CGT Manufacturing: Key Considerations for Implementing AI/ML Approaches
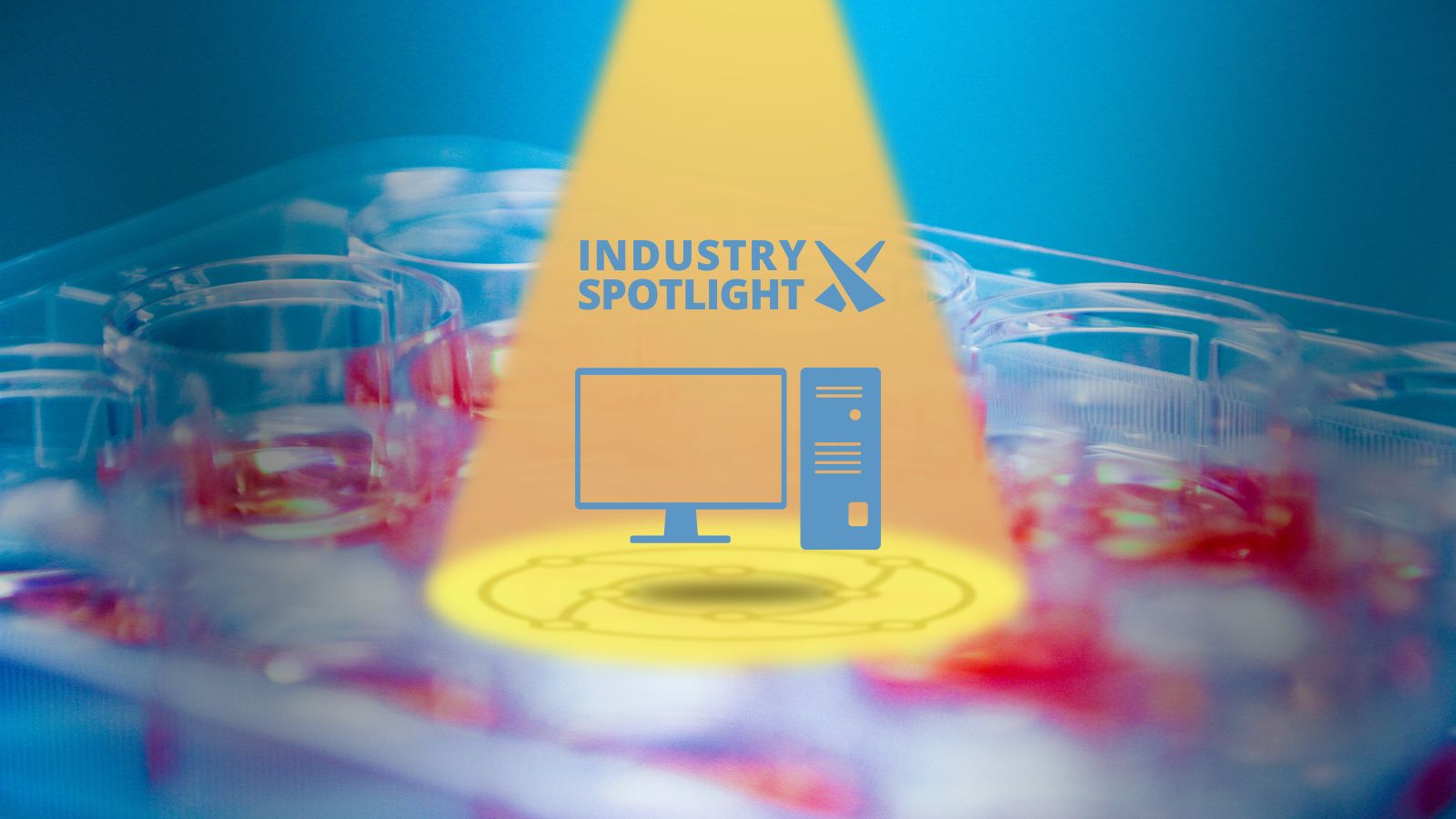
Some of the biggest obstacles in cell and gene therapy (CGT) manufacturing and development include scalability and the potential implementation of process optimisation.
AI (artificial intelligence) and ML (machine learning) approaches have the potential to accelerate several facets of the CGT development pipeline, including the identification of potential therapeutic candidates in the early stages of development.
Noise has also been made recently by the submission of developmental therapeutics to the FDA partially on the basis of phase I and II trials populated with real-world data as a means of streamlining the time taken in the clinical trial phase.
If implemented properly, AI and ML approaches have the potential to reduce the CGT developmental pipeline - which currently takes roughly a decade for a product to go from inception to final approval - by around a year.
However, augmenting CGT manufacturing with AI/ML approaches takes more than just substituting the more menial aspects of production with software.
Here, we explore three key considerations for the implementation of alternative approaches in CGT manufacture.
1. Reskilling Takes Time and Investment
A major hurdle to the greater implementation of AI/ML approaches in CGT manufacture is skill-based.
Implementing AI strategies in an environment not designed around them requires an overhaul of inbuilt procedure, along with the potential reskilling of researchers and staff with a background in biochemistry rather than data science.
AI approaches are introduced to streamline pipelines for data science, data working, and process development.
However, they can inversely slow development down if researchers have to spend time vetting data and getting it to a workable standard instead of being able to interpret it for the next stage of development.
2. Data Quality is Key
As the industry adage goes, ‘garbage in means garbage out'. In other words, a model is only as good as the data provided to it.
Machine learning specifically looks for historical patterns and then makes predictions based on that history.
In this context, the importance of reliable data cannot be overstated in the implementation of pipelines for data science.
The next generation of technologies promise to ease major bottlenecks in CGT production, not only by eliminating human errors but also through enabling process scalability and reliability.
3. Not All Processes Can Be Automated
As the CGT industry is highly competitive, manufacturing teams need to make a viable process with minimal business strategy during early development and then begin to rapidly scale.
While the prospecting phase of development is one that could be automated to a degree, some of the processes involved are also highly skilled and manual.
Large-scale CGT manufacturing approaches and potential solutions are tricky to automate, meaning there are no solutions which currently exist in the market.
Recent investments in the CGT field related to AI/ML in process development have centred on technologies to improve the reliability of manufacturing and throughput.
However, there is still significant potential for the acceleration of CGT manufacturing using AI.
Some examples of automation systems already present in CGT manufacture include bespoke devices for cell washing and virus purification.
While AI/ML approaches are not a ‘one-size fits all' solution for delays and holdups in CGT manufacturing, the proper interpretation of deep learning approaches and data interpretation may help to speed up the process.
Get your weekly dose of industry news and announcements here, or head over to our Cell portal to catch up with the latest advances in cellular therapies.