Paving the Road to Multi Omics Bioinformatics
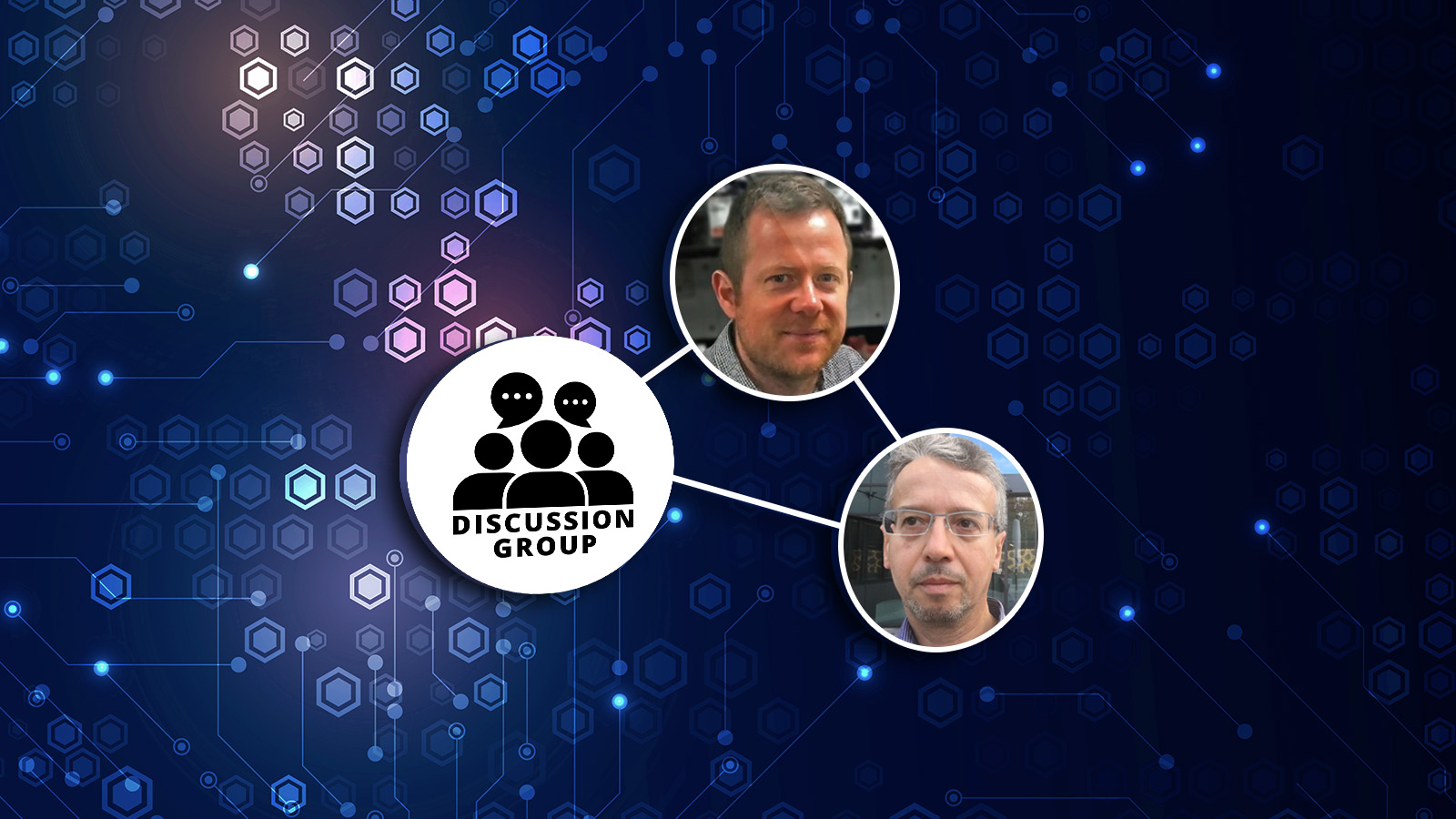
Our May Omics Series discussion group focused on the current uses and potential of multi-omics bioinformatics. Oxford Global’s discussion groups bring together a select group of 15-20 key industry leaders for approximately one hour for in depth knowledge sharing and conversation.
Taking the lead on this discussion group were Hywel Williams (Senior Lecturer in Bioinformatics, Cardiff University) and Raffaele Calogero (Professor, University of Torino).
Single “omics” technologies provide a view of the molecules that makes up the cell, tissue and organism. However, the view is usually limited only to single area such as genomic, transcriptomic, proteomic, and metabolomic levels. Integrating these technologies, including single cell level to generate a global view is often referred to as multi-omics approach.
Challenges
The number of variables in multi-omics studies can be enormous, creating additional obstacles compared to more conventional approaches. Omics data also often requires additional processes to infer missing values.
Software Limitations
The lack of standards and commercial software for multi omics data creates issues to verify results. One attendee explained that “utilising sometimes unique, newly developed software makes it almost impossible to really compare across datasets and to get valid comparisons.”
