represents a pivotal shift in drug discovery, offering unprecedented opportunities to enhance efficiency, accuracy, and innovation. Through specific case studies, we've seen the tangible benefits these technologies bring, from breakthroughs in identifying new drug candidates to streamlining existing workflows. While challenges remain, ongoing efforts to address data quality, computational resources, and ethical considerations are paving the way for a future where AI and ML play a central role in discovering the next generation of therapeutic agents. The importance of continued innovation in these technologies cannot be overstated, as they hold the key to advancing LBVS and revolutionizing the field of drug discovery.
Get your weekly dose of industry news here and keep up to date with the latest ‘Industry Spotlight’ posts. For other Discovery content, please visit the Discovery Content Portal.