Advanced Antibody Engineering: AI, ML, and Computational Tools
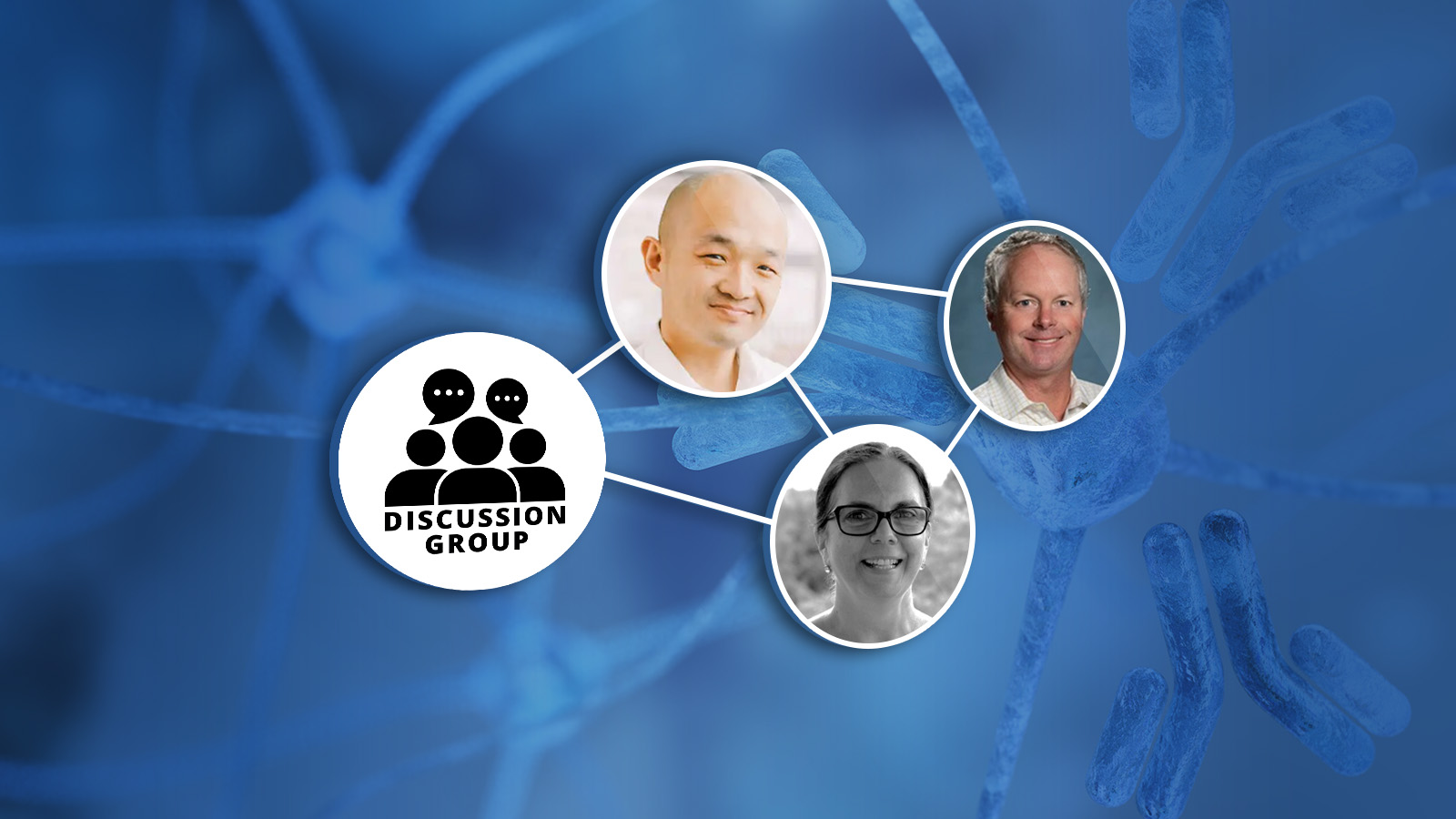
Our April group came together for an hour of specialist discussion about the current state and future directions of antibody engineering with advanced computational technologies. This Discussion Group was a select group of key industry leaders from various pharmaceutical and biotech companies. Notable attendees were senior representatives from GlaxoSmithKline, Pieris Pharmaceuticals, Lundbeck, and Zoetis, to name a few.
