Investigating Biases in Diagnostics and Biomarkers: Three Case Studies
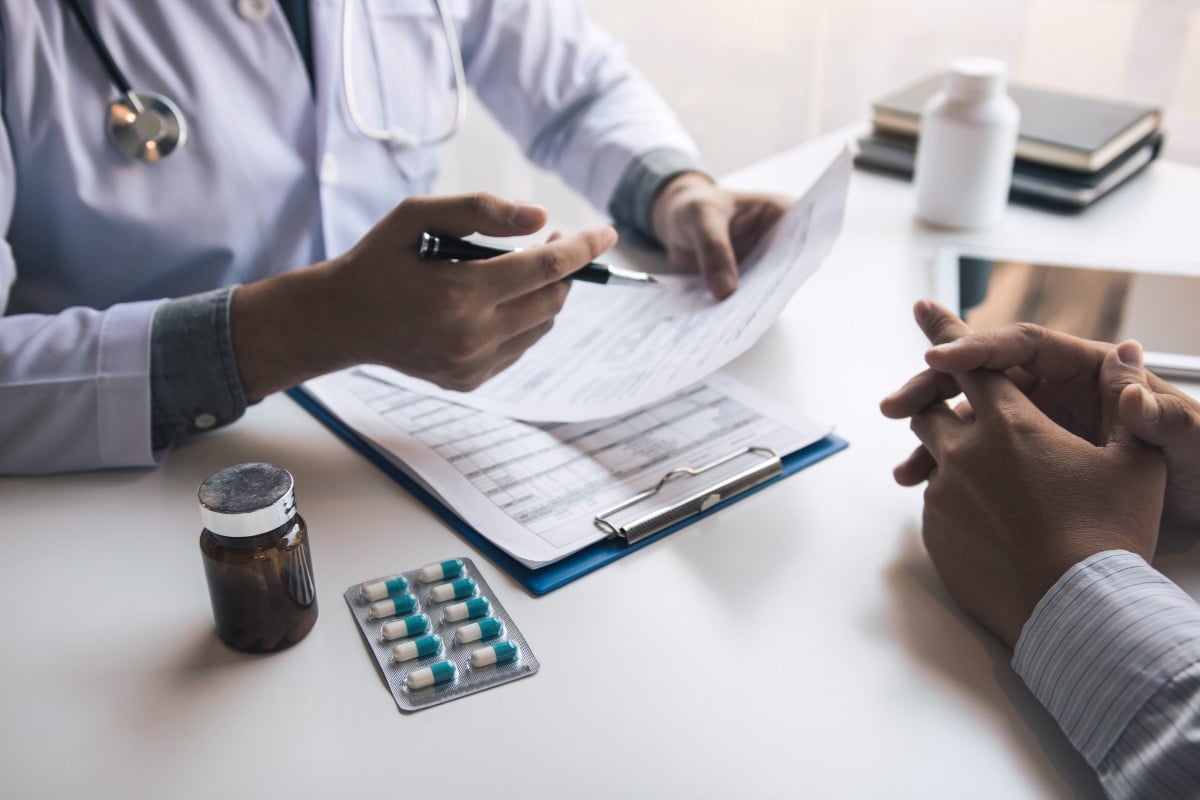
In the evolving landscape of medical diagnostics, there have been exciting advancements that hold promise for enhancing the accuracy and efficiency of diagnosis and treatment. However, recent studies underscore a critical caveat: the susceptibility of biomarkers and diagnostic tools to biases in their efficacy and application.
This article examines three case studies that illuminate the multifaceted nature of biases in diagnostics, urging for a nuanced understanding and proactive mitigation strategies in medical practice and research.
Caution About a Reliance on AI Diagnostic Tools
Diagnosticians now have more tools than ever at their disposal to make essential diagnoses which could improve patient outcomes. However, one concern with the advent of these technologies that is becoming increasingly relevant is the impact that assistance from artificial intelligence (AI) may have on biases.
