Interview with Simon Gao, Clinical Imaging Scientist at Genentech
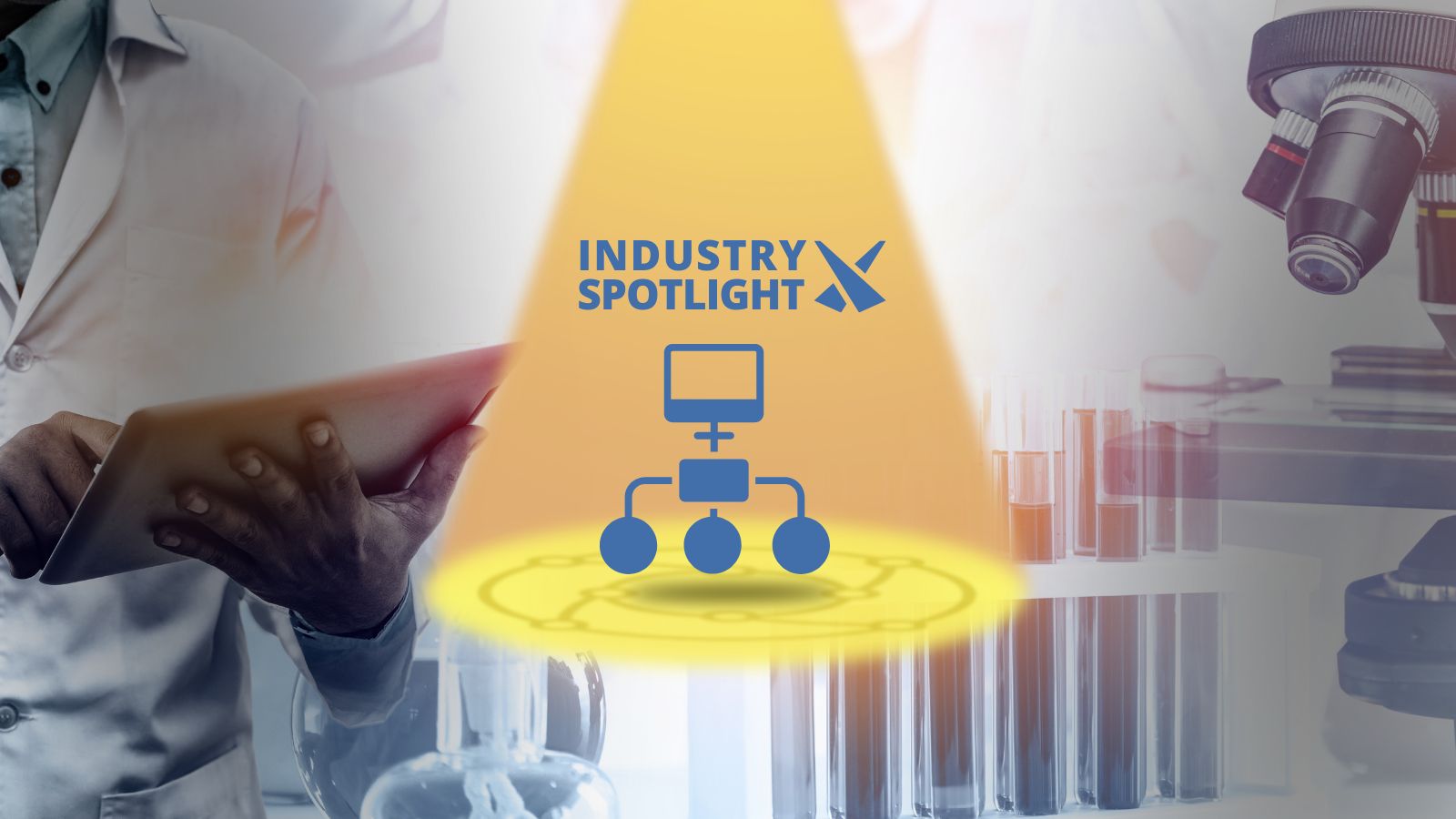
In this Insight Article, we caught up with Genentech's Simon Gao to discuss the current landscape of clinical imaging, biomarker discovery, and how AI-based approaches are assisting disease information capture.
Could you tell us a bit about your role within Genentech as a clinical imaging scientist?