Philip M. Kim, Professor at The Donnelly Centre for Cellular and Biomolecular Research at the University of Toronto, led the discussion, whilst Slobodanka Dina Manceva, Senior Scientist at Teva Pharm and W. Blaine Stine, Senior Director, AbbVie, were there to support. Key discussion topics included best practices for implementing Machine Learning (ML) and Artificial Intelligence (AI) and computational tools to harness the development of next-generation antibody therapeutics.
Design of Epitope-Specific Antibodies with Machine Learning Methods:
Kim kicked off the session with a brief overview of the current industry strategies for computational antibody design. Entitled ‘Design of Epitope-Specific Antibodies with Machine Learning Methods,’ the presentation alluded to optimised therapeutic model design using ML. Kim opened with the following sentiment: “we live in an exciting time where data availability, computing resources, and novel artificial intelligence methods enable fully de novo design for biologics.”
Protein design as a field has come a long way since the 90s. The field relies heavily on three advances of the past decade: data, technology advances in hardware, and artificial intelligence. “We live in an era now where we depend increasingly on physics-inspired models being superseded by data-driven models based on AI and neural networks that capture integral relationships,” Kim continued.
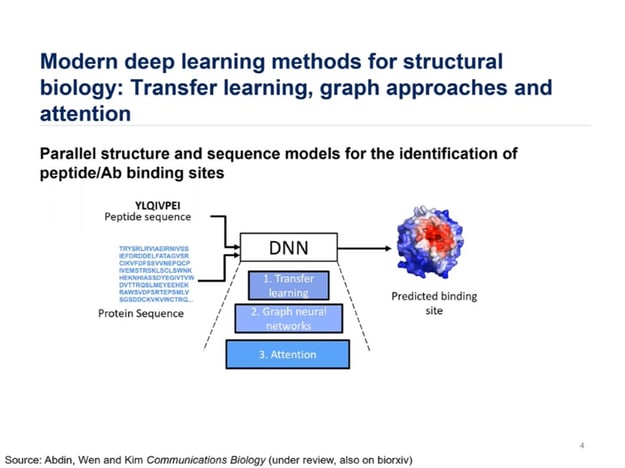
Figure. 1 – Deep Learning Methods for Identification of Peptide Binding Sites.
Advances in data availability can be channelled into the design of antibodies. Kim identified three integral components of the machinery employed by his lab to determine the parallel structure and sequence models for the identification of peptide binding sites (see figure. 1). Components include transfer learning, graph neural networks, and Attention.
According to Kim, “transfer learning can be used to compensation for peptide-protein data scarcity.” Transfer learning can be applied in two ways: pre-training the model on protein-fragment protein complexes and embedding protein sequences with contextualised language models. Speaking on the advantages of graph neural networks, Kim claimed that “graphs are a natural way to represent molecular structure.” They are both rotation and translation invariant and do not require discretisation.
“We live in an exciting time where data availability, computing resources, and novel artificial intelligence methods enable fully de novo design for biologics”
Finally, Attention modules are adapted from the graph input to identify long-range dependencies in sequence inputs. The Kim lab’s developed model is primarily composed of attention modules. Its three utilities include learning sequence context in the peptide, learning sequence and structural context in the protein, and learning the relationships between peptide and protein residues. As Kim puts it, “Attention is one of the most elegant ways to capture structural features.”
Application Findings: Model Architecture:
The three-component method is then used to initiate Reciprocal Attention, a novel AI feature that captures peptide-protein and protein-protein interactions (figure. 2). Reciprocal Attention allows information to flow in dual directions, from the peptide to the protein and back from the protein to the peptide.
