Pathway Mining and Proliferation Markers: Refining the Identification of Cancers for Systemic Treatments Through Building Digital Twins of Disease Pathways
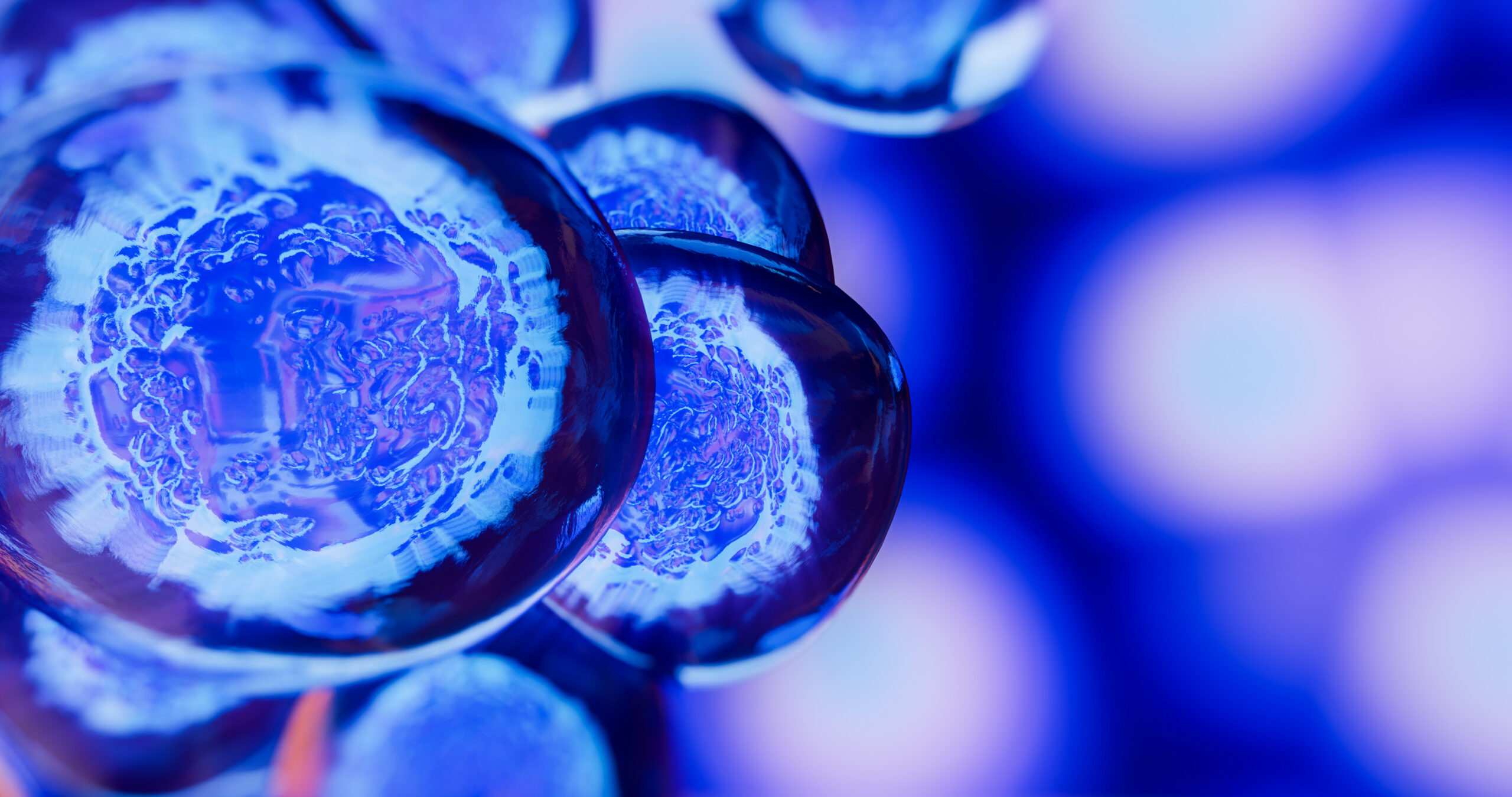
Presented by Graham Ball, Professor of Bioinformatics at Nottingham Trent University
Transcribed by Ben Norris
Graham Ball has spent a significant part of his career talking to people about machine learning. In the two decades since he started working in the field of Omics data, there have been huge advances in the technologies available for improving the development of new treatments. His chosen area of research has focused on developing a machine learning array for identifying disease targets associated with breast cancer, where there is an unmet need for treatment. The mission for Ball’s research team is to identify the best proliferation marker for guiding the choice of systemic treatments for breast cancer, with investigation of ‘cross-talk’ between tumour molecules through pathway mining potentially leading to a better understanding of breast cancer development.