I am a part of our Early Clinical Development (ECD) Clinical Imaging Group (CIG). ECD is responsible for taking Genentech’s molecules/therapies through Phase I and II clinical trials. Clinical Imaging Scientists (CIS) from CIG support our development teams with all aspects related to clinical imaging and image analysis. This ranges from designing the imaging strategy with the clinical and biomarker subteams, working with our operations and procurement teams on contracting an imaging vendor, aligning with imaging vendors on the imaging protocol and charter, to overseeing internal exploratory image analysis projects. The CIS within CIG are split by some combination of disease area and imaging modality. I mostly support our studies in Ophthalmology.
In your experience, what have been the biggest changes or overhauls to the clinical imaging and biomarker discovery landscape to date?
We have had two big focuses in Ophthalmology. One, we have tried to become much better about making our images findable, accessible, interoperable, and reusable (FAIR). A lot goes into making images FAIR, particularly in Ophthalmology where relevant metadata are not always captured, and it is common for imaging system manufacturers to have image file formats that are proprietary. Making images FAIR is basically the first step to be able to do anything with them, including taking advantage of state-of-the-art advanced analytics and AI-based methods.
- Patient response to immunotherapy could be calculated through AI-calculated biomarker
- Are neuroimmune proteins the key to neurodegenerative disease diagnosis?
- Collaborative approaches to next-gen sequencing and biomarker discovery through GOAL
And two, we have tried to be more quantitative. As an example, in the past, our imaging biomarker assessments were often in terms of presence or absence at the eye level. Now, we ask for more when dealing with critical metrics, whether that be counts, areas, or volumes.
Do AI-driven approaches to biomarker discovery have the potential to be a more significant change in terms of biomarker identification and data interpretation?
We have seen two clear use cases for AI-based approaches. Using AI-based segmentation models to enable detailed characterisation of disease as captured on imaging. It is generally too time consuming for expert readers/graders to draw, outline, or otherwise annotate every disease relevant feature in images. Building AI-based segmentation models on some initial batch of annotations (which could be generated via a crowd labelling approach[1]) and having the experts only review and correct errors can save a significant amount of time. The corrections can also be used to improve future iterations of the segmentation models.
Using image-based deep learning models to predict disease progression when disease progression can be quantified in the image. We have seen deep learning models[2] significantly outperform models built using the clinical data we collect in studies[3]. We have worked with our study statisticians to also show that these predictions, when used as covariates in the primary efficacy analysis, can improve power and thus the confidence in our trial results[3]. However, the challenge with a deep learning model is that it is essentially a black box, and interpretation of how the model gets to its prediction is not obvious but could provide new biomarker insights. We have been exploring approaches to interpretation[4], but there is still a lot more work to do in this space.
Is there an appetite within your area of the field for a higher level of automation, or is the implementation and integration of new workflows not feasible at present?
It depends on the specifics. Anything which may potentially impact patient safety rightfully gets a lot of scrutiny. Thus, for example, the lower hanging fruit could be improving automation as it relates to image management and flow. There is still quite a bit of opportunity there. As well, I mentioned image labelling previously. Some level of automation is necessary to make it even feasible in many cases.
Still the biggest piece is getting the proper buy-in and planning ahead. It can take a while to convince all the stakeholders of the value and align on a path forward.
Finally, do you have any thoughts on how future advances in AI and machine learning could influence clinical imaging and biomarker discovery?
It would be great to see more breakthroughs in the deep learning model interpretation front. Having a model which predicts more accurately than historical methods is nice, but if that model could teach us something new about the disease, biology, and so on, then that would really change how things are done.
Get your weekly dose of industry news and announcements here, or head over to our Biomarkers portal to catch up with the latest advances in cellular therapies.
References:
[1] Zhang, M. et al. (2022) “A Hierarchical Optical Coherence Tomography Annotation Workflow with Crowds and Medical Experts,” Investigative Ophthalmology & Visual Science June 2022, Vol.63, 3013 – F0283
[2] Anegondi, N. et al. (2023) “Deep Learning to Predict Geographic Atrophy Area and Growth Rate from Multimodal Imaging,” Ophthalmology Retina, 7(3), pp. 243–252.
[3] Steffen, V. et al. (2022) “Development and Validation of Prognostic Models to Increase Power of Clinical Trials for Geographic Atrophy (GA),” Investigative Ophthalmology & Visual Science June 2022, Vol.63, 1498.
[4] Cluceru, J. et al. (2022) “Feature Discovery using Ablation Studies for Deep Learning–Based Geographic Atrophy (GA) Progression Prediction,” Investigative Ophthalmology & Visual Science June 2022, Vol.63, 3859.