Integrating Machine Learning into Single-Cell Omics Analysis
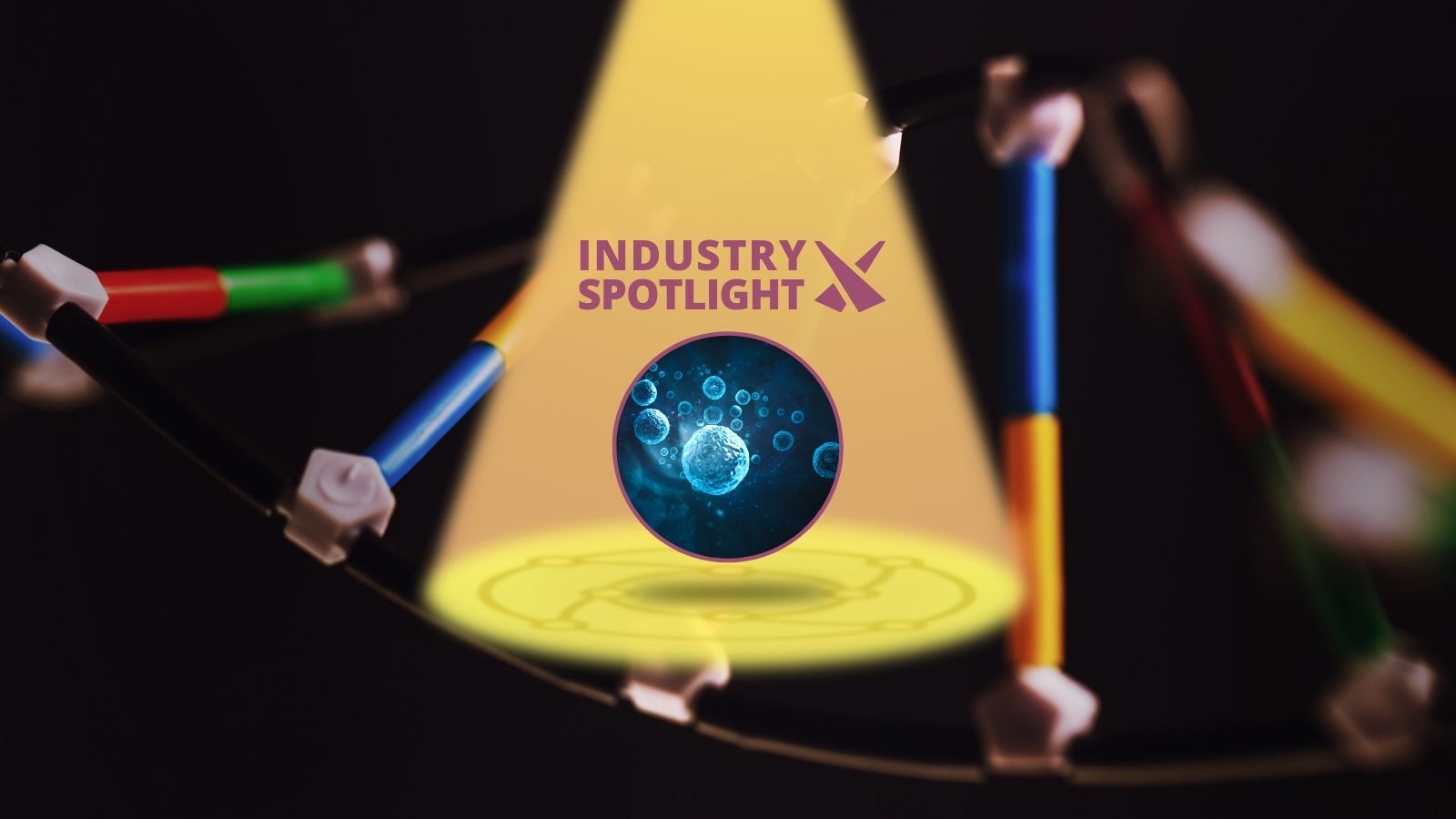
Single-cell omics technologies produce huge amounts of data describing the genomic, transcriptomic, or epigenomic profiles of many individual cells in parallel.
With the volume and breadth of data generated, computational models are urgently needed to process the large amounts of data produced by single-cell omics technologies.
To infer biological knowledge and develop interpretable predictive models, machine-learning (ML)-based models are increasingly being used on account of their flexibility, scalability, and success in other fields.
The past five years have seen a surge in new ML-based method development for low-dimensional representations of single-cell omics data, batch normalisation, cell type classification, and multimodal data integration.
Many of the recent advances in the field have seen deep learning used to automatically extract information from large sets of single-cell data, tackling problems such as batch normalisation and multimodal data integration.
This also marks a period where systemic benchmarks started to highlight the challenges associated with these methods, as well as their potential to accelerate and streamline the interpretation of data obtained from single-cell imaging.
Applying ML Approaches to Improve Data Interpretability
Raw single-cell transcriptomic count data, as well as their epigenomic counterparts, provides a high-dimensional and noisy depiction of each cell by assessing the activity of thousands of genes in a DNA loci simultaneously.
Dimension reduction techniques represent a useful step for removing technical noise from a sample, and for preparing data for visualisation, classification, and further analysis.
Jointly analysing single-cell transcriptomic data from many experiments - potentially from different laboratories using different technologies - is a key strength afforded by integrated analysis facilitated by ML.
This can be achieved through the integration of several modalities in order to enhance the performance of downstream tasks such as cell type labelling and the identification of subpopulations.
Several ML approaches developed for this purpose include characterising cells across measurements or projecting measurements into a common latent space.
However, while the potential utility afforded by the batch correction and integration of heterogenous scDNA-seq data is significant, this approach runs the risk of capturing batch effects and other confounding factors.
Determining the Right Strategy for ML in Single-Cell Omics
Along with the increase in the volume and resolution of single-cell genomics data, there has been an explosion in the tools available for dealing with single-cell genomics data.
Over 800 tools have been published for scRNA-seq analysis so far, many of which have been based on ML approaches.
Of these, the majority have been imported from other fields, with some features subsequently unsuited for genomic challenges and the reality of biological data.
Subsequently, researchers looking to augment their data interrogation have to choose carefully when looking at ML approaches to use for augmenting single-cell data interpretation and analysis.
Get your regular dose of industry news and announcements here, or head over to our Omics portal to catch up with the latest advances in tumour analysis. To learn more about our upcoming NextGen Omics UK conference in London, click here to download an agenda or register your interest.