RELATED:
One issue with machine learning algorithms is the fact that biases in the datasets that they are trained on will affect their output. Furthermore, due to the size and complexity of these models, it can often be difficult to identify these unhelpful proclivities without extensive data analysis.
A study published in JAMA in December 2023 showed that clinicians using AI to assist in diagnoses would be significantly affected if the model that they used presented biases.
In the study, clinicians used an AI model which was intentionally biased to suggest certain diagnoses. For example, introducing a disproportionate likelihood of pneumonia for patients over 80, and an overdiagnosis of heart failure in obese patients.
The researchers found that bias would factor into clinicians’ own diagnoses when they were helped by a biased AI. Clinicians that had no AI helper made the correct diagnosis 73% of the time, with support from an unbiassed AI, it was 75.9%. However, the group that had assistance from an AI with pre-programmed bias only made the correct diagnosis 61.7% of the time.
The results show not only that AI is not an infallible tool for diagnostics, but also that clinicians can be susceptible to undertaking an AI’s biases themselves. Therefore, a reliance on AI tools can have negative consequences when it comes to the diagnosis of patients.
"The paper just highlights how important it is to do our due diligence, in ensuring these models don't have any of these biases," senior author Michael Sjoding, Associate Professor of Internal Medicine at the University of Michigan, told Live Science.
Failing to Consider Patient Populations in Biomarkers
Another area in which bias can be expressed is in failing to consider differences and requirements of patient populations.
RELATED:
A recent example of this emerged in January 2024, where a study in the Journal of the National Comprehensive Cancer Network reported that a common chemotherapy efficacy biomarker may underestimate the value of chemo for some young Black women.
The Oncotype 21-gene breast recurrence score (RS) is a test ordered for ER-positive, HER2-negative breast cancer patients. The biomarker was developed to predict the benefit that a patient would likely gain from undergoing adjuvant chemotherapy.
RS is the most commonly ordered multigene breast cancer biomarker in the United States. Researchers at the University of Illinois Chicago (UIC) have been investigating the disparity in treatment outcomes for Black women with ER-positive breast cancer.
Analysing the data, they found that the RS may rank some young Black women as unlikely to benefit from chemotherapy, when in fact they may have. Their analysis further showed that lowering the RS cutoff point could prove beneficial for this patient population.
Kent Hoskins, Professor of Oncology at UIC, told Inside Precision Medicine that “the research shows that it may be inappropriate for doctors to use exact cutoffs and tests regardless of race or ethnicity because there are underlying differences in biology.”
Herein, certain biases in biomarkers and diagnostics may present themselves when taking a ‘one-size-fits-all’ approach. Therefore it is vital for research to accommodate for the broad spectrum of patient populations to ensure that no patient – or group of patients – is left behind.
Gender Bias: Social and Historical Factors
It should not be forgotten that it was not until 1875 that the ‘Enabling Act’ allowed women to practice medicine in the UK. The knock-on effect of this results in the majority of historical medical research being male-centric, trials conducted by men for men.
RELATED:
Therefore, having women excluded from centuries of medical research may have rendered some bias in diagnosis and medical trials. This could be manifest in a variety of fields and indications, from female reproductive health to the lack of understanding of the prevalence of autoimmune disease in women.
It is important to recognise that scientific research is not conducted outside of a social context, and operating within a systemic vacuum is bound to return the same biases that exist in the modern world. Furthermore, these biases are not purely historical. There is still a disparity in the funding that is allowed for research into women’s health versus men’s health.
Conclusion
As we navigate the complexities of modern medicine, it's imperative to confront biases entrenched within diagnostic practices and biomarker evaluations. The case studies explored here underscore the nuanced interplay of technological advancements, societal dynamics, and historical legacies shaping healthcare outcomes.
Looking ahead, addressing biases demands a multifaceted approach that encompasses rigorous scrutiny of AI algorithms, inclusive research methodologies, and heightened awareness of historical disparities.