Data Environments in Healthcare: Real-World Datasets and Data Integration
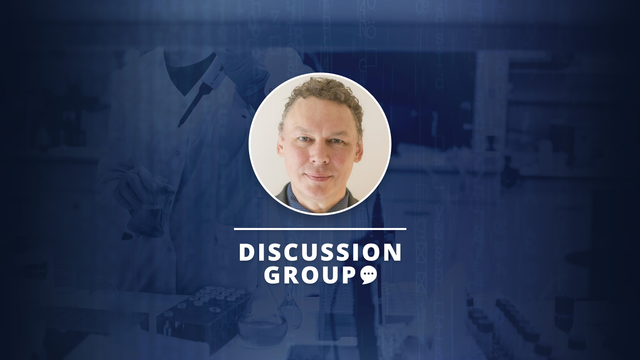
Data environments in healthcare are a major driver for the integration of real-world data (RWD) into the pharmaceutical industry, with the need for more detailed data available at lower cost. Currently, the focus is on developing automation processes that will arrive at the correct outcome following good science principles, with an emphasis on the incorporation of AI/ML approaches in drug development.
November’s PharmaTec Discussion Group was hosted by Igor Rudychev, Vice President at Enterprise Analytics, and focused on the applications of Real-World Datasets and Data Environments. Rudychev opened the discussion by emphasising the broad applicability of (RWD), explaining that it spans multiple countries and even multiple continents. Some of the main focuses of the hour’s discussion included RWD usage in information generation and its applicability in bioinformatics.
Rudychev started by defining RWD as information captured for application in clinical settings through various types of data capture software. He said that a significant amount of healthcare data comes from insurance claims, patient registries and data gathered from outside clinical trials. Some clinical trials may differ from RWD by data composition.
Another source of medical data is that collected through smart devices — hundreds of millions of patients generate data from their smartphones. With the proper frameworks implemented, this patient-generated data can be linked with clinical insights. Additionally, data governance frameworks can be a valid source for information. This discussion explored the applications and finer points of data environments in healthcare.
Data Environments in Healthcare: Data Silos
Rudychev asserted that there had been a revolution in RWD regarding genomics and patient instructional data. Some of the present challenges facing RWD implementation right now include issues with patient history, which may be incomplete across different medical and clinical institutions. Even concerning local specialists, information may be lost when patients are transferred between clinics.
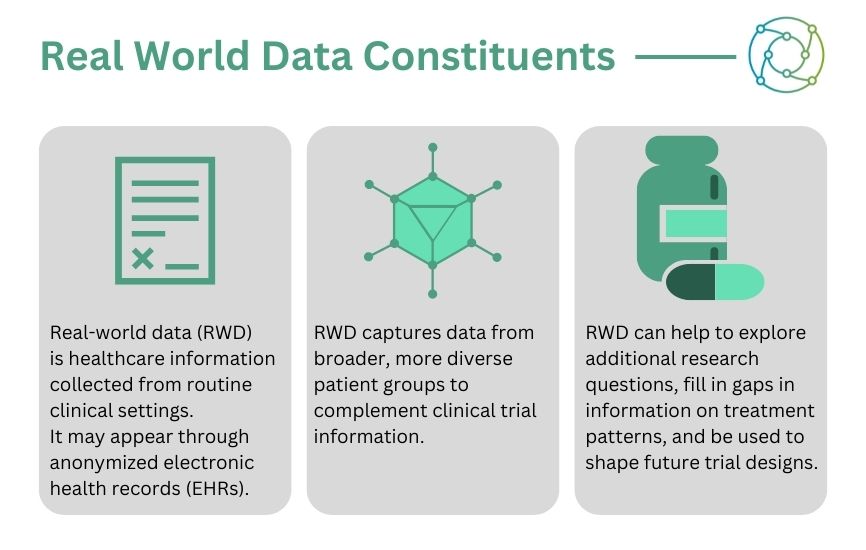
A key focus in RWD implementation has been on obtaining a clearer picture of patient history and disease characteristics. However, one of the trickiest issues from the clinical data standpoint is the capture of data within the clinical pipeline. Rudychev offered that the difference with EMR data sources was that when a patient moves on from a particular clinic this is not reflected in the records. In his view, data publication is a big issue for understanding etymology and how many patients with a particular healthcare issue live in each country.
