AI in Digital Pathology: The Applications of Explainable AI and Deep Learning Approaches in Clinical Practice
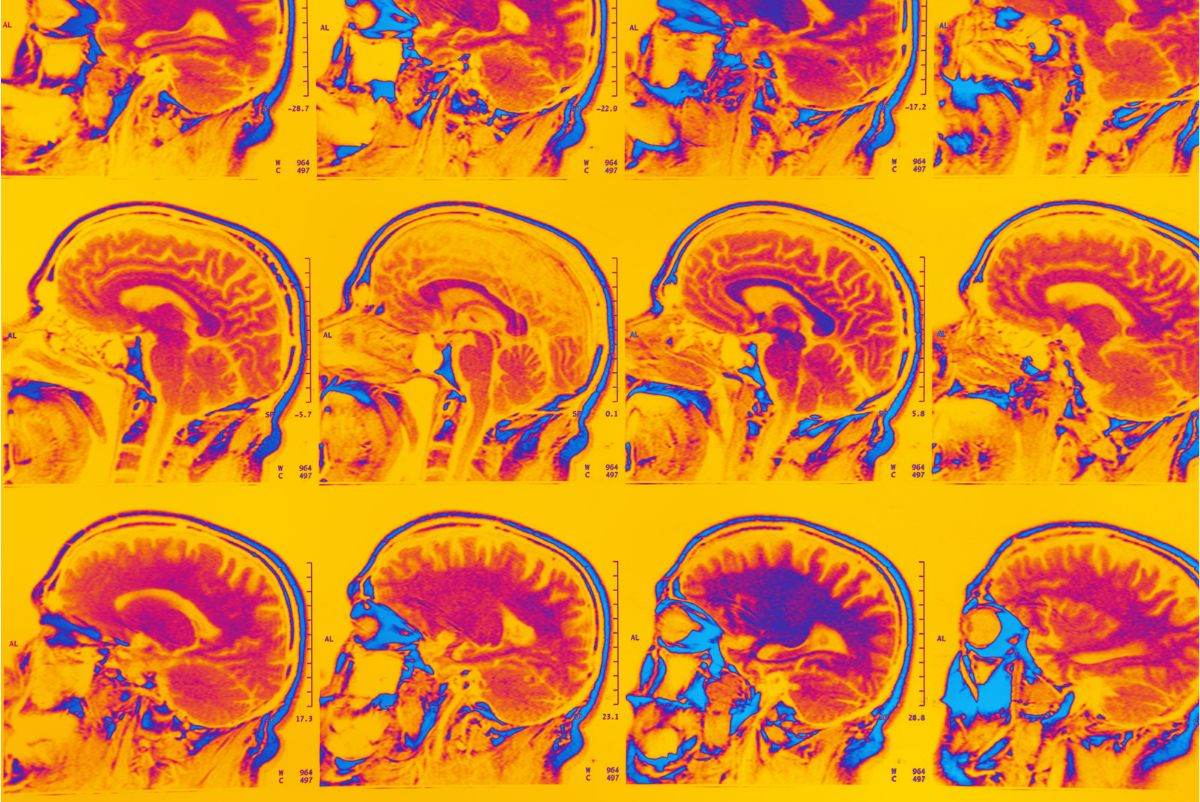
With an ever-growing number of medical biomarkers making their way into clinical practice, machine learning algorithms are forecast to become a tool regularly used to help medical researchers navigate the huge volume of healthcare data now available. However, algorithm transparency has been highlighted as a key consideration to address before these approaches are commonly adopted. Should artificial intelligence (AI)-generated data be used to inform a medical decision without first being interpreted?
Explainable AI — validational information that allows users to interrogate the results of machine learning outcomes — is a major focus among digital pathologists. Some of the industry’s leading figures discussed pros and cons at Biomarkers 2023, evaluating where the field is now and assessing what more needs to be done before explainable AI approaches can be broadly adopted in the clinic. Here, we discuss some of the potential applications of explainable AI in digital pathology and the monitoring of neurodegenerative disease progression.
Neurodegenerative disease research is one field that could benefit from a range of innovations in computer processing power and advances in AI programming complexity. Potential applications of AI in this field include the diagnosis and early detection of diseases such as Alzheimer’s, Parkinson’s, and Huntington's, reinforcing predictions of disease progression.
AI Approaches in Neurodegeneration and Neural Psychiatry
Initial studies have applied natural language processing to patient datasets in the context of their medical history to help screen for known signs of neurodegenerative disorders. AI technology can also be used to predict cognitive impairment and anticipate how severely a patient’s motor skills may decline over time. Researchers may also employ AI in digital pathology to discover potential drug and biological targets that could augment future treatment options.
