Spatial Data: Computational Methods and Deep Learning-Based Models
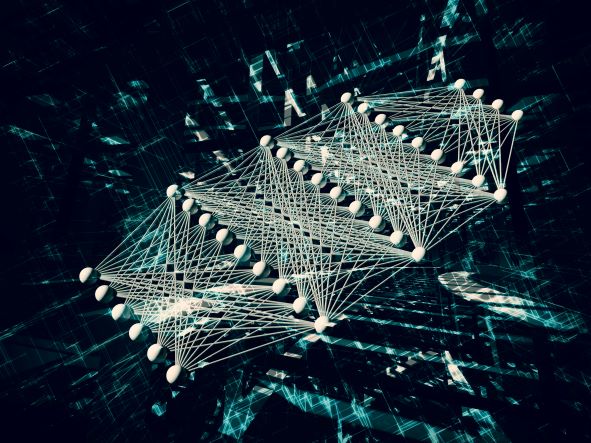
Presented by: Shirin Elizabeth Khorsandi, Senior Lecturer at King's College London
Edited by: Ben Norris
The importance of transparent data use and handling in healthcare has been emphasised by its utility in responding to the Covid-19 pandemic. Looking to the future, data from the spatial mapping of cancer types could be used to coordinate new healthcare responses. For Shirin Elizabeth Khorsandi, Senior Lecturer at King’s College London, the excitement centred around healthcare data and provision should be treated with a degree of caution, but the potential is huge.
Working With Data: Creating Structure from Noise
Shirin Elizabeth Khorsandi introduced herself as an AI enthusiast who saw a lot of potential in the new data currently emerging from healthcare provision. “There’s been an explosion in the different techniques for spatial over the past few years,” she told the audience at Oxford Global’s Spatial UK: In Person event in March 2022. “What’s happening at the moment is you’re getting different layers of data from different cancer types.” She explained that this represented a massive amount of spatial data which will inevitably cause issues for the future, particularly regarding computational cost and storage.
“The problem with a lot of the data which is around at the moment is it comes in a lot of different formats,” continued Khorsandi. “Structured, unstructured, semi-structured… it creates issues with concepts regarding normalisation, how you deal with noise, and the joys of missing data.” She highlighted the importance of being able to access repositories and atlases – datasets which can be utilised to experiment in silico following data collection.
Concerning cell data, there are different algorithms which can compute both cell data and manifold approximations of that data. “How you apply those dimensional reductions depends on how you handle the big data sets,” Khorsandi added. “Then comes the uncertainty – are you looking at something that is biologically important, or is that biologically important stuff missing?”
AI Development and Spatial Data
Having established some of the operational parameters she considers during her work, Khorsandi moved on to discussing forms of artificial intelligence. Increasingly, researchers and computer scientists are developing algorithms which are specifically developed for spatial data, “The algorithm becomes more complicated, with layers with different representations of data,” added Khorsandi.
“From data, you have your input of training data, you decide what features you’re going to select,” Khorsandi continued. “You prepare the data, you reapply the data, you apply the algorithm, and you get your output or prediction depending on what you’re trying to predict.” From here, research becomes a question of validating how well the machine learning model is working, with different layers of AI applicable depending on how complex the algorithm is. Khorsandi explained that the algorithm becomes more complex with progression into convoluted networks.
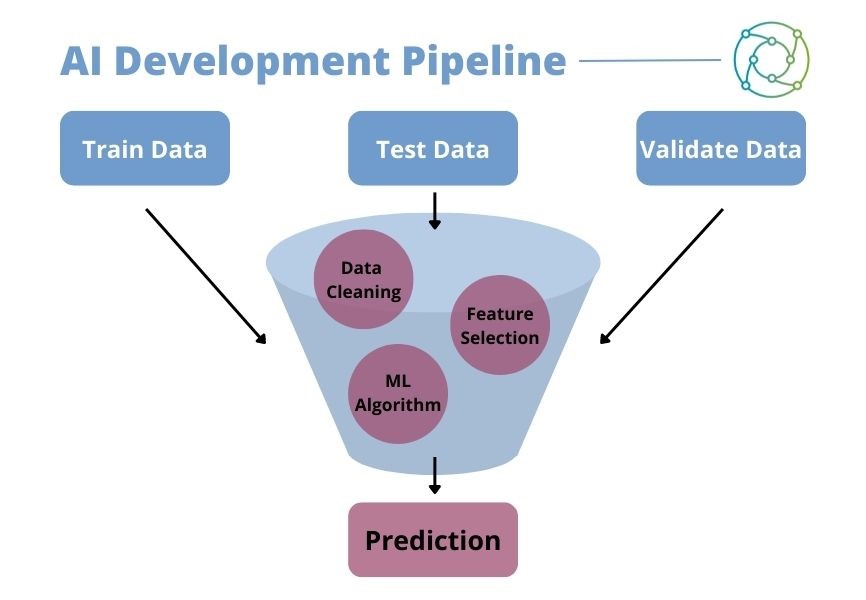
Deep learning models are a key avenue for the investigation and refinement of knowledge delivery. “You can define the nodes within layers depending on how you want to define your modelling within computational space,” said Khorsandi. “Ultimately, which algorithm you use will depend on what your problem is and the data you’re dealing with.” With more experimental science, there tends to be a skew towards deep learning.
Cluster Computing, High-Performance Computing and Digital Twins
“A lot of the boom in AI at the moment is a combination of new datasets which we haven’t had before, and these algorithms which are now available,” Khorsandi said. “Also important is the way we’ve now got computing power to allow us to do this kind of deep learning analysis.”
Khorsandi briefly touched on digital twins – virtual representations of real-world objects and datasets which are updated from real-time data. “I’m a bit of a futurist,” she said. “I do think as we understand these big datasets more, we can create a digital twin in computational space.” This would enable the performance of real-time analytics to test an intervention in silico to see what will happen when given parameters are altered. “Maybe that will be a way of preventing disease in future.”
“Put your toe in and have a play – you can’t break a computer.”
As a note of caution, Khorsandi warned other researchers to be aware of the algorithm they were using and the data it was trained on. “I think there have been some errors from over-trusting AI, and I think one of the important ones is bias. You’ve got to be clear what your endpoint is.” She stressed that studies should be clear on what they were trying to ask from their data, but also urged her peers to experiment. “Put your toe in and have a play. You can’t break a computer.”
Trusted Research Environments in Healthcare Data
Rounding off, Khorsandi emphasised the importance of involving the public to increase tissue access and its association with clinical data. “I think the main thing is publicising it to patients,” she said. “A lot of what I’ve seen from the clinical area is that clinicians and nursing staff are too busy. Conducting proper consent takes time and everyone regards it as an additional chore in the clinical pathway.” Properly executing this in the right way would be a stepping stone towards a higher level of data integration.
- Watch: Spatial Multiomics Symposium
- Spatial Analysis of the TME: Key Perspectives and New Areas of Focus
- Spatial Transcriptomics: Novel Tools and Innovations
“Part of the future of the NHS is a big transformation towards digitisation,” concluded Khorsandi. “They create these trusted research environments where healthcare data is going to be stored and accessed by researchers within the healthcare system.” She pointed to recent events which had reinforced the utility of healthcare data sharing. “I think Covid-19 highlighted how if you engage with patients, everyone’s on the same page with how translational research can help deal with a problem.”
Want to read more about the latest advances in genomic data handling and analysis? Head over to our Omics portal for further insights from the industry’s best and brightest. If you’d like to register your interest in our upcoming Spatial Biology: US conference, visit our event website.