Confronting Major Challenges in RWD
Another focus in data environments in healthcare regarding the management and maintenance of RWD is data leakage — where other companies have patients who leave healthcare systems and transfer to others, resulting in a loss of patient visibility. One member of the audience said having access to all available patient data was crucial in this regard. “This would involve combining clinical notes with clinical applications,” they said. “We’re looking at the ability to link that at a patient level — that’s the Holy Grail.” Rudychev voiced his complete agreement, adding that missing or patchy lab result data was a big challenge regarding patient data for injectables.
“I don’t think there’s a single answer to ‘what’s the biggest challenge’,” they continued. “I think that continually evolves depending on the nature of the question.” Another attendee said they were in the process of forming patient databases for large levels of drug candidates. “How do you define the most specific molecule within the trial, and how do you combine those different asset prospects?”, they asked. “These are the questions we need to answer to address those smaller differences.”
Hurdles to Overcome in Implementing Data Environments in Healthcare
Another important consideration in the handling and integration of RWD is the source of this information. In this context, access to a variety of information is key to understanding how a product is performing in preclinical or clinical settings. Rudychev said that in the past he had found it useful to have one platform with a pipeline. However, currently there is no one approach that can be used without human oversight, and he added that putting together fragmented datasets was a big challenge.
“If you haven't thought about how you're going to incorporate real-world data into subsequent analysis, you're going to limit yourself.”
“I think there are a number of major gaps,” concurred another audience member. “Sometimes you’re using things in your inclusion and exclusion criteria that aren’t normally available in the outside world.” Integrating RWD into a clinical trial is a massive boon for validity and dataset integrity, particularly when dealing with external data. However, they added that any efforts to incorporate RWD require a high amount of judgement in the trial’s design. “If you haven’t thought about ‘how am I going to incorporate real-world data into subsequent analysis’, you’re going to limit yourself because you’ve measured things in a way which is not compatible.”
Summaries and Conclusions Regarding RWD
Some future challenges regarding RWD that require addressing include the fact that most aggregated RWD sources are a few years old, so as datasets they may not reflect the latest clinical data. Additionally, RWD often only provides a partial view of a patient’s journey and may lack information on medical visits. This can result in patchy information on lab results, treatments, procedures, and genetic information that would otherwise help to provide a more well-rounded picture of a patient.
- Read: Oxford Global's R&D Key 40 Stories
- The Applications of Machine Learning in Clinical Data Analysis
- Utilising AI and Machine Learning in Computational Drug Design
With all said, RWD analyses has several limitations. One is that the source and type of data used may limit the generalisability of the results and of the endpoints. Additionally, observational real-world studies can only evaluate association and not causality. Due to these limitations, real-world data analyses cannot be used as standalone evidence to validate the efficacy and safety of a treatment. However, RWD now represents a major source of information for understanding current outcomes and improving future analysis in data environments in healthcare.
Rudychev rounded off the discussion by asserting that now, increasingly, clinical trials are based in genomics. He added that Oxford Global’s initiative was a great approach to creating an open forum for series-specific topics. Rudychev highlighted potential future topics such as the utility of preclinical data, as well as discussions around the current availability of real-world data at the patient level.
Want the opportunity to take part in future discussion groups? Check out our membership options to gain full access to all upcoming discussions in the PharmaTec series, as well as exclusive content. If you’d like to attend our upcoming Pharma Data and Digital Medicine UK: In-Person congress, visit our event website to download an agenda and register your interest.