Calogero shared that his team try to use “software that's been published and gone through peer review. But once that software is out, and it's in the world for people to use, what happens when that software gets updated?” The issue with software updates is that any additions or changes will not have gone through the peer review process. He continues by asking “can we be sure that the additional attributes that are that are added to the software are actually valid?”
Williams also added the point that “most of us are not buying software; we're getting it free. The vast majority of this software is open sourced and accessed through things like GitHub.” While open-sourced software is more transparent and reduces costs, many organisations will create additional forks to suit their needs. With multiple versions of software available this can obfuscate how results were generated and make it more difficult to perform comparative analyses.
Hardware Limitations
Standard consumer and commercial laptops and desktops lack the power to process the large volumes of complex data needed for efficient multi-omics bioinformatics. Williams explains that “It’s a challenge for people to be able to access the compute required to run multi omics analysis. You're going to need a high-powered high-performance computing. It is very unlikely that you will be able to do multi omics analysis on a standard PC laptop. You have a choice between using your own server clusters or using cloud-based systems.” The high cost of buying or leasing the required computing power presents a barrier to entry.
Williams notes that “a number of sites are starting to move to Amazon Web Services. That seems to be a popular way to access compute power without the infrastructural burden of having to buy your own cluster and keep it running and updated five years or so.”
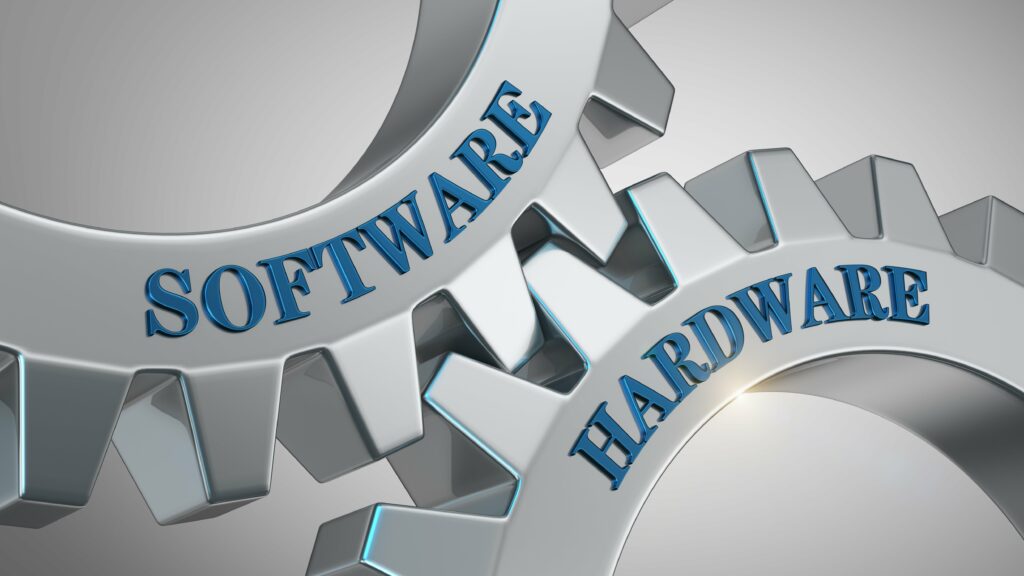
Multi Omics Data: Integration and Validity
Breakthroughs in high-throughput techniques and increasing availability of multi-omics data generated from a large set of samples. This has enabled incredible amounts of information to be gathered and analysed, several promising tools and methods have been developed for data integration and interpretation.
Integrating omics data through a computational pipeline often requires a huge storage space. The underlying pre-processing steps vary for different omics data because the data was generated using different technical platforms. Hence, integrating multi-omics requires creating a pipeline that integrates data generated from different workflows. It is important to ensure that the differences observed in the samples before integration are due to biological variability and are not a technical artifact of the data.
Horizontal and Vertical Integration
Most multi-omics datasets are organised “horizontally or “vertically”. Horizontal datasets are typically generated from one or two technologies, for a specific research question and from a diverse population and represent a high degree of real-world biological and technical heterogeneity. Horizontal or homogeneous data integration, therefore, involves combining data from across different studies, cohorts or labs that measure the same omics entities.
Vertical data refers to data generated using multiple technologies, probing different aspects of the research question, and traversing the possible range of omics variables including the genome, metabolome, transcriptome, epigenome, proteome, microbiome, etc. Vertical, or heterogeneous, data integration involves multi-cohort datasets from different omics levels, measured using different technologies and platforms.
The fact that vertical integration techniques cannot be applied for horizontal integrative analysis and vice-versa opens up an opportunity for conceptual innovation in multi-omics for data integration techniques that can enable an integrative analysis of both horizontal and vertical multi-omics datasets.
Conclusion
The discussion group concluded with a look to the future. A global, shared network of experiment data combined with the progress of organoid’s may one day answer some of today’s most pressing challenges.
At Oxford Global, we couldn’t have been more pleased with the turnout for our multi-omics and bioinformatics discussion group. The conversation was engaging, the debate stimulating, and the event provided the perfect setting for exchanging ideas. You can learn more about Oxford Global’s other omics discussion groups and upcoming events here.