The Power of Real-World Data Analytics in Biomarker Discovery: A Game-Changer in Medical Research
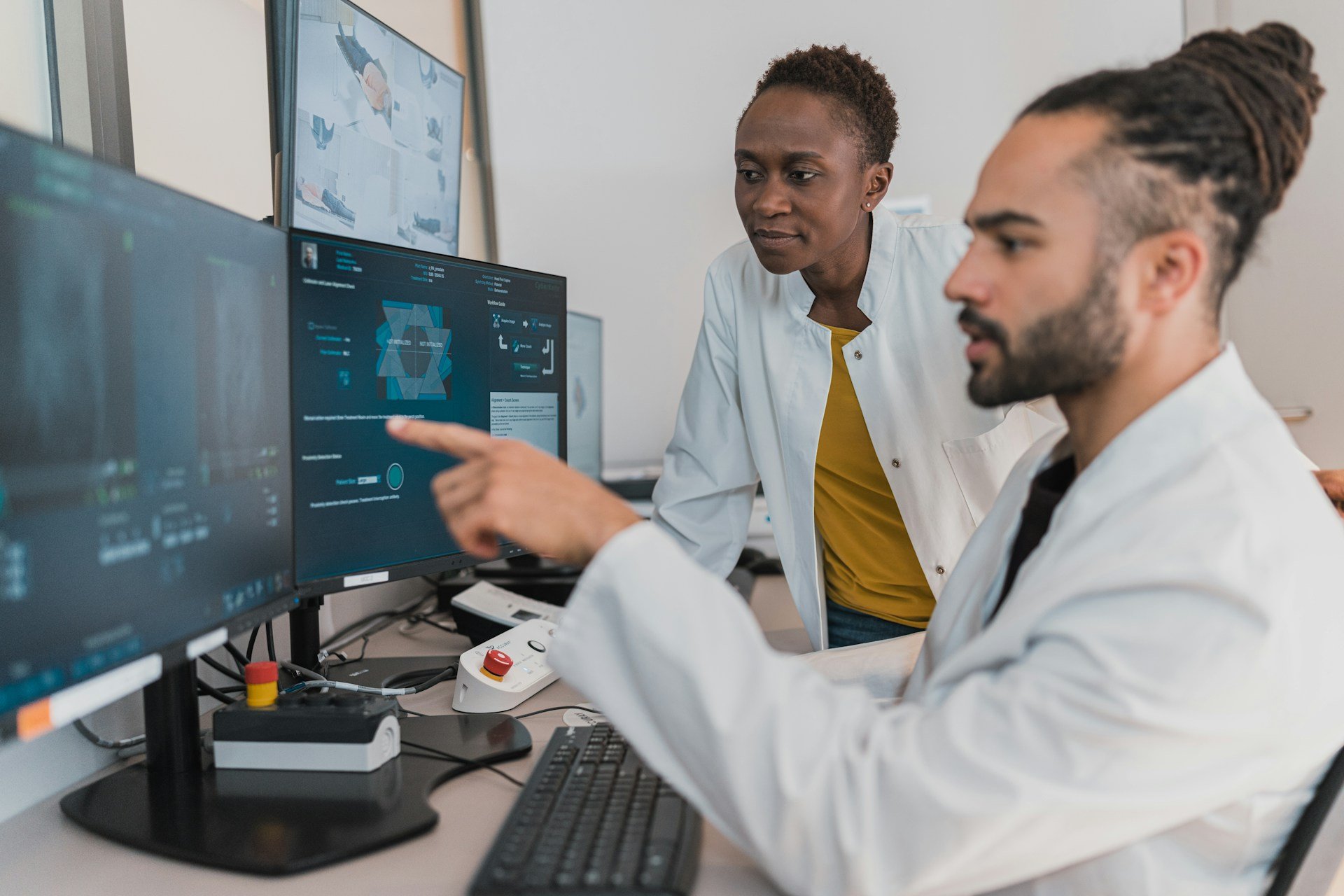
Biomarker discovery is a crucial aspect of medical research, as it involves identifying measurable indicators that can be used to diagnose and monitor diseases. These biomarkers play a significant role in understanding the pathophysiology of diseases, identifying potential therapeutic targets, and improving patient outcomes. Traditionally, biomarker discovery has relied on controlled clinical trials and laboratory experiments. In this article Biomarkers by Oxford Global further explores the challenges, application and best uses for Real-World Data.
What are Real-World Data Analytics?
Real-world data analytics refers to the analysis of data collected from routine clinical practice, such as electronic health records, claims databases, and patient registries. This data provides valuable insights into patient characteristics, treatment patterns, and outcomes in real-world settings. Real-world data analytics leverages the power of big data and advanced analytics techniques to uncover patterns, associations, and trends that can inform medical research and decision-making. By analysing real-world data, researchers can gain a better understanding of disease epidemiology, treatment effectiveness, and patient outcomes.