Kim conceptualised the model workflow as consisting of “self-attention layers which allow the model to learn from sequence and structural context.” The Reciprocal Attention module then updates protein and peptide embedding while enforcing symmetry. The final layers project model then embeds down to residue-wise predications.
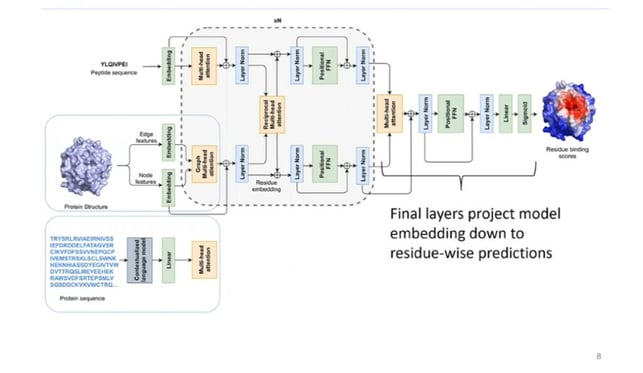
Figure. 2 – Example Peptide Network.
Applications for AI in Antibody Development:
After Kim’s presentation concluded, Stine opened the floor to discuss the biggest opportunities for computational AI and ML approaches for discovering new therapeutic biologics. He asked, “what are the most practical applications right now for problem-solving for these emerging methods today? And what can we expect to see on the horizon?” Kim identified the most effective application as being library design for language models. Audience members agreed, with some attesting to its maturation and evident commodification.
Pipeline applications were identified as de novo antibody design in their potential to open a new target space within the field. De novo design refers to the computational procedures used to yield the antibody binding sequence from a target epitope. Kim remarked that one of the most compelling aspects of de novo design is its relative ease of application.
- Could Targetting Complex Membrane Proteins be an Understudied Opportunity in Antibody Engineering?
- Discover More About Optimising Receptor Trafficking with Bispecific Antibody-Drug Conjugates
- What’s Next for Antibody-Based Therapeutic Discovery and Optimisation?
One audience member queried whether “de novo design is still possible in instances of less well-defined loop structures?” The panel agreed that whilst conceptually it is possible, statistical evidence remains insufficient. Possible workarounds include targeting a set of main structural clusters and relying upon a single selection.
Data Management for Computational Biologics Design:
A further audience member inquired about available applications to manage data output. The said party explained their predicament as follows: “when we apply a similar next-generation sequencing approach, we usually end up with a lot of data, but there is a limit to what we can actually go and test in our lab”. Whilst Manceva indicated the value of large data availability, she acknowledged that showing proof of concept early on creates a streamlined process which can help to reduce data volume created during extensive testing.
Kim remarked that one of the most compelling aspects of de novo design is its relative ease of application.
Stine further suggested the potential value of “pushing computational methods to acquire smaller sets of antibodies and by extension guarantee production and characterisation”. Additionally, using small-scale methods could facilitate rapid iteration and data generation. This, in turn, provides a method of learning, allowing the model to automatically retrain and redesign accordingly.
The discussion concluded with some final thoughts on the future of AI and ML design for antibody engineering. With the promise of alpha fold technology and an ever-expanding array of biotech start-ups entering the arena, the field of antibody-based therapeutics is certainly catching momentum. At Oxford Global, we couldn’t have been more pleased with the turnout for our April biologics Discussion Group. The conversation was engaging, the debate stimulating, and the industry insights invaluable. We will continue our Discussion Group series in May with a session focusing on ‘Oral Delivery of Peptides’. Learn more about the Oxford Global Discussion Group series at our Biologics Portal.
Want to find out more about the latest market news and industry insights? Join our Oligonucleotide Chemistry & Therapeutics Symposium and discover the latest in oligonucleotides chemistry, process and analytical development, therapeutics, and antisense therapy.