Enhancement of Genome Analysis Through Machine Learning
Researchers chose P53, a common gene mutation in breast cancer patients, as the focus for proliferation and apoptosis-related pathways due to its association with anti-tumoural behaviour. The mutation is related to the regulation of uncontrolled cell growth, proliferation, and apoptosis, as well as DNA repair and the inhibition of angiogenesis. Ball’s study investigated the state of microarray cohorts, with a focus on merging datasets in parallel to eliminate false discoveries and improve the reliability of results. The project yielded rich datasets, which all contained transcriptomic data in addition to clinical and pathological data.
The Cancer Genome Atlas (TCGA), a database established by the National Cancer Institute in America to record genomic data about different cancer types, was used as a validation cohort to cross-reference observations. TCAG-derived figures and information were used for classification and parameterisation of data, with the concept — as explained by Ball — being that biomarkers could be used to identify other biomarkers. As in other biomarker-focused fields of research, the current approach is to be able to manufacture ‘one drug for all individuals’, which would enable much faster provision of treatment to patients in need.
- Transforming Clinical Development with Biomarkers
- The Patient-Focused 'Paradigm Shift' That Could 'Revolutionise' Clinical Trials
- New Developments in Multiplex Immunofluorescence Using Spatial Technologies
It is possible to profile individuals through proteomic and transcriptomic technologies to look for specific gene and protein expressions, allowing for the stratification of key phenotypic groups. Specific methods for mapping biological pathways and the interactions between them involve the use of non-linear discovery tools, including Intellomx Distiller, Intellomx Pathway Miner, and Intellomx Driver.
Pathway Mining and Biomarker Identification
The process for gene enrichment involves analysing the distribution of the performance of single genes through an artificial neural network (ANN)-based gene set enrichment. Intellomx Distiller was used to seek commonalities between different molecules, with the process repeated for each marker in the pathway. Interactions were subsequently enriched based on the strength of interactions between specific biomarkers that occur repeatedly across randomly enriched lists. “If it occurs repeatedly across 20 lists then the correlation is very strong,” Ball explained. There are principles that determine the interactions of pathway miners: the software can nominatively ‘mine’ interactions from whole transcriptomes and repeat this process for each marker in the P53 pathway.
