The Dawn and Future of Spatial Transcriptomics
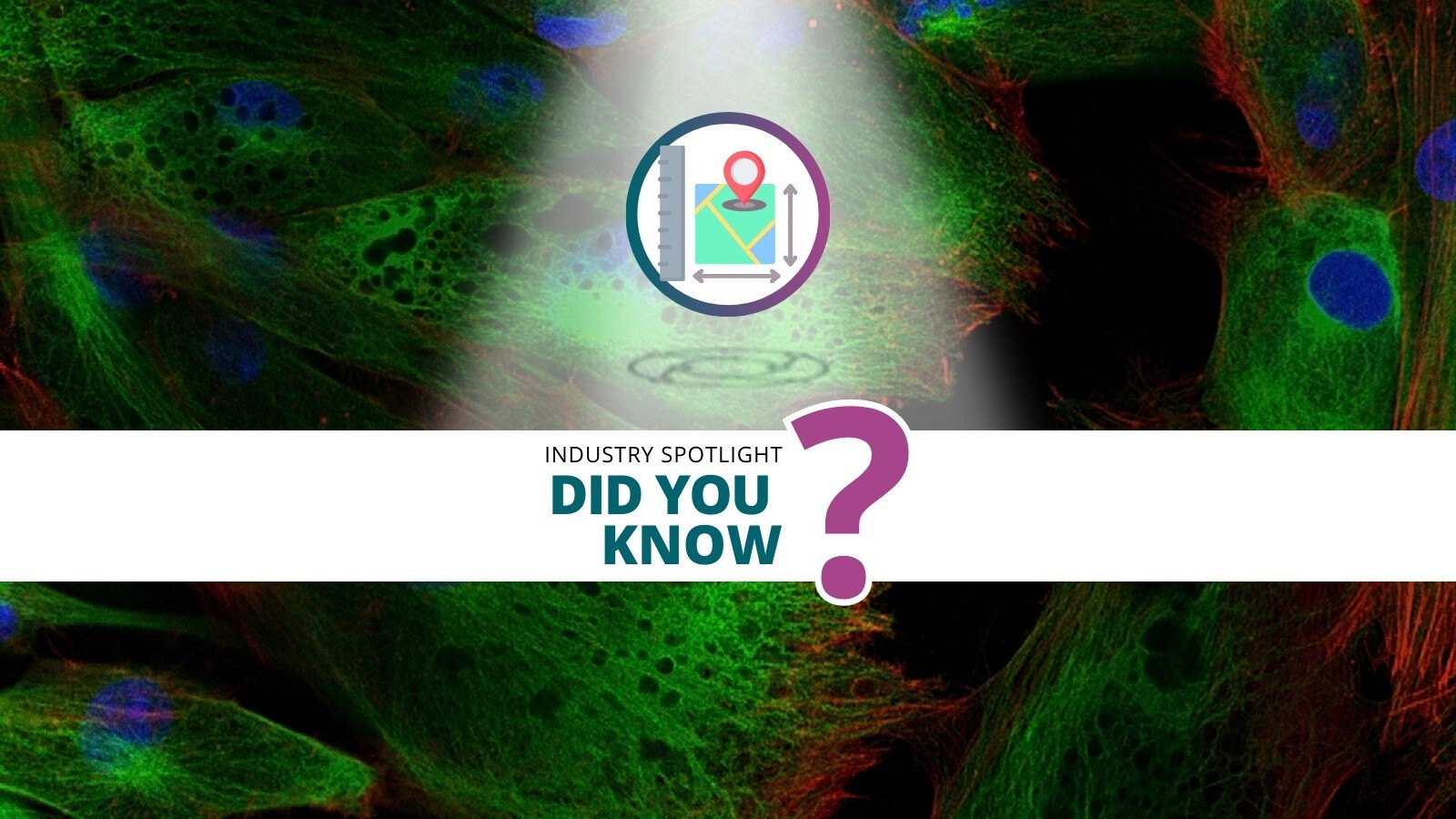
Nature Methods' method of the year in 2020 was spatial transcriptomics, an advanced technique that enables researchers to measure and map gene expression within the spatial context of a tissue sample.
The paper that kicked off the spatial craze was authored by Patrik L. Ståhl et al. and published in Science in 2016. Before this paper, analysis of gene expression in tissues was limited to RNA-sequencing of a few proteins at a time, and was homogenised, meaning the data lacked spatial context.
However, including the positional context of transcripts is important to obtaining a fuller understanding of biopsies. Ståhl et al coins the term 'spatial transcriptomics' for the technique that it introduces. The pioneering technique visualises sections of a tissue biopsy with full spatial resolution
"By positioning histological sections on arrayed reverse transcription primers with unique positional barcodes, we demonstrate high-quality RNA-sequencing data with maintained two-dimensional positional information from the mouse brain and human breast cancer."
Being able to see where genes are expressed within a tissue allows researchers to perform novel bioinformatic analysis with the goal of improving diagnostics and drug discovery.
The technology was eventually bought by 10x Genomics and commercialised under the product name 'Visium'.
Methods for spatial transcriptomics have since been refined and diverged from the original paper in some ways, but here's how the method generally works now:
First, the tissue sample is prepared and preserved using methods that maintain its structural integrity, like freezing or formalin-fixation and paraffin-embedding (FFPE). The sample is cut into thin sections using a microtome and then mounted onto a slide which is pre-coated with oligonucleotide barcodes which indicate the spatial position on the slide.
Next, permeabilization allows mRNA to diffuse out of the samples' cells which are captured by the barcoded oligonucleotides. Then, researchers can reverse-transcribe the captured mRNA into complementary DNA (cDNA) on the slide. As the cDNA preserves the oligo barcode, when the cDNA is collected, researchers can decode its locational information when it is sequenced.
Finally, the cDNA is amplified in preparation for its sequencing. High-throughput sequencing is used to identify and quantify the gene expression levels. Combining that sequencing data with information from the spatial barcodes then allows the researchers to construct a spatial gene expression map including patterns within the tissue.
One direction that the field of spatial transcriptomics has taken has been to pair the technology with digital pathology and image analysis techniques. Computational tools, notably machine learning (ML) techniques, have been used to analyse spatial gene expression data which provides insights into the spatial organization of biological processes and cell types within a sample.