Machine Learning Metabolomics Approach Used to Diagnose Ovarian Cancer
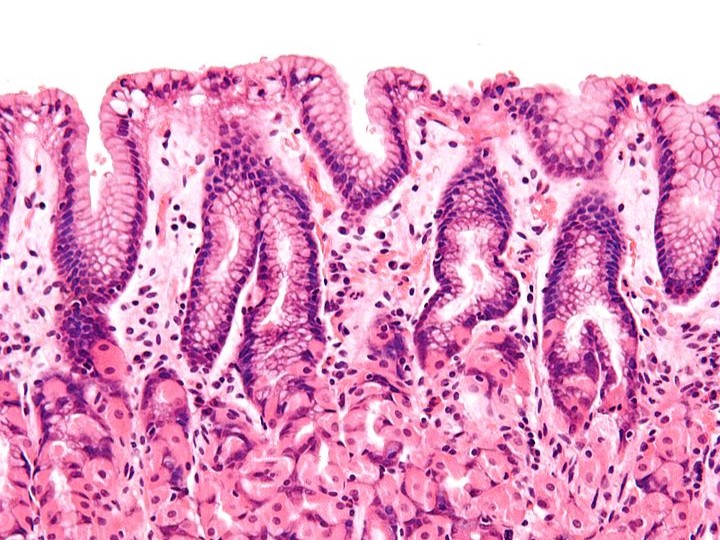
A study published in Gynecologic Oncology outlines how machine learning can be used to detect early stage ovarian cancer – often called ‘the silent killer’ – with 93% accuracy. This could represent a major turning point in the average survival rate of the disease.
The paper, “A personalized probabilistic approach to ovarian cancer diagnostics,” was published by researchers at Georgia Tech’s Integrated Cancer Research Center (ICRC). They believe that their computational method of diagnosis outperforms conventional testing methods – especially in early-stages.
Ovarian cancer is very difficult to detect in its early stages, as this is when it tends not to present any symptoms. This is particularly problematic as the five-year survival rate of late stage ovarian cancer is only 31%, as opposed to 90% when it is caught early. Therefore, there has been widespread effort to fulfil the unmet need represented by early detection methods of the disease.
The study enrolled 431 participants with ovarian cancer and 133 participants without the disease. A machine learning classification model then analysed profiles of metabolites obtained from patients’ serum samples. This returned a probability of the patient having ovarian cancer or not. Scientists were impressed by the accuracy of the model, which was reported to be 93%.
RELATED:
- Enhancing Patient Care: Exploring the Role of Digital Biomarkers in Patient-Centric Healthcare
- AI in Digital Pathology: The Applications of Explainable AI and Deep Learning Approaches in Clinical Practice
- A Wearable Ultrasound Patch for the Home Detection of Breast Cancer
Corresponding author on the study, John McDonald, said: “This personalized, probabilistic approach to cancer diagnostics is more clinically informative and accurate than traditional binary (yes/no) tests. It represents a promising new direction in the early detection of ovarian cancer, and perhaps other cancers as well.”
McDonald, an emeritus professor at Georgia Tech’s School of Biological Sciences, is the Founding Director of the ICRC, which strives to improve methods to predict, diagnose, and treat cancer.
The personalised nature of the technology is a key feature. Rather than repeating the same methodology for each participant in the study, machine learning models are able to learn from the broad samples they are presented with.
Jeffrey Skolnick, Georgia Tech Regents’ Professor and co-author of the paper, said that “The set of human metabolites is a collective measure of the health of cells. And by not arbitrarily choosing any subset in advance, one lets the artificial intelligence figure out which are the key players for a given individual.”
Another co-author, Dongjo Ban, worked as a Graduate Research Assistant throughout the study, he said that it was for this reason that “we chose metabolic profiles as the backbone of our analysis.”
Now, the researchers on the study are seeking to commercialise their efforts by spinning out into a company. They plan to ask for the FDA’s approval to begin trials for the diagnostic tool.
Featured image credit:
Copyright © 2011 Michael Bonert.
You are free to share and adapt this image as per the CC BY-SA 3.0.