Spatial Omics Technologies: Spatial Proteomics, Mass Spectrometry, and Imaging Data Uses
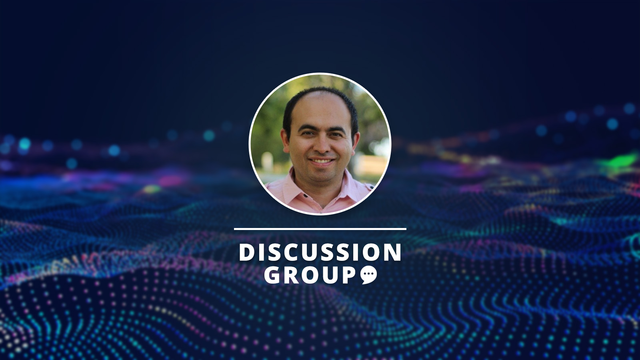
Oxford Global’s October 2022 Discussion Group in the NextGen Omics series focused on the applications of spatial proteomics in image analysis and the sequencing of the tumour microenvironment through spatial omics technologies. The discussion was led by Ahmet Coskun, Bernie-Marcus Early-Career Professor at the Georgia Institute of Technology. A major focus of the session centred on image analysis, along with the fundamentals of cellular behaviour and cell-to-cell interactions. Deep learning applications in the monitoring of the tumour microenvironment (TME) featured as a topic which held promise for future analysis.
“There are so many steps involved in RNA-seq, and other imaging approaches,” opened Coskun. “It brings us to a point where we need to be very proficient at image processing and understand and register these images with an accuracy that is acceptable.” He continued that many results in his field are based on antibody quality, and that this was a key factor in spatial proteomics. “There are suggestions in the literature that multiple epitopes in the same basic cell can be compared and cross-validated.”
Applications of Mass Spectrometry and Imaging Data Uses
Coskun began by giving an overview of his research background and recent areas of focus. “My lab has been investigating tumour microenvironment using spatial technologies,” he explained. “We are looking at spatial proteomics datasets where different colours correspond to individual immune cells.” He highlighted a prostate cancer sample, where certain colours corresponded to a particular cell phenotype, and explained that in other scenarios machine learning can be used to highlight the subsidiary distribution of molecules in enriched sections of tissue.
Coskun explained that this approach stemmed from the rationale that antibodies can be conjugated to isotopes as well as fluorophores. “With a tumour microarray sample, we use the UV laser to generate a signal that we can then read through mass spectrometry,” he said. This signal can be interpreted to detect biomarkers for cancer and tumour region distribution. “We are also looking at T cells and their diversity,” Coskun added, “and their diversity from stem-like T cells all the way to cytotoxic and effector T cells.” By processing multiplex data, cancer stroma, macrophages, and T cell markers can all be viewed in the same dataset, abetting understanding of the TME through the application of spatial omics technologies.
- Discussion Group Report: Antibody-Mediated Rejection and Next-Gen Sequencing in Precision Medicine
- Commentary: Utilising Single-Cell Metabolomics to Reveal Metabolic Cell States
- Insight Article: Current Bioinformatics Trends in Single-Cell Data Analysis
“We are getting to the point now where we are looking at sub-cellular populations of proteins,” continued Coskun. “We are studying signalling factors using multiplex data, looking at cell nuclei.” The regulation of signalling within the cytoplasm can be studied; signalling may be spatially distributed within different subcompartments of the cells. Multiplex data was coloured and grouped by clustering methods, with differences in single-cell signalling patterns observed in the same cellular subpopulation. “We can also do this in three dimensions,” he said. Discussing future aims, Coskun added that the next step would be to combine different imaging datasets to incorporate all aspects of the protein.
Sequencing Information and Advances in Image Data Storage
One question from the audience concerned different layers of interplay in protein complexing. “I’m wondering if there’s another layer you can build in here,” she said. “If you look at tumour slides, you will have an interplay of different cellular types. Would this spatial information give you different dynamics of protein interplay between a tumour cell versus another type of cell?” Coskun replied that a big focus in his research around spatial proteomics at present was looking at multiple cell features.