Prognostic biomarkers — clinical or biological characteristics informing clinicians of probable patient health outcomes following treatment — are central to neurodegenerative research. These enable the categorisation of patients according to their risk of disease progression or death, and accordingly can be used to adjust treatment intensity for individual patients. Researchers can gain a more detailed understanding of disease states by analysing multiple molecular biomarkers together, helping in the development of personalised treatment plans.
Anja Hijzen, Associate Director and Clinical Scientist at Johnson & Johnson, believes there is significant potential in the digital pathology field. “I’m very keen to see how we can implement AI in neurodegeneration and neural psychiatry,” said Hijzen. “How can we use pattern recognition and those very specific algorithms to diagnose a disease like Alzheimer’s? It’s an open space for me, but I think we can transform the way we are doing it.”
Digital Pathology: Coming to Incorporate Deep Learning Approaches
The growing number of complex molecular biomarkers available to clinicians has led to an increase in both cost and time for decision-making in routine daily oncology. Advances in deep learning have enabled the extraction of previously unobtainable information — for example, from routine histology images of cancer. However, this has increased the volume of data that clinicians need to wade through in the process of arriving at a diagnosis.
These recent strides have left the pathological community divided regarding best-practice approaches to data interpretation and integrating deep learning into the clinic. Kevin Randall is Lead Scientist in Molecular Pathology at Catapult Medicines Discovery, and has seen the development of computerised image analysis for pathology from its infancy in the 90s through to the implementation of deep learning approaches. His main goal is to break down barriers for the adoption of modern technologies and make it easier for new drugs to come to market.
In Randall’s experience, the pathology community is conservative in its outlook, which can delay the uptake of new technologies which might speed up developmental processes. “It’s taken them years to accept looking at digital pathology for images rather than looking at glass,” he said. However, this change would not be a question of AI in digital pathology replacing pathologists. “It’s a tool that we can use to improve outcomes for patients, because that’s the basis of why we’re doing this.”
Encouraging Explainable AI in Digital Pathology
A key opportunity for this improvement of outcomes is in timesaving approaches for assay standardisation, as Randall explained through an anecdotal account of a clinical trial on PD-L1 (Programmed Death Ligand 1). “The pathologists who were involved in that clinical trial had to have two days’ training to be able to consistently report on those biopsy samples,” said Randall. “And that’s with one stain.” In the field of precision medicine, many different assays require interpretation. “Where are pathologists going to find the time to have two days’ training so they’re consistent across the board?”
- Using digital biomarkers for disease detection and monitoring
- How smartphones have enabled the passive monitoring of neurodegenerative diseases
- Prognostic insights courtesy of the tumour microenvironment
If explainable AI is more heavily integrated in digital pathology, explainability and transparency will be crucial to gaining the trust of pathologists. “The majority of pathologists I’ve talked to about deep learning are very wary of black box approaches,” said Randall. “If they can’t explain to their clinicians how a decision is being arrived at, they don’t feel as if they can accept it.” He called for a rationale for black boxes, with overlays to demonstrate to pathologists the features which a deep learning program would regard as important. “Not only is it relevant to the pathologists, but it might lead to a better understanding of the disease process as well.”
Integrating AI and Neural Psychiatry into Digital Pathology
Data access is also a constraint in the discipline of digital pathology. Tim Showalter is Vice President of Clinical Development at Artera, and Professor of Radiation Oncology and Urology at the University of Virginia. He feels that many talented AI teams within biopharma are constrained by the data they have access to. While high-quality data needs to be interpreted well, well-trained data interpreters need access to that data in the first place. “The answer is bringing folks within the industry together to figure out what the right risk tolerance is and what the opportunities are, and that’s challenging,” said Showalter.
While some challenges and limitations for AI in digital pathology include a barrier to adoption, the ability to rapidly process large amounts of tissue data with a high degree of accuracy makes it a potentially invaluable resource for the treatment of diseases which are highly heterogeneous. “I think we need some new alternatives,” said Hijzen. “For these diseases, the information about how they present and how we could personalise treatment would be really important. If we could achieve that, that would be fantastic.”
Want to learn more about the applications of AI and machine learning in digital pathology? Head over to our Biomarkers portal for the latest news and updates on advances in the field and insights from the industry’s best and brightest. If you’d like to learn more about our upcoming Biomarkers US conference, visit our event website to download an agenda and register your interest.