Disruptive Innovation in Diagnostics
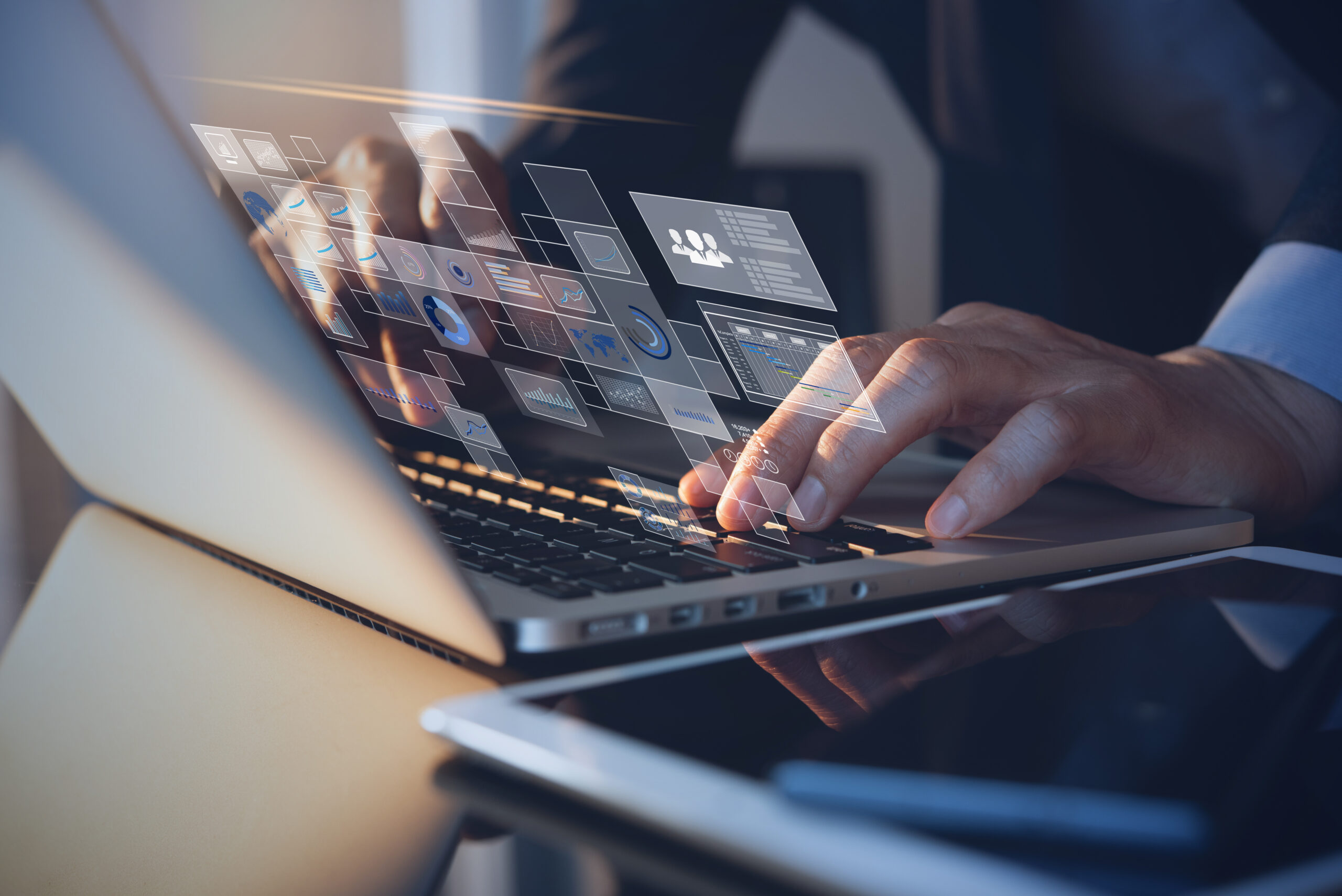
Disruptive diagnostics uses cutting-edge technology to make testing for diseases more effective, accurate, and efficient. Advances in this area will see the adoption of personalised medicine take centre stage in the pharmaceutical arena and will lessen the burden of healthcare costs.
Over the past few years, the increase in data digitisation has seen a rise in the prevalence of Artificial Intelligence (AI) and Machine Learning (ML). With big data sets comes big responsibility, and with that, a prevalent need for better data management tools.
In fact, the rapid advances in diagnostic technology have meant that the global molecular diagnostic market is projected to grow from 9.56 billion USD in 2020 to 16.12 billion USD by 2026, at a CAGR of 9.21%. Key players in the field include Qiagen, Roche, Molbio Diagnostics Pvt. Ltd., to name a few.
But what are the novel technologies impacting molecular diagnostics? And what does it take to bring a diagnostic test to the market?
Disruptive Innovation in AI and ML:
AI for disruptive innovation in diagnostic is a promising and well-established form of application. As Graham Ball, Professor of Bioinformatics at Nottingham Trent University, explains, “although there is currently a significant hype around AI and machine learning at the minute, some of these technologies have been around for quite some time.”