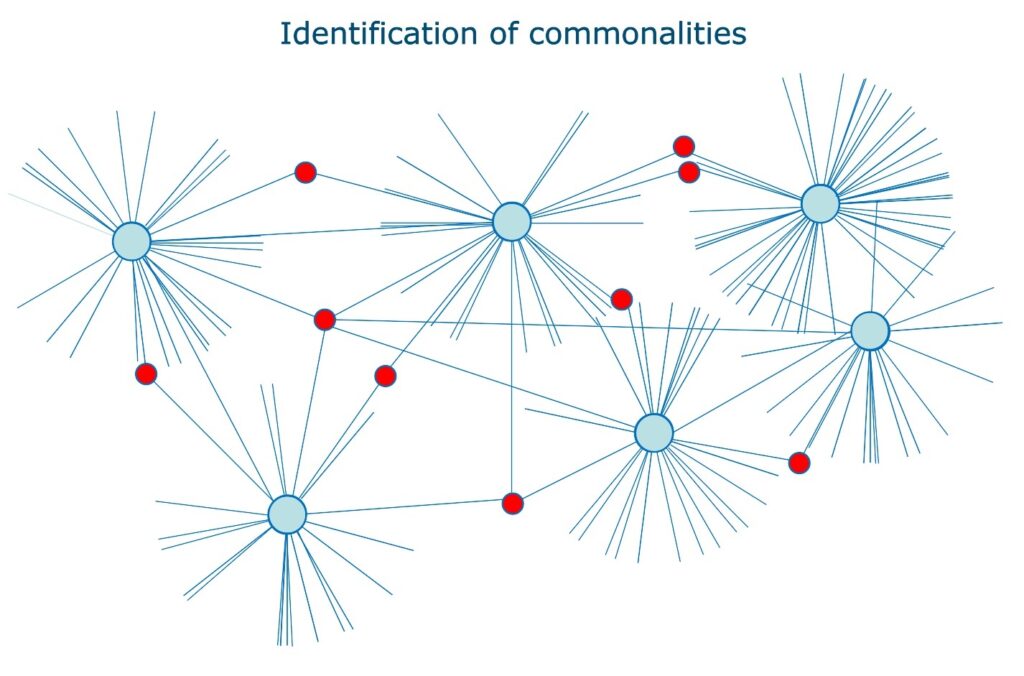
Ball stressed that there is more to the field than linear analysis, emphasising the applications of biomarker modelling. “Biology is not just a list of molecules… through our development of a digital twin of the pathway, we identify the most influential molecules in the system — the drivers of that system — and that’s based on a matrix of interaction.” The process of interference modelling identifies the most influential markers or drivers in a pathway and enables the examination of their interactions in the context of a diseased state versus a healthy state. Strongly influential markers have been observed to define phenotypes via potential therapeutic targets, meaning modelling driven by pathway mining can be strongly targeted to a particular organism or disease.
Cause and Effect: Phenotypic Markers
One of the most compelling findings from Ball’s team is that strongly influential markers are observed to define a phenotype. Additionally, select phenotypes can be switched on and off by knocking out those markers. This makes them highly targeted to both the organism and the disease, which could enable the targeted deactivation of malign tumours in future therapies. A preliminary study knocked out proliferation in cells, which also managed to prevent ripening in tomatoes. “It’s a bit like sticking your finger into the middle of a spider’s web,” continued Ball. “If you knock out the hub you remove that whole area of effect.”
“If you knock out the hub area you remove that whole area of effect… it’s a bit like sticking your finger into a spider’s web.”
Modelling enables the construction of a matrix of interaction, so the influences on feedback processes can be modelled and analysed. This kind of pathway modelling in a controlled study can be intensely complex, necessitating a methodical approach to pathway mining and analysis. Driver analysis can identify the most influential molecules in each system, which collapses the inferred network by summing features. “Basically, it’s taking this complex matrix and collapsing it down to allow us to rank the markers,” explained Ball.
Potential Applications of Omics Data Analysis
Results gathered by Ball’s team indicate that the drivers discovered through the pathway mining study change the biology of the disease, suggesting that future pathway modelling approaches may enable the deactivation of potentially cancerous or mutated cells. One of the several applications of this approach is to MultiOmics data. “The transcriptome isn’t the end of the story," Ball elaborated. "What we’re effectively doing is developing a digital twin.”
This approach is an emerging technique which is well-known in other engineering disciplines, but applying it to biology is still novel. “Now we’re looking at the potential for identifying pathways in tumours, but also seeing how those pathways behave in stroma." One future challenge for the interrogation of Omics data concerns tissue heterogeneity; Ball outlines sub-cellular populations and regional tumour populations as areas that need consideration. “The interplay between Omics and machine learning has to be more closely tied in to keep developing algorithms that are suitable.”
If you want to stay up to date on the latest developments in cancer identification and treatment, our Biomarkers series has frequent updates from leading figures and researchers in the industry. Register here for our upcoming Biomarkers UK: In-Person event.