Research Spotlight: Multi-Omics Data Harmonization for SARS-CoV-2 Virus and Drug Target Identification
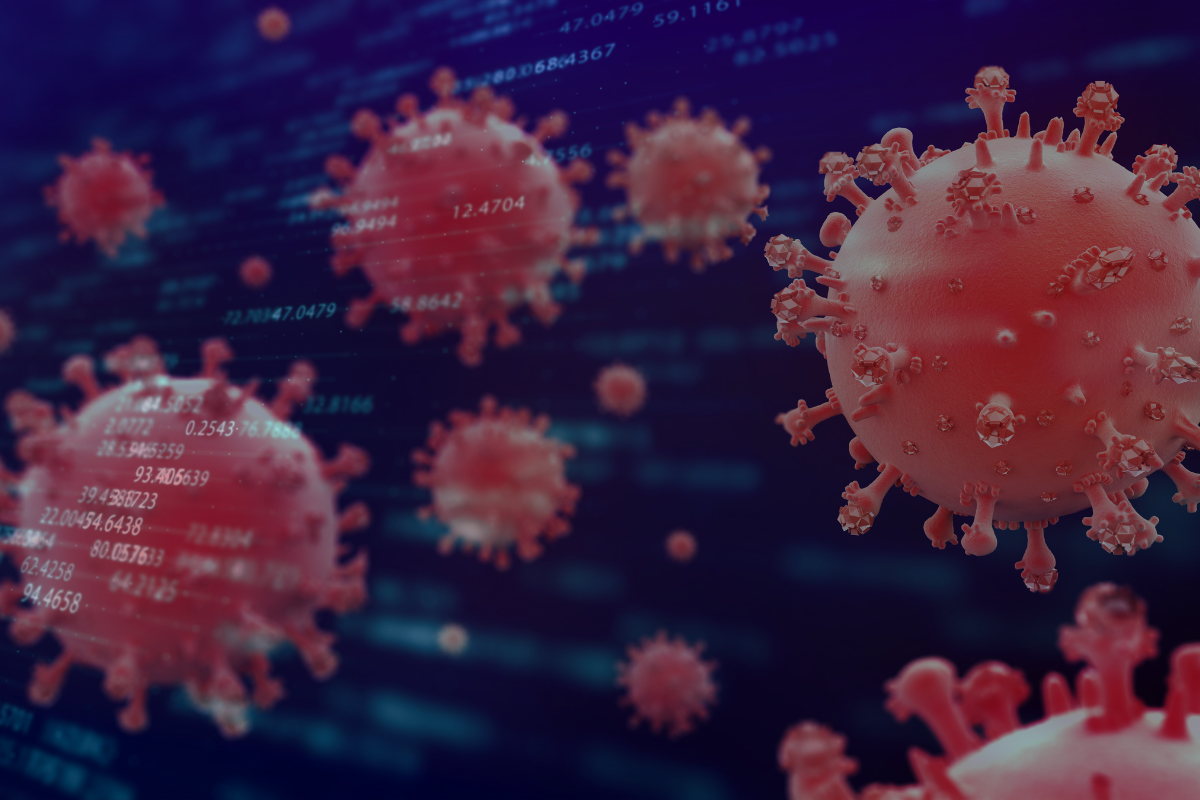
A team of researchers from Monash University in Melbourne, Australia have designed a multi-modal data harmonization pipeline to assist in drug screening. They have used the platform in a case study for the discovery of COVID-19 drug targets.
Since the beginning of the COVID-19 pandemic, a great deal of research has been centred on understanding the complex biology of the SARS-CoV-2 virus. Furthermore, this resulted in a large amount of epidemiological and molecular data about the structure and spread of the virus.
One of the most promising methodologies to do so has been using an aggregation of different functional omics data (rather than a single type of omics data) to interpret the molecular mechanisms of the virus.
RELATED:
- Spatial and Single Cell Transcriptomics Uncovers Mechanisms for Ischemic Stroke Recovery
- Post-Event Proceedings – NextGen Omics US 2023
- Spatial Transcriptomics Technologies: Current Trends and Analysis
The paper, published in Briefings in Bioinformatics, comments that the large amount of analysis within these studies only considers these data from "a narrow perspective, generating a single, specific category (or modality) of data corresponding to a single omics type."
Therefore, it is the view of the Melbourne team to favour separate analysis of processed data on each 'omics level' rather than integrating the data simultaneously and in parallel. It suggests that integrating the data in parallel may mask valuable information and therefore, the biomarkers that result may fall short of providing an accurate understanding of how the disease came about.
Biological systems are complex and heterogeneous, it is the paper's view that single-omics analysis of these data are not enough to get a clear picture of those systems.
It says: "Complex traits and diseases such as COVID-19 are often a result of composite interplay between the genome, environment and multiple layers of functional genomics, for example the lipidome, metabolome, proteome and transcriptome."
Rather, the paper highlights that a high-throughput, multi-omic approach is needed to understand the multiple layers of a biological system. Herein, the researchers consider integrating multiple omics datasets in what they term ‘multi-modal harmonisation.’ Their paper it presents a "generic, reproducible and flexible open-access data harmonisation framework that can be scaled out to future multi-omics analysis to study a phenotype in a holistic manner."
"Predicted advantages include greater data resolution, reduced noise and the ability to answer questions that a single data modality cannot, as demonstrated by existing studies. Furthermore, the user will also have a higher degree of confidence in the results due to their concordance on separate data categories."
The researchers demonstrated the pipeline using a drug screening case study. They combined different types of multi-omics data and looked for the lowest level of statistical associations between these data features in two specific scenarios. They were essentially trying to identify subtle relationships between different biological components in those situations.
They used the features with the strongest correlations for drug-target analysis which yielded 84 drug target candidates. Further analysis (computational docking and toxicity analysis) then returned seven 'high-confidence' targets which the paper thinks could be starting points for drug development. They were: amsacrine, bosutinib, ceritinib, crizotinib, nintedanib, and sunitinib.
Full citation:
Chen, T., Philip, M., Lê Cao, K. A., & Tyagi, S. (2021). A multi-modal data harmonisation approach for discovery of COVID-19 drug targets. Briefings in bioinformatics, 22(6), bbab185. https://doi.org/10.1093/bib/bbab185