National University of Singapore Unveil Novel Single-Cell RNA Analysis Technique
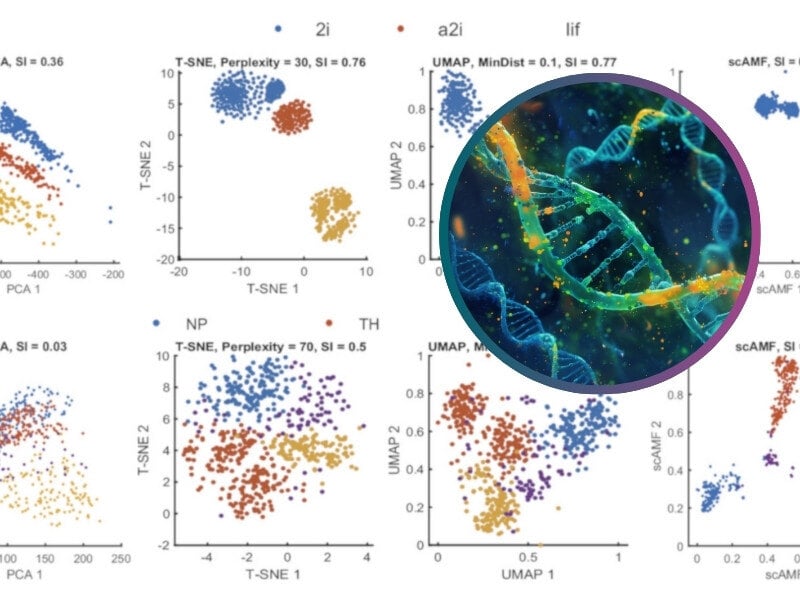
Researchers at the National University of Singapore (NUS) have outlined a novel mathematical approach for analysing single-cell RNA sequencing (scRNA-seq) data. The technique hopes to improve the speed and accuracy of analysis of scRNA-seq data through the enhanced clustering of cell types.
The Singaporean team, led by Associate Professor Zhigang Yao at NUS’s Department of Statistics and Data Science, have named their method scAMF (Single Cell Analysis via Manifold Fitting).
Related:
- Research Spotlight: Multi-Omics Data Harmonization for SARS-CoV-2 Virus and Drug Target Identification
- In Conversation With... Fan Zhang, Assistant Professor at the University of Colorado
Traditional forms of scRNA analysis which aim for low dimensional data representation can be inaccurate at delivering stable scRNA-seq clustering. scAMF aims to solve this need by fitting the data as a low dimensional manifold within the high dimensional space where the gene expression data are measured.
According to the team, this method is able to reduce noise in the representation of these data while also preserving the information necessary for the characterisation of cell types and states. By reducing noise, scAMF is able to improve the spatial distribution of scRNA-seq data: bringing together gene expression vectors of cells of the same type and clearly delineating between those different cell types.
Yao said: "Our approach effectively denoises scRNA-seq data by fitting a low-dimensional manifold in the high-dimensional space. This method significantly improves the accuracy of cell type classification and the clarity of data visualisation."
The team have had their work published in the Proceedings of the National Academy of Sciences, where Yao was lead author. The article explains:
This strategy effectively reduces distances between similar cell types while preserving expression information, significantly improving scRNA-seq clustering and visualization compared to existing state-of-the-art techniques. This groundbreaking advancement establishes a standard in scRNA-seq analysis, opening exciting avenues for future research and potential clinical applications.

Manifold Fitting (RHS) clusters raw data (LHS) into discrete clusters of cell types.
Credit: PNAS, Zhigang Yao et al. https://doi.org/10.1073/pnas.2400002121
Furthermore, the article outlines scAMF’s effectiveness at visualising higher dimensional scatter data in lower dimensions while preserving clustering of datapoints, as compared to other methods. It explains:
In analyzing the Kolodziejczyk data (Top row of Fig. 5A), PCA demonstrates limited class differentiation, with greater variation within classes than between them. T-SNE, akin to PCA, manages some separation but indicates potential within-class divisions, likely arising from batch effects in the dataset. UMAP, however, inaccurately splits the “2i” class into two distinct clusters. In contrast, scAMF effectively segregates the three classes, achieving clear distinction and creating densely packed clusters for each class, thus demonstrating its superior clustering capability.
Clustering of data using scAMF compared to other methods (T-SNE, UMAP, and PCA), with ‘silhouette index’ provided.
Credit: PNAS, Zhigang Yao et al. https://doi.org/10.1073/pnas.2400002121
Yao has worked on manifold fitting as a method of non-linear data analysis before in a study which said it had “profound implications in statistics and computer science, especially for dimensionality reduction and processing non-Euclidean data.”
Next, Yao’s team want to use what they learned in building scAMF to construct high-resolution, multiscale cell atlases.
Related:
"Our ongoing work has already shown promising results across numerous benchmark datasets, revealing novel biological insights," said Yao. "We've applied it to the Human Brain Cell Atlas and identified new subtypes and marker genes for various cell types."