Multimodal Biomarkers: How Screening Approaches Can Benefit From AI
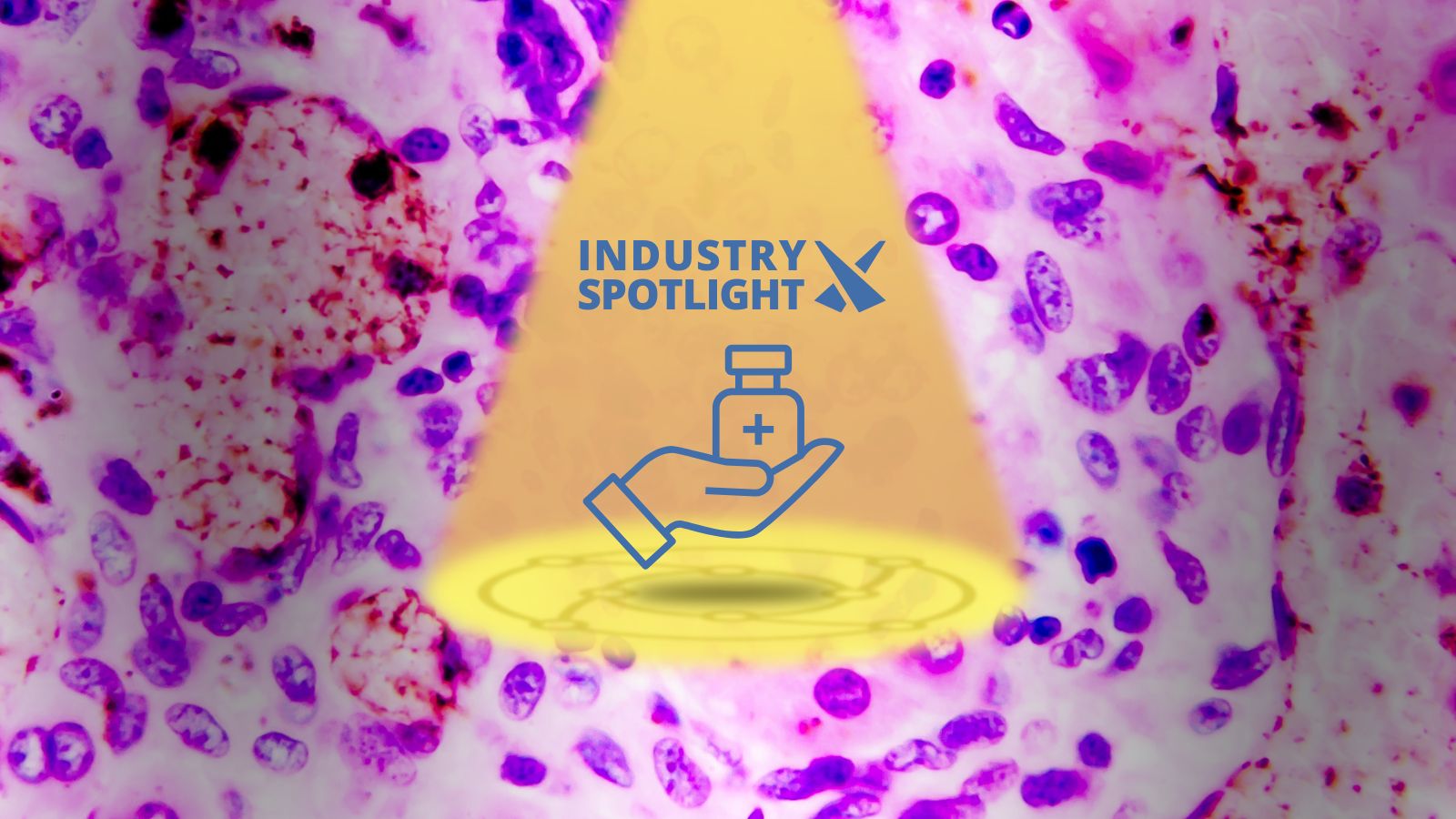
Multimodal biomarkers may help to personalise decision-making following screening scenarios for men with high-risk prostate cancer.
Broadly, the current applications of AI in medicine have addressed narrowly-defined tasks using one data modality, such as a computed tomography (CT) scan or a retinal photograph.
The development of AI models that incorporate data across different modalities is poised to bridge the gap and enable the application of multimodal biomarkers in individualised medicine.
An article featured in Urology Times discussed the results of a prognostic trial over standard clinical and pathological variables.
In the trial, a multimodal AI biomarker was able to predict which men with high-risk disease had the highest risk for a distant metastasis or prostate-cancer specific mortality.
These results can be used to inform treatment for patients, helping clinicians to select an approach that will be beneficial to the patient without causing them undue stress or discomfort.