“When we are conducting this analysis, we are looking at not just cell type. We are also looking at CODEX 3D subsidiary and similar analysis, using super-resolution microscopy and reconstructing this in 3D to learn what is going on in the tumour microenvironment.” Coskun gave the example of studying the organisation of features within a tumour with respect to neighbouring cells. Regarding the upper limit of analysis for markers, Coskun said that the feasibility was there for roughly 100. He added that in the case of cancers with a very small tissue size limitations could occur regarding sample integrity, and that this was a current challenge for US commercial technologies working based on bleaching technologies.
Another question came from Jens Brodbeck, Director at Gilead Sciences, concerning the impact of dataset size and field of view on analysis. Coskun explained that the high-resolution experiment in question enabled the generation of an image of 100 nm at super-resolution. “We are only looking at very highly-resolved data images,” he explained. “We use these networks for large storage — maybe a couple of hundred gigabytes — and then you combine them for a multiplex sample.”
Applying Deep Learning Techniques to Spatial 3D Modelling
Increasingly, artificial intelligence and deep learning can be integrated into spatial analysis to accelerate the process of staining regarding segmentation accuracy. Coskun moved on to an exploration of next-generation spatial 3D modelling techniques such as spatial transcriptomics. “Microscopes — by design — have the capability to go across the sample. If it’s just white field, you could go up to a couple of hundred micrometres with a decent quality separation — if it’s spinning disk confocal microscope you could go up to about a millimetre or so.” He explained that in the case of fixed samples, lattice light sheet microscopes — modified imaging tools which increase the speed of image acquisition while decreasing cellular damage from phototoxicity — provide a useful means of quickly investigating samples in 3D.
Different platforms for analysis result in different impacts on tissue integrity. “In terms of bioinformatics and data management, I think we should benefit from the sequencing community and structural biology,” said Coskun. One panel member agreed that there were a multitude of sequencing approaches available regarding spatial omics technologies. “There’s been an explosion in technologies around validation, and there was a lot of work in getting each functioning as they got more complex.”
“We’re zooming in on the T cell receptors,” explained Coskun. “If we were to use high-resolution imaging platforms, we would be looking at the receptor distributions.” Increasingly, new technologies facilitate the assembly of 3D spatial proteomics structures with 3D images from H&E staining. “I think this is what we are going to see in the community – there is a nice initiative at the moment where companies are starting to upload antibody-based articles.” By adopting consistent data practices across the community, the hope is that consortiums will be easier to put together as each individual laboratory adopts this practice.
Spatial Omics Technologies and Concluding Remarks
“You’ve beautifully shown that we can generate datasets of higher and higher complexity,” said Brodbeck of Coskun’s research. “Considering tumour heterogeneity, would we not benefit from looking at more examples with less complex approaches? How do you transform the complex data acquisition on a relatively low sample number into a scalable screening funnel to pursue a scientific hypothesis?”
“With the in situ sequencing methods, you only have to add a couple of extra steps to add a huge extra capacity for unique targets.”
“You’ve described one angle where you want to get as many markers as you physically can to help refine your question,” said one audience member. “The opposite could be true where you have a patient dataset with differences between patients.” He described a dual pipeline with a focus on the unbiased end. “Once you’ve qualified this, you can go straight to the middle — rather than looking at depth or breadth, you can look at different aspects of the study.”
The audience member explained that increasing the number of cycles and fluorophores involved in a single cell subset was important when aiming for higher perplexity. “With the in situ sequencing methods, you only have to add a couple of extra steps to add a huge extra capacity for unique targets, in terms of tissue quality but also assay time. I think that’s got a lot of strength for reaching those higher levels.”
Coskun agreed, adding that RNA-seq showed promise in conjunction with barcoding approaches. Wrapping up, he thanked the attendees for taking part in the discussion and said he hoped to see them for a future event concerning spatial omics technologies soon. “Please enjoy doing spatial proteomics and the study of cellular interactions in your core and individual labs.”
Want to take part in one of our future Omics discussion groups? Check out our community membership options to gain access to all of our past and present groups, as well as key insights and events in the imaging and spatial analysis field. If you’d like to register your interest in our upcoming NextGen Omics UK: In-Person event, click here to download the agenda and learn more.