What is the Role of Biomarkers in Medical Research?
Biomarkers are measurable substances or characteristics that indicate the presence or progression of a disease. They can be found in various biological samples, such as blood, urine, or tissue. Biomarkers can provide valuable insights into disease mechanisms, aid in early diagnosis, predict treatment response, and monitor disease progression. For example, in cancer research, biomarkers can help identify specific subtypes of cancer, predict patient response to treatment, and monitor disease recurrence. The discovery of novel biomarkers is essential for advancing medical research and improving patient care.
What are the Challenges in Biomarker Discovery?
Traditional approaches to biomarker discovery have several limitations. Clinical trials, although valuable, are often expensive, time-consuming, and may not capture the real-world complexities of patient populations. Additionally, laboratory experiments may not fully reflect the heterogeneity and diversity of patients seen in clinical practice. Furthermore, the identification of biomarkers from complex datasets requires sophisticated analytical methods and tools. These challenges have led researchers to explore alternative approaches, such as real-world data analytics, to overcome these limitations.
The Importance of Real-World Data in Biomarker Discovery
Real-world data offers several advantages in biomarker discovery. Firstly, it provides a more comprehensive and representative view of patient populations, including those who may be underrepresented in clinical trials. This diversity allows for the identification of biomarkers that are relevant across different patient subgroups. Secondly, real-world data provides a longitudinal perspective, capturing the natural history of diseases and treatment outcomes over time. This longitudinal data enables the identification of biomarkers that can predict disease progression or treatment response. Lastly, real-world data can be used to validate biomarkers discovered through experimental approaches, providing real-world evidence of their clinical utility.
Real-World Data Analytics in Biomarker Discovery: A Game-Changer
The integration of real-world data analytics in biomarker discovery has the potential to revolutionize medical research. By leveraging large-scale datasets, advanced analytics techniques, and machine learning algorithms, researchers can uncover hidden patterns and associations that may not be apparent in smaller, controlled studies. Real-world data analytics enables the identification of novel biomarkers, the discovery of new disease subtypes, and the prediction of patient outcomes. This approach can accelerate the translation of research findings into clinical practice, ultimately improving patient care.
Applications of Real-World Data Analytics in Biomarker Discovery
Real-world data analytics has been successfully applied in various areas of biomarker discovery. For example, in cardiovascular research, real-world data has been used to identify novel biomarkers for predicting the risk of heart disease and stroke. In oncology, real-world data analytics has helped identify biomarkers that can predict patient response to immunotherapy and guide treatment decisions. Real-world data has been instrumental in identifying biomarkers for rare diseases, where traditional clinical trials may not be feasible due to small patient populations. These applications highlight the versatility and power of real-world data analytics in biomarker discovery.
Case Studies Showcasing the Power of Real-World Data Analytics in Biomarker Discovery
Several case studies have demonstrated the transformative potential of real-world data analytics in biomarker discovery. For instance, a study analysing real-world data from a large patient cohort identified a novel biomarker for early detection of Alzheimer's disease. This biomarker not only showed high diagnostic accuracy but also correlated with disease progression and treatment response. Another study utilized real-world data to identify a biomarker signature that predicted the risk of developing type 2 diabetes in at-risk individuals. These case studies exemplify how real-world data analytics can uncover clinically relevant biomarkers with significant implications for disease diagnosis, monitoring, and treatment.
Prospects and Advancements in Real-World Data Analytics in Biomarker Discovery
The field of real-world data analytics in biomarker discovery is rapidly evolving. Advances in data collection, integration, and analysis techniques are constantly improving our ability to extract meaningful insights from large-scale datasets. Machine learning and artificial intelligence algorithms are becoming more sophisticated, enabling the identification of complex biomarker patterns and associations. Collaborations between researchers, clinicians, and industry partners are essential for harnessing the full potential of real-world data analytics in biomarker discovery. The future holds great promise for the continued advancements and applications of real-world data analytics in transforming medical research.
Conclusion: The Transformative Potential of Real-World Data Analytics in Biomarker Discovery
Real-world data analytics has emerged as a game-changer in biomarker discovery. By harnessing the power of large-scale datasets and advanced analytics techniques, researchers can identify novel biomarkers, predict disease outcomes, and improve patient care. Real-world data provides a comprehensive and representative view of patient populations, capturing the complexities of real-world clinical practice. The applications and case studies showcased in this article demonstrate the immense potential of real-world data analytics in transforming medical research. As technology continues to evolve and datasets grow larger, real-world data analytics will undoubtedly play a pivotal role in accelerating biomarker discovery and advancing medical knowledge.