Multimodal Biomarkers in High Risk Prostate Cancer
Findings from the study, pioneered by Daniel E. Spratt of Case Western Reserve University, stated that a multimodal artificial intelligence (MMAI) biomarker was successfully validated across six phase III randomised trials.
In the study, data from almost a thousand patients was analysed, with a set of seven genes significantly associated with biochemical recurrence (BCR) identified in patients with prostate cancer.
A machine learning model was used to identify biomarkers which can predict the likelihood of this biochemical recurrence.
- Best-practice approaches for investigating issues posed by NASH and liver cirrhosis
- Keytruda sees trial success in treating endometrial cancer patients
- Eli Lilly focus on Phase III trial for Alzheimer's treatment following FDA setback
Despite all patients having high-risk disease, the MMAI biomarker identified those with highly variable risks for metastatic disease and prostate cancer-specific mortality.
The algorithm was also able to accurately identify and classify different regions of prostate cancer on the MRI images, which may have important implications for planning treatment and monitoring disease progression.
The model received a prediction accuracy rate of 75%, which is higher than that currently used in clinical parameters.
Further research will be needed to validate these findings and allow the full integration of AI into clinical urology practice.
Still, overall the data from these studies demonstrates the potential of AI in identifying predictive and prognostic multimodal biomarkers in prostate cancer, as well as other clinical diseases.
Get your weekly dose of industry news and announcements here, or head over to our Biomarkers portal to catch up with the latest advances in cellular therapies.
Related Resources
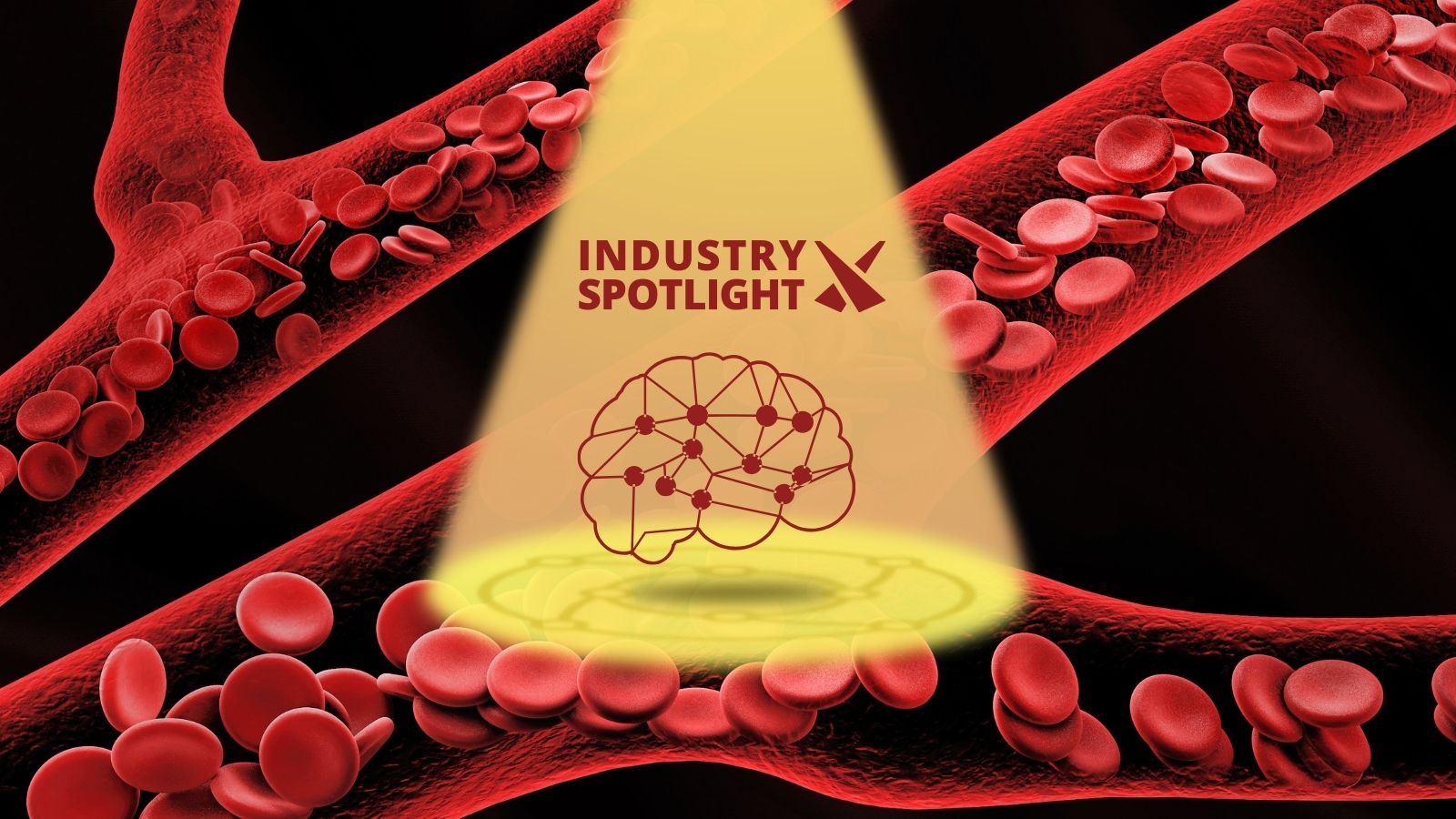
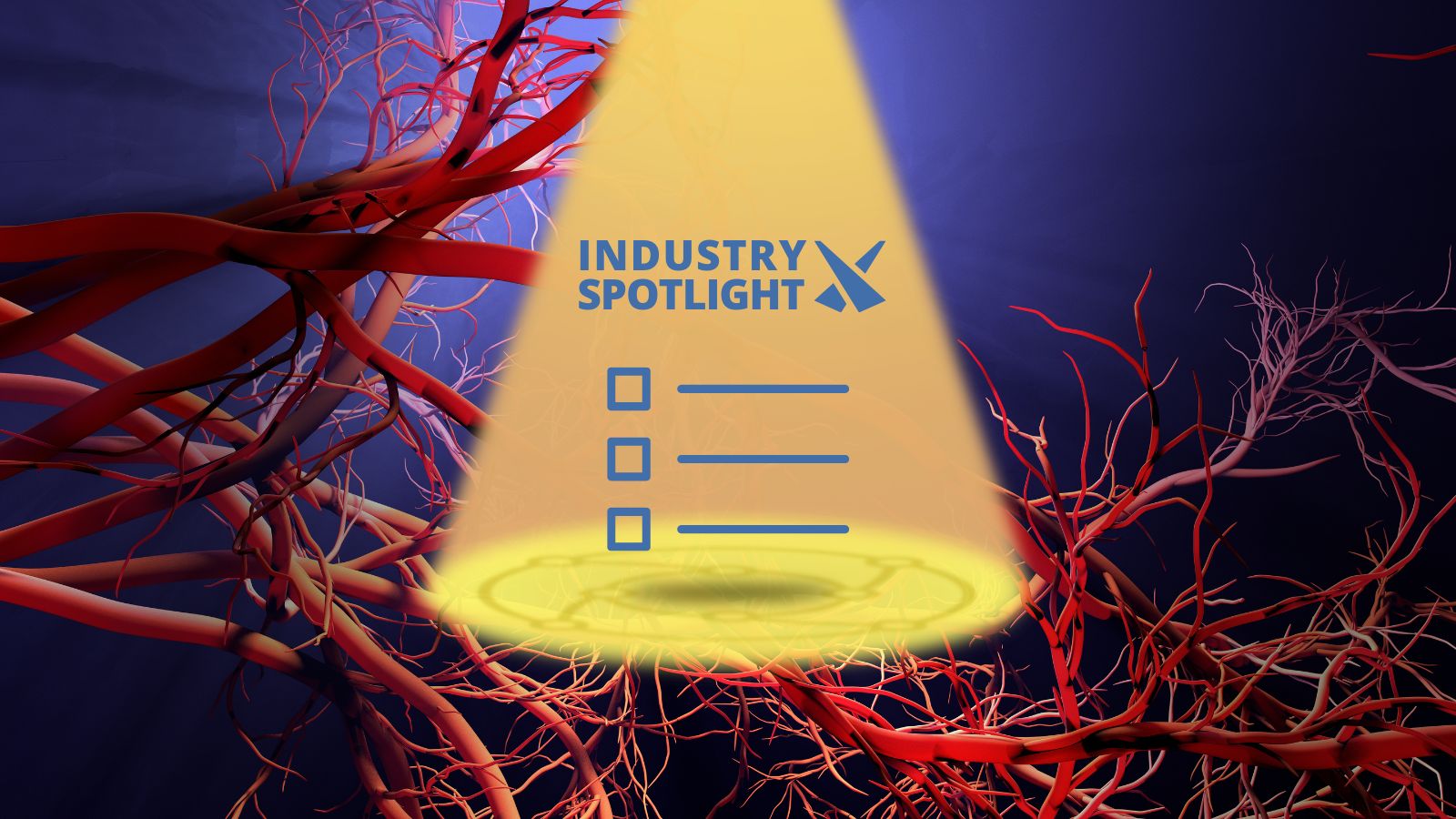