Despite its advantages, one of the predominant drawbacks to the large data sets produced by AI and ML is false discovery.
In fact, the first breakthrough of AI for the pharmacology industry came as early as 1950 with the advent of early Neural Networks. However, it was not until the latter half of the 20th-century that widespread use became prominent.
Despite its advantages, one of the predominant drawbacks to the large data sets produced by AI and ML is false discovery. “When you are looking at tens and thousands of variables, the chances of finding something that is not real, can be high,” Ball explains. Controlling false discovery during large-scale experiments is integral to ensuring the validity and reproducibility of statistical findings.
Data Cohorts – A Robust Solution to False Discovery:
Data Cohorts provide a cohesive resolution to large data analysis. Running deep learning algorithms in parallel can provide a dynamic means of cross-validation. As Ball puts it, “instead of lumping all the data together, parallel data cohorts break down the statistics into a more comprehendible format.” This form of behavioural analytics sorts the data into related groups based on shared characteristics which facilitates biomarker identification.
The John Van Geest Cancer Research Centre at Nottingham Trent University uses cohort analysis in breast cancer data sets to identify proliferation features to predict anthracycline response. The centre identified four data sets comprising around 4,500 cases and, in each group, posed multiple questions to establish a rank order of features and predictive molecules.
“Out of the top 100, we found 36 features and molecules that were predictive of those questions,” Ball explained. These 36 features looked at the probability of one single feature occurring randomly across all data sets. The final probability was a value of approximately 10 to –36. The John Van Geest Cancer Research Centre concluded that data cohort analysis provided a robust strategy to enrich proliferation across multiple data sets and a cross-validated means of biomarker development.
Standardisation for Biomarkers – The Implications of Data Regulation:
Once a new marker candidate is identified, it must then become standardised. According to Andreas Voss, Medical Director at Apis Assay Technologies, “biomarker standardisation can be a tricky and difficult business.” General Data Protection Regulation (GDPR) and In Vitro Diagnostic Regulation (IVDR) are the external factors that can both facilitate and become a bottleneck for disruption innovation.
The two regulatory initiatives heavily influence development strategies. Whilst IVDR protects and encourages technological and dynamic advances in pharmacology, ensuring sufficient standardisation often comes with GDPR scrutiny. As Benjamin Dizier, Head of In Vitro Diagnostics at UCB, puts it, “assays in development need to have a clearly defined clinical and predictive intent, where you identify what decision they will determine.”
- How can the Industry Transform Itself with Improved Biomarkers for Clinical Development?
- Discover Cutting-Edge Techniques to Streamline Clinical Testing in Precision Medicine
- Read our 2021 Biomarkers Market Report to Discover the Latest Industry Insights and Trends
The assay marker must have an outlined use of purpose, whether it is to determine a different course of treatment or to monitor patient response. “However, GDPR and IVDR affect this decision considerably, especially when it comes to disruptive innovation and more complex diagnostic,” Dizier continues. “In GDPR, there is an issue concerning how much data is generated using bioinformatics and how much data can be used to refine the signal which has been identified,” he elaborates.
A common problem is that developers amass a considerable amount of valuable data, which would greatly benefit the patients, but without the ability to make full use of it. “The bar is high when it comes to bringing a diagnostic test to the market, with extensive consultations required to meet quality and approval needs,” Dizier explained. As it stands, there are a significant number of regulatory steps, considerations, and restrictions that developers must adhere to. Therefore, current industry concerns look towards implementing a strategised approach to allow a free-flowing diagnostic process.
Future Perspectives in Innovative Diagnostics:
Greater planning is key to overcome and sufficiently address bioinformatics’ standardisation demands. In particular, Dizier suggests developers “account for and anticipate the amount of work it takes to become fully compliant with regulatory bodies, at the earliest possible stage.” This will ensure minimal delay and lessen the impact of data restriction on the final assay’s approval.
Other strategies for innovation include improving concordance between algorithms in data sets. By comparing two different algorithms and analysing results, the industry will be able to take actionable steps toward streamlining diagnostic procedures and regulations.
As Ball explains, ensemble panels “allow for quick identification of statistically compelling data and irrelevant background noise.”
Finally, building ensemble panels can offer a stable and objective means of analysis. As Ball explains, this approach “allows for quick identification of statistically compelling data and irrelevant background noise.” Ball even spoke of building the ensemble model with the algorithms in mind to ensure optimal functioning and data readout.
Despite its complexity, disruptive innovation in diagnostics is an ever-evolving area of industry development. The emergence of new biological insights from spatial omics, transcriptomics, and nanostring technologies, means the complexity and utility of biomarkers have dramatically expanded over the past couple of years. ML and AI capabilities have provided exciting opportunities for biomarker discovery through to companion diagnostic commercialisation. To optimise regulatory success and quality control, integrated and robust data management systems must be achieved.
Want to stay up to date with the latest Biomarker news? Register now for Oxford Global’s flagship event, Biomarkers US: In-Person. This is a must-attend forum covering the latest trends transforming biomarker and translational research.