Transforming Genomics with AI
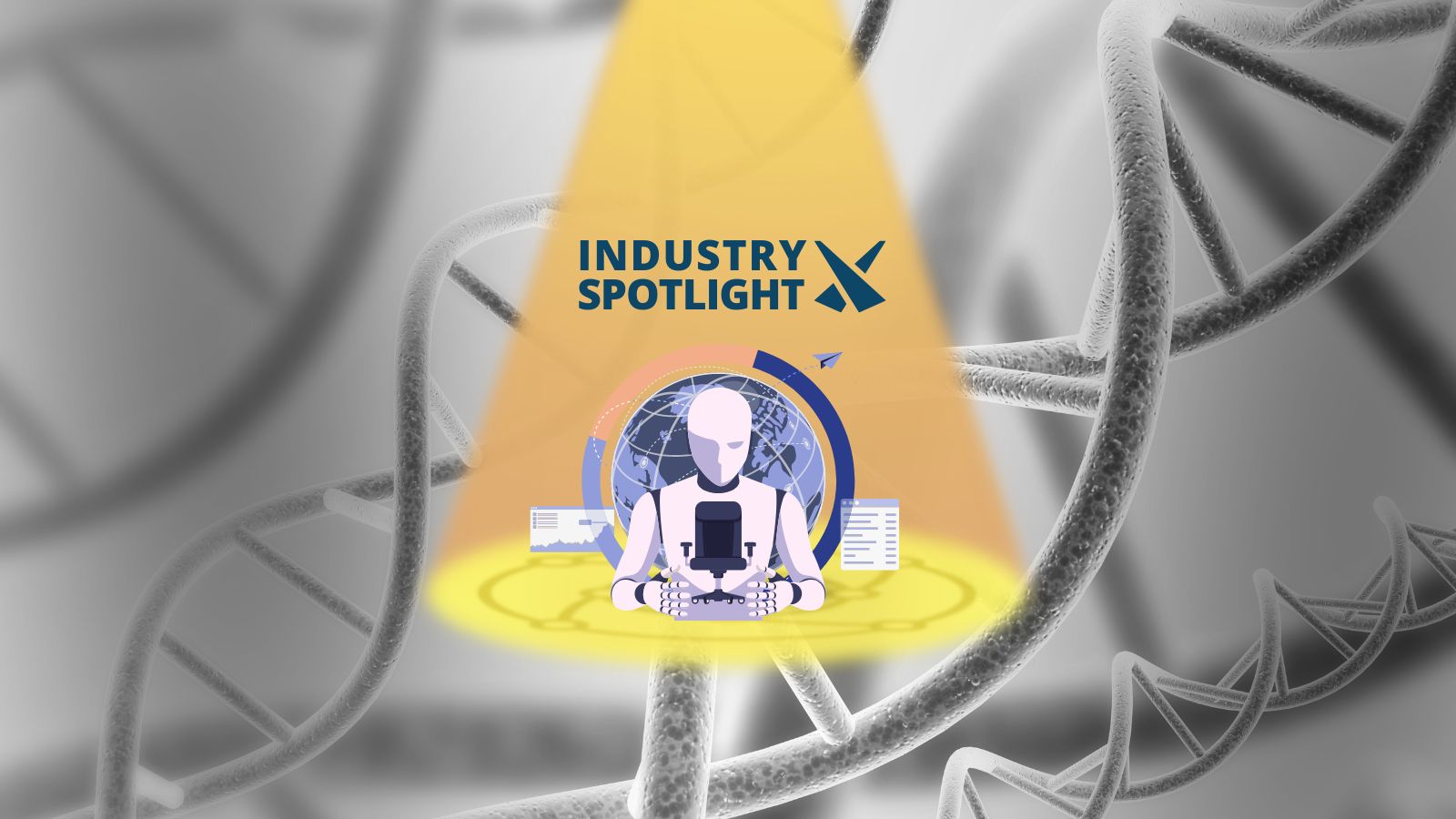
A digital revolution is underway in whole genome sequencing. Across the world, genomic programs are gaining momentum since the recent decline in costly high-throughput and next-generation sequencing.
Instead, a transition in the widespread adoption of a low-cost computerised approach to data analysis is taking place. This embrace of accelerated data science and AI for analysing genome sequencing has facilitated an increase in the throughput and read accuracy of the human genome.
Genome analysis generates approximately 100 gigabytes of raw data. This figure goes on to double following sequencing with complex algorithms and deep learning processing applications. As a result, there is a growing need for accelerated workflows to accommodate this explosion in bioinformatics data.
Deep learning is one way to achieve this. In particular, deep learning offers the ability to interpret the image and signal data of neural networks and analyse up to 3 billion nucleotide pairs of the human genome.
- Novel Genome Editing Techniques: What Will Come Next after CRISPR-Cas9?
- Machine Learning Algorithm Measures Prime Editing Efficiency
- Video Interview: The Future Landscape of Genome Editing For Disease Models
Such a technique improves read accuracy and guarantees that base calling happens closer to real-time, advancing the genomics workflow. Alignment technologies such as BWA-MEM further assist genomic analysis by enabling the rapid mapping of DNA sequences reads to a reference genome.
Other alignment algorithms include starfusion (or STAR), which uses RNA-sequencing data to deliver fast and accurate alignment to understand gene expression. In addition to this, the Smith-Waterman algorithm provides a promising and vigorous programming accelerator.
This embrace of accelerated data science and AI for analysing genome sequencing has facilitated an increase in the throughput and read accuracy of the human genome.
When it comes to variant calling, arguably one of the most vital stages of sequencing, identifying differences between the patient sample and reference genome requires optimised callers. Callers increase the speed of analysis and help researchers determine genetic diseases and new drug targets based on variants such as small insertions and deletions, single-nucleotide changes, or complex rearrangements.
Among the most promising callers technologies include the Broad Institute’s GATK, which helps remove false positives, Google’s DeepVariant, and the NVIDIA Clara Parabricks suite of tools which reduces the runtimes from 16 hours to less than 5 minutes.
Forecasting the next wave of genomics is an exciting prospect. When predicting the next big thing in whole genome sequencing, it can be argued that the future is already here. As the industry edges towards a fully AI and computer-based approach, it is safe to say the boundaries of whole genome sequencing are already being pushed.
Get your weekly dose of industry news and announcements here, and keep up to date with the latest ‘Industry Spotlight’ posts.
Visit our Omics Portal to learn more about the latest research into gene editing and its latest developments. If you’d like to register your interest for Oxford Global‘s upcoming Spatial Biology UK: In-Person event, click here.